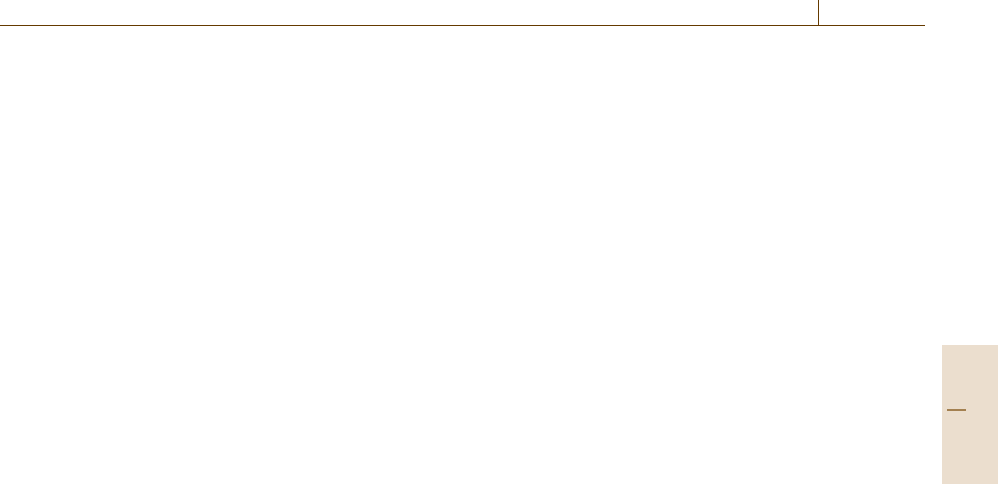
Quality in Measurement and Testing 3.1 Sampling 41
to assuring sampling quality is to assume that the cor-
rect application of a correct sampling protocol will give
a representative sample, by definition.
An alternative approach to assuring sampling qual-
ity is to estimate the quality of sampling empirically.
This is analogous to the approach that is routinely taken
to instrumental measurement, where as well as specify-
ing a protocol, there is an initial validation and ongoing
quality control to monitor the quality of the measure-
ments actually achieved. The key parameter of quality
for instrumental measurements is now widely recog-
nized to be the uncertainty of each measurement. This
concept will be discussed in detail later (Sect. 3.4), but
informally this uncertainty of measurement can be de-
fined as the range within which the true value lies,
for the quantity subject to measurement, with a stated
level of probability. If the quantity subject to measure-
ment (the measurand) is defined in terms of the batch
of material (the sampling target), rather than merely
in the sample delivered to the laboratory, then meas-
urement uncertainty includes that arising from primary
sampling. Given that sampling is the first step in the
measurement process, then the uncertainty of the meas-
urement will also arise in this first step, as well as in all
of the other steps, such as the sampling preparation and
the instrumental determination.
The key measure of sampling quality is therefore this
sampling uncertainty, which includes contributions not
just from the random errors often associated with sam-
pling variance [3.1] but also from any systematic errors
that have been introduced by sampling bias. Rather than
assuming the bias is zero when the protocol is correct,
it is more prudent to aim to include any bias in the es-
timate of sampling uncertainty. Such bias may often be
unsuspected, and arise from a marginally incorrect appli-
cation of a nominally correct protocol. This is equivalent
to abandoning the assumption that samples are represen-
tative, but replacing it with a measurement result that has
an associated estimate of uncertainty which includes er-
rors arising from the sampling process.
Selection of the most appropriate sampling proto-
col is still a crucial issue in this alternative approach.
It is possible, however, to select and monitor the ap-
propriateness of a sampling protocol, by knowing the
uncertainty of measurement that it generates. A judge-
ment can then be made on the fitness for purpose (FFP)
of the measurements, and hence the various components
of the measurement process including the sampling, by
comparing the uncertainty against the target value indi-
cated by the FFP criterion. Two such FFP criteria are
discussed below.
Two approaches have been proposed for the esti-
mation of uncertainty from sampling [3.2]. The first or
bottom-up approach requires the identification of all of
the individual components of the uncertainty, the sepa-
rate estimation of the contribution that each component
makes, and then summation across all of the compo-
nents [3.3]. Initial feasibility studies suggest that the use
of sampling theory to predict all of the components will
be impractical for all but a few sampling systems, where
the material is particulate in nature and the system con-
forms to a model in which the particle size/shape and
analyte concentration are simple, constant, and homo-
geneously distributed. One recent application success-
fully mixes theoretical and empirical estimation tech-
niques [3.4]. The second, more practical and pragmatic
approach is entirely empirical, and has been called top-
down estimation of uncertainty [3.5].
Four methods have been described for the empirical
estimation of uncertainty of measurement, including that
from primary sampling [3.6]. These methods can be ap-
plied to any sampling protocol for the sampling of any
medium for any quantity, if the general principles are
followed. The simplest of these methods (#1) is called
the duplicate method. At its simplest, a small proportion
of the measurements are made in duplicate. This is not
just a duplicate analysis (i. e., determination of the quan-
tity), made on one sample, but made on a fresh primary
sample, from the same sampling target as the original
sample, using a fresh interpretation of the same sampling
protocol (Fig. 3.1a). The ambiguities in the protocol, and
the heterogeneity of the material, are therefore reflected
in the difference between the duplicate measurements
(and samples). Only 10% (n ≥ 8) of the samples need
to be duplicated to give a sufficiently reliable estimate
of the overall uncertainty [3.7]. If the separate sources
of the uncertainty need to be quantified, then extra du-
plication can be inserted into the experimental design,
either in the determination of quantity (Fig. 3.1b) or in
other steps, such as the physical preparation of the sam-
ple (Fig. 3.1d). This duplication can either be on just one
sample duplicate (in an unbalanced design, Fig. 3.1b), or
on both of the samples duplicated (in a balanced design,
Fig. 3.1c).
The uncertainty of the measurement, and its compo-
nents if required, can be estimated using the statistical
technique called analysis of variance (ANOVA). The fre-
quency distribution of measurements, such as analyte
concentration, often deviate from the normal distribution
that is assumed by classical ANOVA. Because of this,
special procedures are required to accommodate outly-
ing values, such as robust ANOVA [3.8]. This method
Part A 3.1