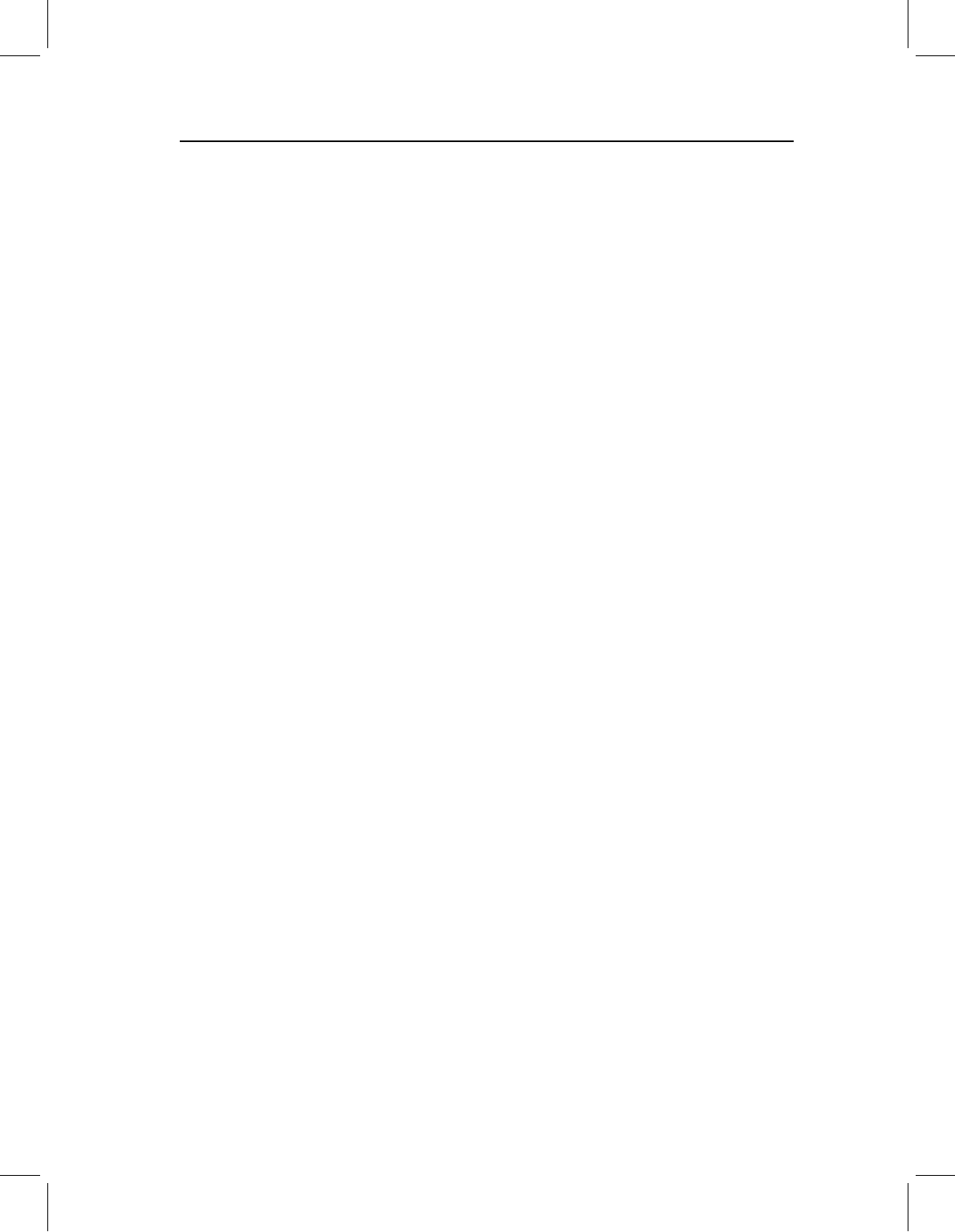
chap-11 4/6/2004 17: 27 page 264
264 GEOMETRIC MORPHOMETRICS FOR BIOLOGISTS
Unlike the situation for PCA, there is no analytic statistical test of the significance of
SAs, nor for the significance of the correlation between blocks. However, resampling-based
approaches can be applied to test these hypotheses. A permutation test, discussed by Rohlf
and Corti (2000), determines if the singular values are larger than could be produced by
a random permutation of associations among variables between blocks (keeping within-
block associations intact). We can ask whether the covariances between blocks exceed
those we would expect by chance. We can also ask if the correlation between singular axes
is significant using a permutation test – this determines whether the correlation between
the scores for each block exceeds what we would expect by chance. Both tests indicate
whether the observed patterns of covariance between blocks are statistically significant.
The similarity between PLS and PCA is important to understand because both impose
a similar constraint on the analysis: both define axes to be mutually orthogonal. SV2 is
defined to be orthogonal to SV1, just as PC2 is defined to be orthogonal to PC1. This
becomes important when biological factors are not orthogonal, which may be the general
rule. Even though the axes (both PCs and SAs) provide a useful, simplified space in which
to explore patterns in the data, the axes themselves need not correspond to any biological
factors. It is likely that PC1 and SA1 have a biological interpretation when they account
for a very large proportion of the variance or covariance, but the remaining axes are,
by definition, constrained to be orthogonal to them, making their interpretation more
dubious. This same issue arises when using PCA for explanatory or even comparative
purposes (see Rohlf and Corti, 2000, pp. 747–748; Houle et al., 2002). It is possible that
no useful (interpretable) axes will emerge from the PLS analysis, and that no significant
correlations between blocks will be found, particularly when the structure of the variation–
covariation within each block is especially complex.
Another important similarity between the methods, which should also inspire a cautious
approach to interpreting results, is that PLS extracts linear combinations of variables
(like PCA), but the relationship between blocks may be non-linear. In such cases, the
first dimension may represent the dominant linear trend, and others represent orthogonal
deviations from linearity. Thus, we would need to interpret SV1 together with SV2 to
understand the relationship between the two blocks, recognizing that a single non-linear
factor accounts for both. Of course, the issue of linearity is also important whether we are
analyzing the data by PCA/PLS, by regression, or by the method discussed in the following
section, CCA. However, most workers recognize that linearity is an important assumption
of regression; non-linearity might not seem so important in studies using PCA or PLS
because neither method is explicitly based on a linear model, so the impact of non-linear
relationships among variables might not seem to violate assumptions of the method.
PLS compared to CCA
Canonical correlation analysis, like multiple regression and PLS, examines the correlation
between blocks of variables. CCA closely resembles multiple regression, although, like
PLS, both blocks are treated symmetrically (there is no presumption that one block of
variables comprises causes and the other comprises effects). Nevertheless, the coefficients
produced by a CCA are interpreted like partial regression coefficients. This means that each
coefficient indicates the contribution made by an independent variable when the effects of