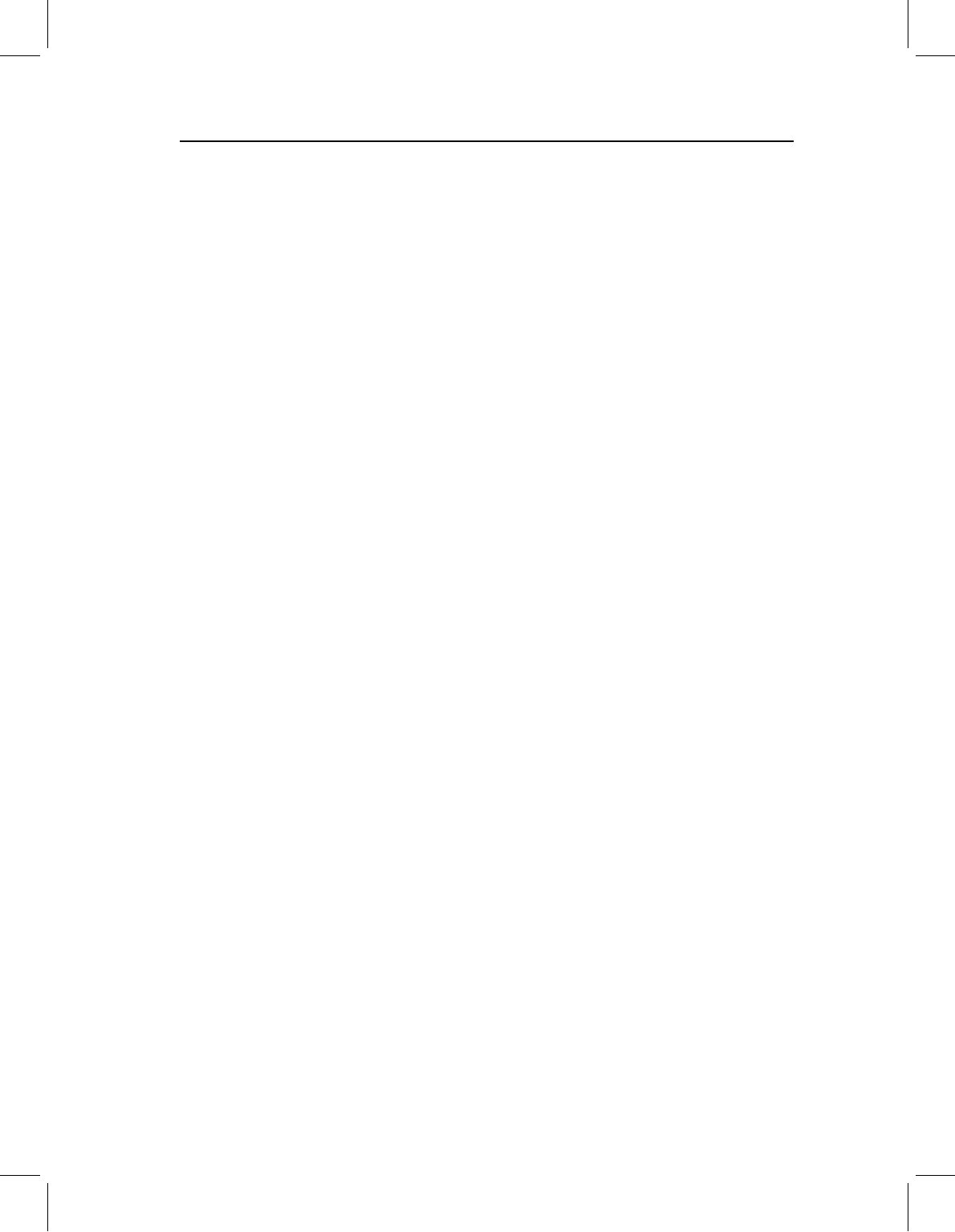
chap-08 4/6/2004 17: 25 page 202
202 GEOMETRIC MORPHOMETRICS FOR BIOLOGISTS
simulation than to determine analytically the distribution of an intricate statistical function,
particularly when the statistic is not a linear function. Because it is necessary to assume
a model of the distributions of the samples, the Monte Carlo method shares most of the
primary weaknesses of analytic statistics; if the observed distribution departs substantially
from the model, the Monte Carlo sets will not represent the actual system of interest. One
useful feature of the Monte Carlo method is the ability to determine the effect of different
distributional models (the ones typically used are the uniform, normal or Gaussian, and
Poisson) on the range of values estimated by the Monte Carlo sets. The comparison of
observed distributions to those produced by Monte Carlo methods is a powerful approach
to hypothesis testing.
For example, if we wish to determine the significance of the observed difference in the
means of sets X and Y:
X ={2, 2, 3, 4, 2, 5, 3, 2, 6, 2, 3, 4, 6, 2, 1, 4, 3, 7, 2, 3, 4, 4, 5, 8, 5, 2, 1, 3, 4, 4, 3} (8.35)
Y ={2, 2, 3, 2, 4, 2, 3, 2, 8, 9, 2, 9, 3, 2, 3, 3, 3, 9} (8.36)
we will test the null hypothesis that the two sets (X and Y) came from the same underlying
distribution, with the observed difference between them being due to a random assignment
of specimens into groups. To form the Monte Carlo set, we will assume that the single
underlying distribution is normal. We then estimate the mean and standard deviation of
this underlying distribution by merging the data sets into a single group. The mean of the
single distribution is 3.67 and the standard deviation is 2.1. To determine the significance
of the observed difference in the means of the two groups, we generate a series of paired
Monte Carlo sets, one with a sample size N
X
=31, one with a sample size N
Y
=18, and
we determine the difference between the two means. We then determine the proportion
of N
Monte Carlo
sets in which the difference between the means of the paired Monte Carlo
sets exceeds that observed between the means of the original data sets.
For the sets X and Y above, the Monte Carlo sets were generated under the assumption
that both samples were drawn from the same normal distribution, with a mean of 3.67 and
a standard deviation of 2.1 (the mean and standard deviation of the combined data sets).
In 480 of 1000 pairs of Monte Carlo sets (48%), the difference between the means of the
paired Monte Carlo sets exceeds the observed difference between the means of the original
data sets, thus the null hypothesis of a single underlying normal distribution cannot be
rejected. It should be noted that the combined data set (of all specimens in X and Y)is
probably not normally distributed, so we might want to repeat the Monte Carlo test using
other models of the underlying distribution.
Monte Carlo simulations are particularly useful for testing different hypothetical sit-
uations when the underlying distributions are believed to be well known. Monte Carlo
methods can be used in cases when bootstrap methods cannot, such as to estimate the
effect of increasing the sample size on the estimated variance; Monte Carlo simulations
are not limited by the observed sample sizes (as bootstrap methods are).
Example: computer-based tests and regression models
To this point, we have focused on t-tests, but computer-based methods are useful for a
wide variety of tests. To develop a more general understanding of these methods, we now