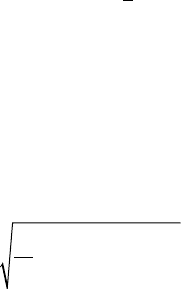
Self-Affine Fractals 117
value
Finally, one may also note here that analyses of covariance showed that
the 22 spectral exponents
were not all equal for each parameter, indicating potential differen-
tial spectral structures of the variables in question at the space-time scales of the whole sampling
experiment.
For frequencies smaller than the observed scale breakings, the inertia of the boat becomes neg-
ligible and the measurements are effectively taken following the ows, that is, in a Lagrangian
framework. One may note here that we had to average the original time series up to the Eulerian/
Lagrangian transition scale (Table 4.2) in order to be in the Lagrangian scales. In that way, our
characterization of the Lagrangian behavior of time series of temperature, salinity, light transmis-
sion, and in vivo uorescence is based on time series exhibiting at least 256 data points (that is, the
lower recommended bound for a data set to lead to reliable spectral analysis). In the following, we
then focused on 13 time series S1–S3, S5, S7, S9, S10–S12, S14, S19, and S21–S22. The previously
described transition is also conrmed by the similar scaling behaviors exhibited by temperature
and salinity time series (Table 4.3), which cannot be statistically distinguished from the theoreti-
cal slope
(Binomial test,
expected in the case of purely passive scalars advected by
Lagrangian uid motions (Monin and Yaglom 1975). On the contrary, in vivo uorescence and light
transmission spectral exponents show very specic behaviors (Table 4.3) that cannot be statistically
distinguished (Wilcoxon-Mann-Whitney U-test, p > 0.05). Moreover, analyses of covariance con-
cluded that the 13 spectral exponents
were not all equal ( p < 0.05) for both light transmission and
uorescence power spectra.
4.2.2 dE T r E n d E d Fl u c T u a T i o n an a l y s i s
4.2.2.1 theory
Detrended uctuation analysis (DFA) is an elegant tool to quantify simply and reliably the corre-
lations found in both stationary and nonstationary data (Peng et al. 1992, 1993, 1994). Compared
to more traditional analyses used to estimate the degree of correlation in temporal signals, such
as power spectrum analysis (see Section 4.2.1), Hurst analysis (Section 4.2.5), and autocorrela-
tion analysis (Section 4.2.7), the advantage of the DFA method is that it can accurately quantify
the correlation property of signals masked by polynomial trends (Hu et al. 2001; Chen et al.
2002). Here, a temporal signal (note that the method described hereafter is also applicable to
a spatial signal x(l)), is integrated by computing for each t the accumulated departure from the
mean of the whole series:
(4.29)
where N is the length of the data set. This integrated series is divided into nonoverlapping intervals
of length n. In each interval, a least-squares regression representing the trend in the interval is tted
to the data (Figure 4.12). The series X( t ) is then locally detrended by substracting the theoretical
values X
n
( t ) given by the regression. For a given interval length n, the characteristic size of uctua-
tions for this integrated and detrended series is calculated by:
Fn
N
Xt Xt
n
t
N
() [()()]=−
=
∑
1
2
1
(4.30)
This computation is repeated over all possible interval lengths. Note, however, that the width of
the regression windows ranged from six data points and to N/4 (Peng et al. 1994). The degree of
2782.indb 117 9/11/09 12:08:48 PM