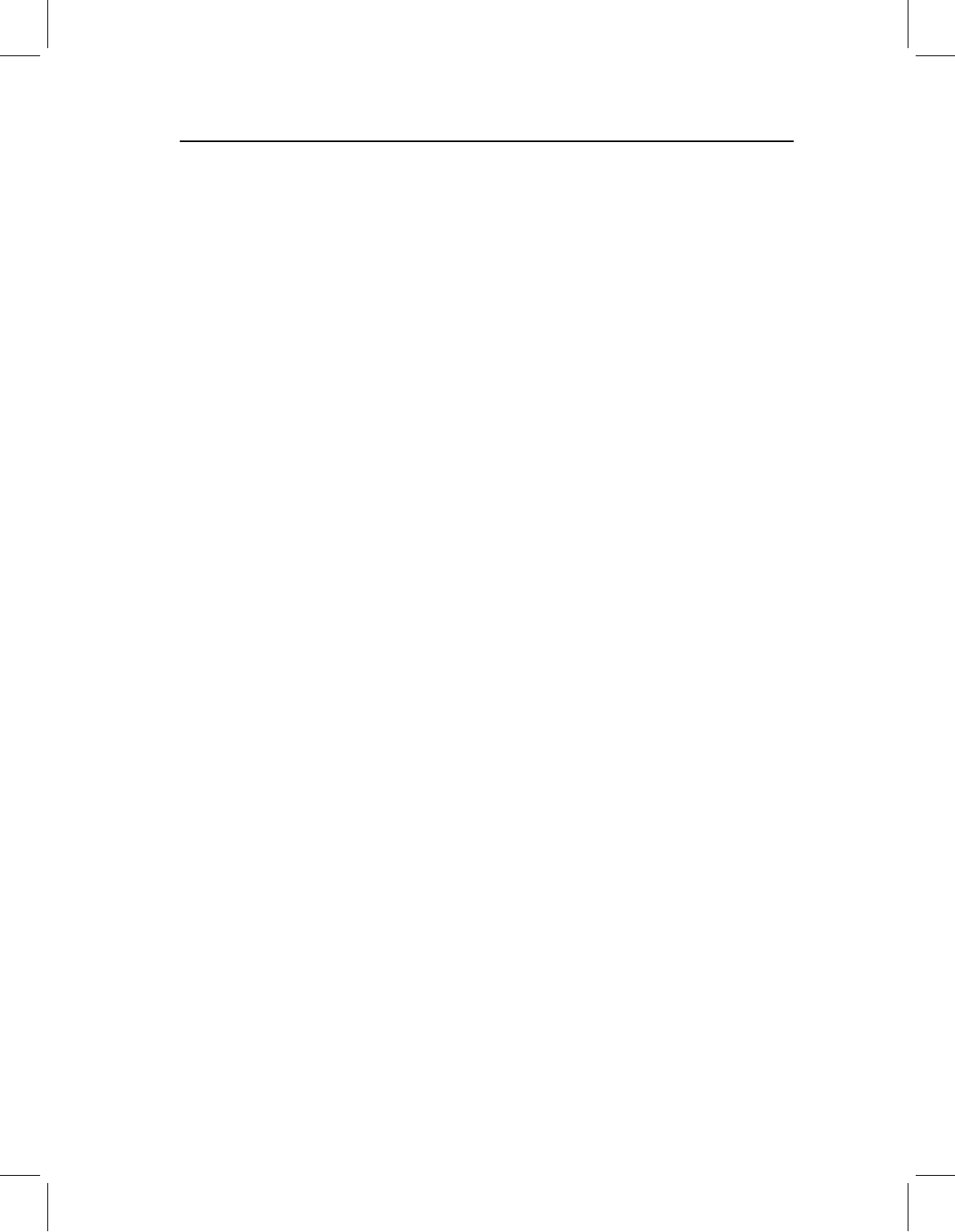
chap-10 4/6/2004 17: 26 page 230
230 GEOMETRIC MORPHOMETRICS FOR BIOLOGISTS
causation and correlation, which is often done by pointing to trends that are accidentally
related; but sometimes the trends are biologically related and yet there still is not a direct,
causal relationship.
To clarify the distinction between prediction and explanation, and also between math-
ematical and biological models, we can consider one common predictor of shape: size.
Often, much (or most) of the variation in shape is predicted by size. Based on the good fit
of our model to the data, we might conclude that size predicts shape, and so it might seem
that size explains shape. However, size is not a process. In the context of developmental
biology, we can explain size in terms of the proliferation of cells that add tissue to a struc-
ture. Because growth rates vary over the organism, cell proliferation (in conjunction with
cell death, cell differentiation, deposition of an extracellular matrix, etc.) produces changes
in shape. In this context, saying that size “explains” shape does not mean that size itself
causes shape; rather, it means that we are using “size” as shorthand for all those develop-
mental processes that jointly alter size and shape. Also, we are modeling this process by
a simple mathematical function, which is the model that is actually tested. In the context
of functional morphology, “size” is also shorthand, but it is shorthand for a more com-
plex argument. The underlying causal hypothesis is biomechanical; the idea is that shape
covaries with size because the mechanically optimal shape for one size differs from that for
another size. However, in correlating shape to size we are not demonstrating that selection
molds shape, nor even that shape affects performance; instead, we are demonstrating that
the relationship between size and shape is predicted by a particular mathematical model.
Most often, that mathematical model is the equation of a straight line, hence the term
“linear regression.” We are fitting the equation of a straight line to the data to find the
coefficients that best predict shape from values of the independent variable (e.g. size).
More specifically, we are trying to find the best estimates of the coefficients m and b of
the equation:
Y =mX +b +ε (10.1)
where Y is the dependent variable (shape in our case), m is the slope of the line, b is the
Y-intercept of the line, and ε is “error” (the variation in Y not explained by X). To predict
Y from X we need to find the values for m and b. Having obtained the best estimates for
them (using the approach described below), we can then ask whether they are statistically
different from zero.
The approach we use to find the values for those coefficients assumes a linear relationship
between X and Y. The reason for emphasizing this assumption is that a strong but non-
linear relationship might look like a weak linear one. Consequently, we end up rejecting
our biological model because the statistical analysis suggests a weak relationship between
variables, but the relation is actually strong but not linear. When the assumption of linearity
holds, our statistical analysis can tell us if Y is only weakly dependent on X – meaning that
knowledge about X does not enable us to predict Y. It is also possible that the relationship
of the two variables is statistically significant, but that m is such a small number that the
effect of X on Y is biologically trivial. It may be a statistically significant relationship, in
that it is stronger than expected by chance, but it might not be biologically significant.
Recognizing this distinction is important, because statistical significance is a matter of
sample size and the power of a test. With very large samples, or very powerful tests, we