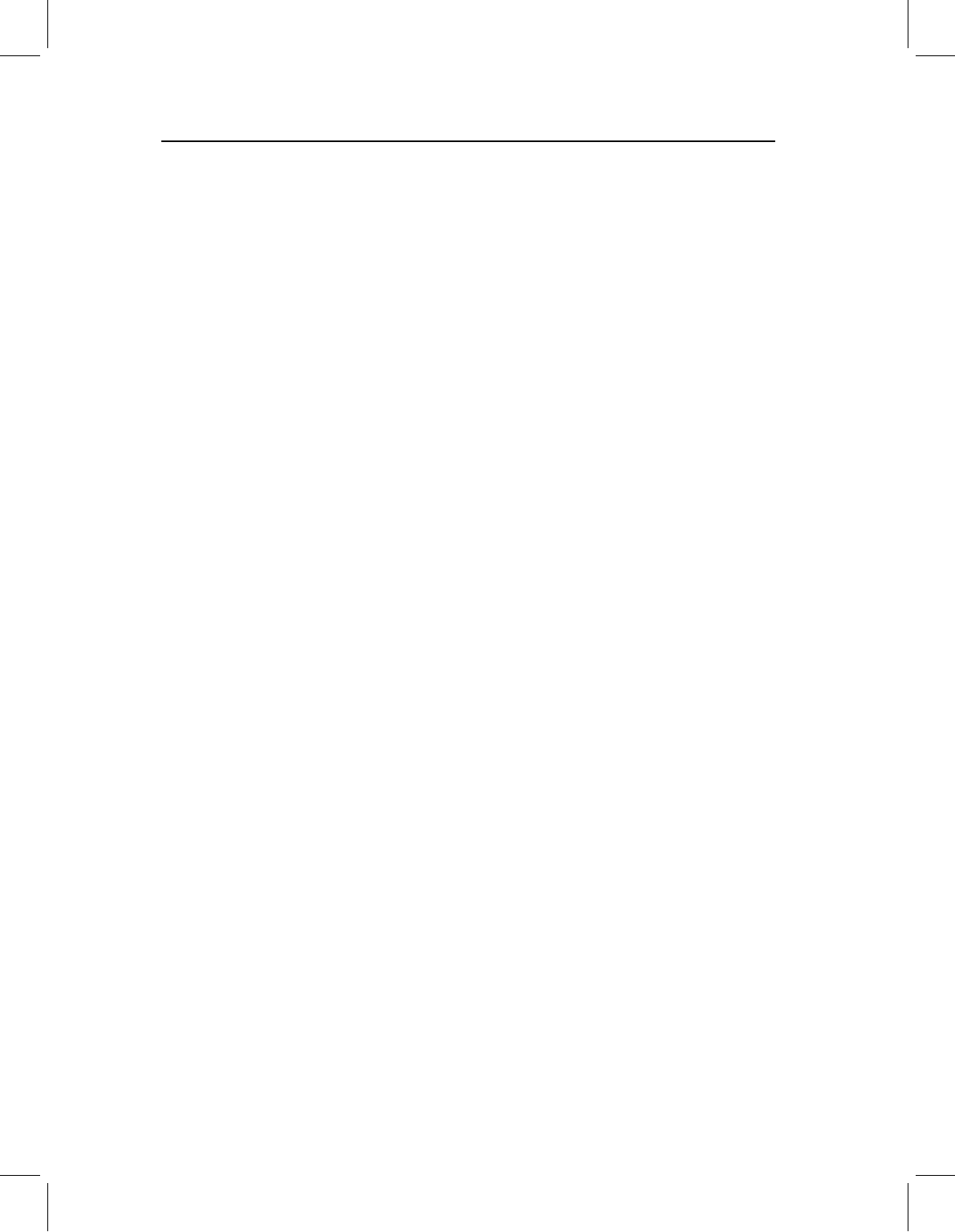
chap-07 4/6/2004 17: 24 page 171
ORDINATION METHODS 171
may complicate interpretations of CVs, it also adds to their utility. As a result of the
rescaling, CV1 is the direction in which groups are most effectively discriminated, which
is not necessarily the direction in which the group means are most different.
Groups and grouping variables
A group is a set of individuals that share a particular state of a discontinuous trait. Exam-
ples of groups include sexes, color morphs, species, and supraspecific categories like guilds.
To be analyzed by CVA the groups must be mutually exclusive, meaning that they cannot
comprise nested or intersecting sets. The groups differ by a categorical variable, which is
sometimes called a “qualitative trait” or a “grouping variable.” The important character-
istic of these variables is that they are not measured nor arrayed in a sequence; they do not
have intrinsic numerical values, and nor do they have an inherent order or sequence.
Sometimes, features that can be scored on a continuous graded scale are treated as cate-
gorical variables. For example, the proportions of meat and vegetation in an animal’s diet
can be quantified and scored along a continuum. Nevertheless, it is a common practice to
sort diets into a small number of categories (e.g. carnivore, herbivore, omnivore). Other
traits that might be treated in a similar fashion include geographic location and age. There
are several reasons for treating these kinds of traits as categorical variables. One is a lack
of sufficient information to justify or support a more finely graded analysis – for example,
a researcher may not have precise data on the proportions of food items in the diets of
all species or individuals in a study. Another reason for treating a quantifiable trait as a
categorical variable is that the investigator may not want to impose a hypothesis of order-
ing on the data, which is often a consideration when groups are not dispersed along a
single straight line. Similarly, the investigator may not want to assume that all steps are of
equal value (e.g. ontogenies often can be divided into discrete instars or age classes based on
sequences of developmental events, but the sequentially numbered steps may represent dif-
ferent amounts of time or ontogenetic change). Under these circumstances, a quantifiable
trait can be treated as a categorical variable and CVA would then be used to describe differ-
ences among the groups delineated by distinct states. However, the user should be aware
that taking this approach also limits the inferences that can be drawn from the result – for
example, an observation that age classes can be differentiated does not necessarily imply
the kind of monotonic progression from age to age that can be inferred from a regression.
Geometric description of CVA
To develop a geometric intuition for CVA, we return to the metaphor of a slightly flattened
watermelon. In PCA, we described the positions of seeds within the watermelon by finding
its greatest dimensions. In CVA, we are not interested in the positions of seeds in the
watermelon; instead, we want to describe the positions of the watermelons in the field
(centroids of the ellipses in Figure 7.10). If all we want to know is the location of each
melon, we could simply plot each melon’s centroids; however, suppose we want to find
the direction in which it is easiest to walk across the field without stepping on any of the
melons (perhaps we want to spread fertilizer in the field). To solve this problem, we want
to find the direction in which the melons are farthest apart. This requires that we know