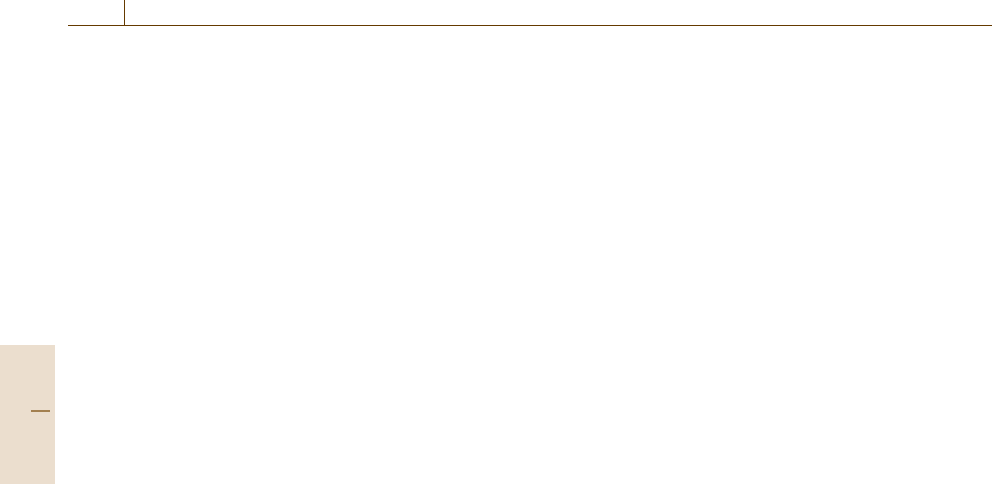
58 Part A Fundamentals of Metrology and Testing
or 5%. For stringent tests, 1% significance or less
may be appropriate. The term level of confidence
is an alternative expression of the same quantity;
for example, the 5% level of significance is equal
to the 95% level of confidence. Mathematically, the
significance level is the probability of incorrectly
rejecting the null hypothesis given a particular crit-
ical value for a test statistic (see below). Thus, one
chooses the critical value to provide a suitable sig-
nificance level.
5. Calculate the degrees of freedom for the test:the
distribution of error often depends not only on the
number of observations n, but on the number of de-
grees of freedom ν (Greek letter nu). ν is usually
equal to the number of observations minus the num-
ber of parameters estimated from the data: n −1
for a simple mean value, for example. For experi-
ments involving many parameters or many distinct
groups, the number of degrees of freedom may be
very different from the number of observations. The
number of degrees of freedom is usually calculated
automatically in software.
6. Obtain a critical value: critical values are obtained
from tables for the relevant distribution, or from
software. Statistical software usually calculates the
critical value automatically given the level of signif-
icance.
7. Compare the test statistic with the critical value or
examine the calculated probability (p-value). Tra-
ditionally, the test is completed by comparing the
calculated value of the test statistic with the critical
value determined from tables or software. Usually
(but not always) a calculated value higher than
the critical value denotes significance at the cho-
sen level of significance. In software, it is generally
more convenient to examine the calculated probabil-
ity of the observed test statistic, or p-value, which
is usually part of the output. The p-value is always
between 0 and 1; small values indicate a low prob-
ability of chance occurrence. Thus, if the p-value
is below the chosen level of significance, the result
of the test is significant and the null hypothesis is
rejected.
Significance Tests for Specific Circumstances. Table 3.2
provides a summary of the most common significance
tests used in measurement for normally distributed data.
The calculations for the relevant test statistics are in-
cluded, although most are calculated automatically by
software.
Interpretation of Significance Test Results. While
a significance test provides information on whether an
observed difference could arise by chance, it is impor-
tant to remember that statistical significance does not
necessarily equate to practical importance. Given suf-
ficient data, very small differences can be detected. It
does not follow that such small differences are impor-
tant. For example, given good precision, a measured
mean 2% away from a reference value may be statis-
tically significant. If the measurement requirement is to
determine a value within 10%, however, the 2% bias has
little practical importance.
The other chief limitation of significance testing is
that a lack of statistical significance cannot prove the
absence of an effect. It should be interpreted only as
an indication that the experiment failed to provide suf-
ficient evidence to conclude that there was an effect. At
best, statistical insignificance shows only that the effect
is not large compared with the experimental precision
available. Where many experiments fail to find a signif-
icant effect, of course, it becomes increasingly safe to
conclude that there is none.
Effect of Nonconstant Standard Deviation. Signifi-
cance tests on means assume that the standard deviation
is a good estimate of the population standard devia-
tion and that it is constant with μ. This assumption
breaks down, for example, if the standard deviation is
approximately proportional to μ, a common observation
in many fields of measurement (including analytical
chemistry and radiological counting, although the latter
would use intervals based on the Poisson distribution).
In conducting a significance test in such circumstances,
the test should be based on the best estimate of the
standard deviation at the hypothesized value of μ,and
not that at the value
¯
x. To take a specific example, in
calculating whether a measured value significantly ex-
ceeds a limit, the test should be based on the standard
deviation at the limit, not at the observed value.
Fortunately, this is only a problem when the stan-
dard deviation depends very strongly on μ in the range
of interest and where the standard deviation is large
compared with the mean to be tested. For s/
¯
x less than
about 0.1, for example, it is rarely important.
Confidence Intervals
Statistical Basis of Confidence Intervals. A confidence
interval is an interval within which a statistic (such as
a mean or a single observation) would be expected to be
observed with a specified probability.
Part A 3.3