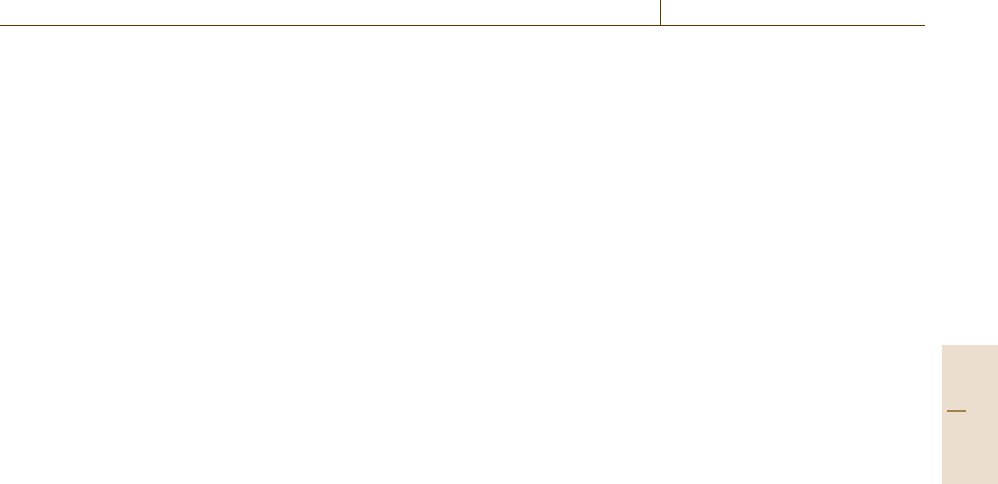
Quality in Measurement and Testing 3.3 Statistical Evaluation of Results 55
the box extends from the first to the third quartile (that
is, it contains the central 50% of the data points). The
median is marked as a dividing line or other marker in-
side the box. The whiskers traditionally extend to the
most distant data point within 1.5 times the interquartile
range of the ends of the box. For a normal distribution,
this would correspond to approximately the mean ±2.7
standard deviations. Since this is just beyond the 99%
confidence interval, more extreme points are likely to
be outliers, and are therefore generally shown as indi-
vidual points on the plot. Finally, a normal probability
plot shows the distribution of the data plotted against the
expected distribution assuming normality. In a normally
distributed data set, points fall close to the diagonal line.
Substantial deviations, particularly at either end of the
plot, indicate nonnormality.
The most common graphical method for two-
dimensional measurement data (such as measurand
level/instrument response pairs) is a scatter plot, in
which points are plotted on a two-dimensional space
with dimensions corresponding to the dimensions of the
data set. Scatter plots are most useful in reviewing data
for linear regression, and the topic will accordingly be
returned to below.
Planning of Experiments
Most measurements represent straightforward applica-
tion of a measuring device or method to a test item.
However, many experiments are intended to test for
the presence or absence of some specific treatment ef-
fect – such as the effect of changing a measurement
method or adjusting a manufacturing method. For ex-
ample, one might wish to assess whether a reduction in
preconditioning time had an effect on measurement re-
sults. In these cases, it is important that the experiment
measures the intended effect, and not some external nui-
sance effect. For example, measurement systems often
show significant changes from day to day or operator
to operator. To continue the preconditioning example,
if test items for short preconditioning were obtained by
one operator and for long preconditioning by a differ-
ent operator, operator effects might be misinterpreted
as a significant conditioning effect. Ensuring that nui-
sance parameters do not interfere with the result of an
experiment is one of the aims of good experimental
design.
A second, but often equally important aim is to min-
imize the cost of an experiment. For example, a naïve
experiment to investigate six possible effects might in-
vestigate each individually, using, say, three replicate
measurements at each level for each effect: a total of
36 measurements. Careful experimental designs which
vary all parameters simultaneously can, using the right
statistical methods, reduce this to 16 or even 8 measure-
ments and still achieve acceptable power.
Experimental design is a substantial topic, and
a range of reference texts and software are available.
Some of the basic principles of good design are, how-
ever, summarized below.
1. Arrange experiments for cancelation: the most pre-
cise and accurate measurements seek to cancel out
sources of bias. For example, null-point methods,
in which a reference and test item are compared
directly by adjusting an instrument to give a zero
reading, are very effective in removing bias due to
residual current flow in an instrument. Simultane-
ous measurement of test item and calibrant reduces
calibration differences; examples include the use of
internal standards in chemical measurement, and the
use of comparator instruments in gage block calibra-
tion. Difference and ratio experiments also tend to
reduce the effects of bias; it is therefore often better
to study differences or ratios of responses obtained
under identical conditions than to compare absolute
measurements.
2. Control if you can; randomize if you cannot: a good
experimenter will identify the main sources of bias
and control them. For example, if temperature is
an issue, temperature should be controlled as far as
possible. If direct control is impossible, the statisti-
cal analysis should include the nuisance parameter.
Blocking – systematic allocation of test items to
different strata – can also help reduce bias. For ex-
ample, in a 2 day experiment, ensuring that every
type of test item is measured an equal number of
times on each day will allow statistical analysis to
remove the between-day effect. Where an effect is
known but cannot be controlled, and also to guard
against unknown systematic effects, randomization
should be used. For example, measurements should
always be made in random order within blocks as far
as possible (although the order should be recorded
to allow trends to be identified), and test items
should be assigned randomly to treatments.
3. Plan for replication or obtaining independent un-
certainty estimates: without knowledge of the
precision available, and more generally of the un-
certainty, the experiment cannot be interpreted.
Statistical tests all rely on comparison of an effect
with some estimate of the uncertainty of the effect,
usually based on observed precision. Thus, exper-
Part A 3.3