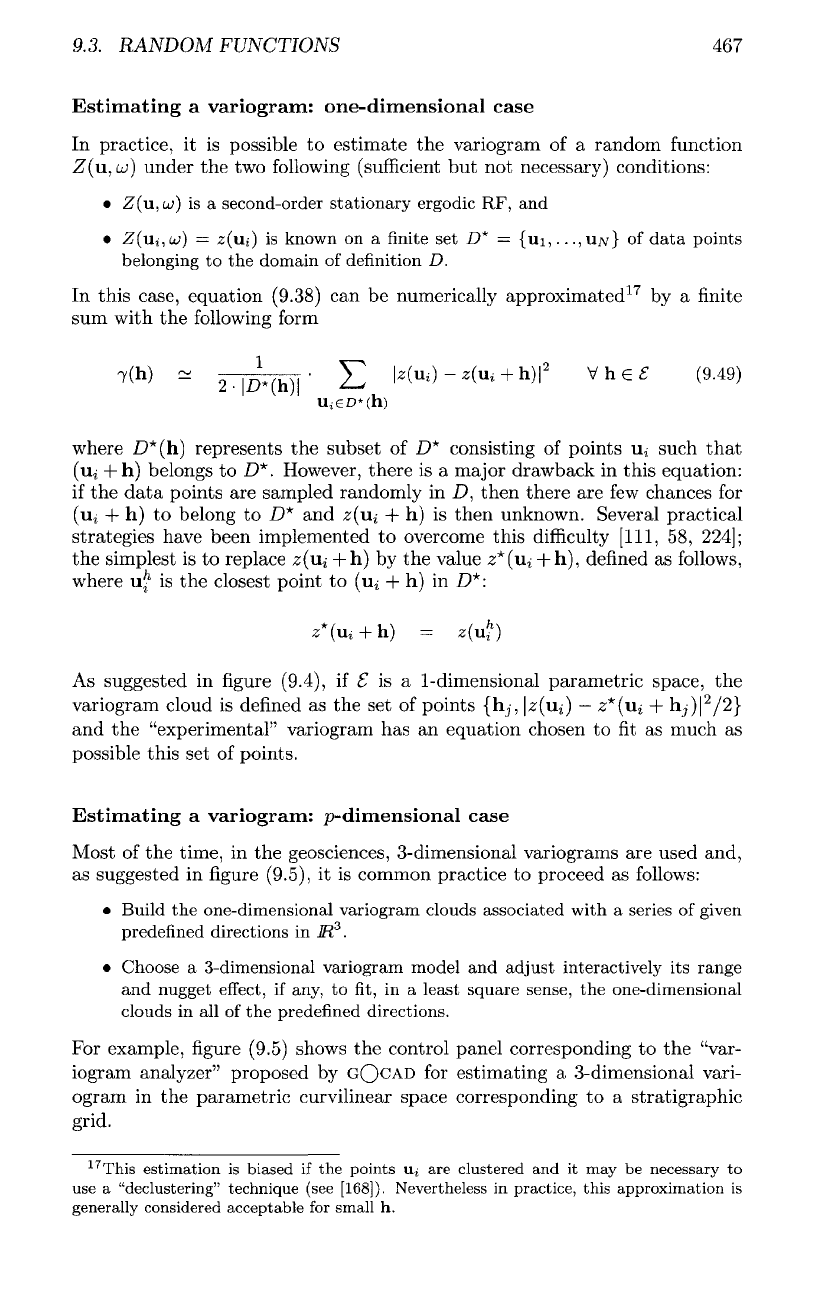
9.3.
RANDOM FUNCTIONS
Estimatin g
a
variogram:
one-dimensional
case
In
practice,
it is
possible
to
estimate
the
variogram
of a
random
function
Z(u,
(jj)
under
the two
following
(sufficient
but not
necessary) conditions:
•
Z(u,cj)
is a
second-order
stationary
ergodic
RF, and
•
Z(ui,uj)
=
z(ui)
is
known
on a finite set D* =
{ui,..
.,UAT}
of
data
points
belonging
to the
domain
of
definition
D.
In
this
case, equation (9.38)
can be
numerically
approximated
17
by a
finite
sum
with
the
following
form
where
D*(h)
represents
the
subset
of D*
consisting
of
points
u^
such
that
(ui
+h)
belongs
to
D*.
However,
there
is a
major drawback
in
this equation:
if
the
data
points
are
sampled randomly
in D,
then there
are few
chances
for
(ui
+ h) to
belong
to D* and
z(\ii
+ h) is
then unknown. Several practical
strategies
have been implemented
to
overcome this
difficulty
[111,
58,
224];
the
simplest
is to
replace
z(iij
+ h) by the
value
z*(\ii
+h),
defined
as
follows,
where
u^
1
is the
closest point
to
(u^
+ h) in
D*:
As
suggested
in figure
(9.4),
if 8 is a
1-dimensional
parametric space,
the
variogram cloud
is
defined
as the set of
points
{hj,
\z(ui]
—
z*(\ii
+
h-,)|
2
/2}
and the
"experimental" variogram
has an
equation chosen
to fit as
much
as
possible this
set of
points.
Estimating
a
variogram:
p-dimensional
case
Most
of the
time,
in the
geosciences,
3-dimensional
variograms
are
used and,
as
suggested
in figure
(9.5),
it is
common practice
to
proceed
as
follows:
•
Build
the
one-dimensional
variogram
clouds
associated
with
a
series
of
given
predefined
directions
in
]R
3
.
•
Choose
a
3-dimensional
variogram
model
and
adjust
interactively
its
range
and
nugget
effect,
if
any,
to fit, in a
least
square
sense,
the
one-dimensional
clouds
in all of the
predefined
directions.
For
example,
figure
(9.5) shows
the
control panel corresponding
to the
"var-
iogram analyzer" proposed
by
GQCAD
for
estimating
a
3-dimensional vari-
ogram
in the
parametric curvilinear space corresponding
to a
stratigraphic
grid.
17
This
estimation
is
biased
if the
points
Uj
are
clustered
and it may be
necessary
to
use
a
"declustering"
technique (see
[168]).
Nevertheless
in
practice, this approximation
is
generally
considered
acceptable
for
small
h.
467