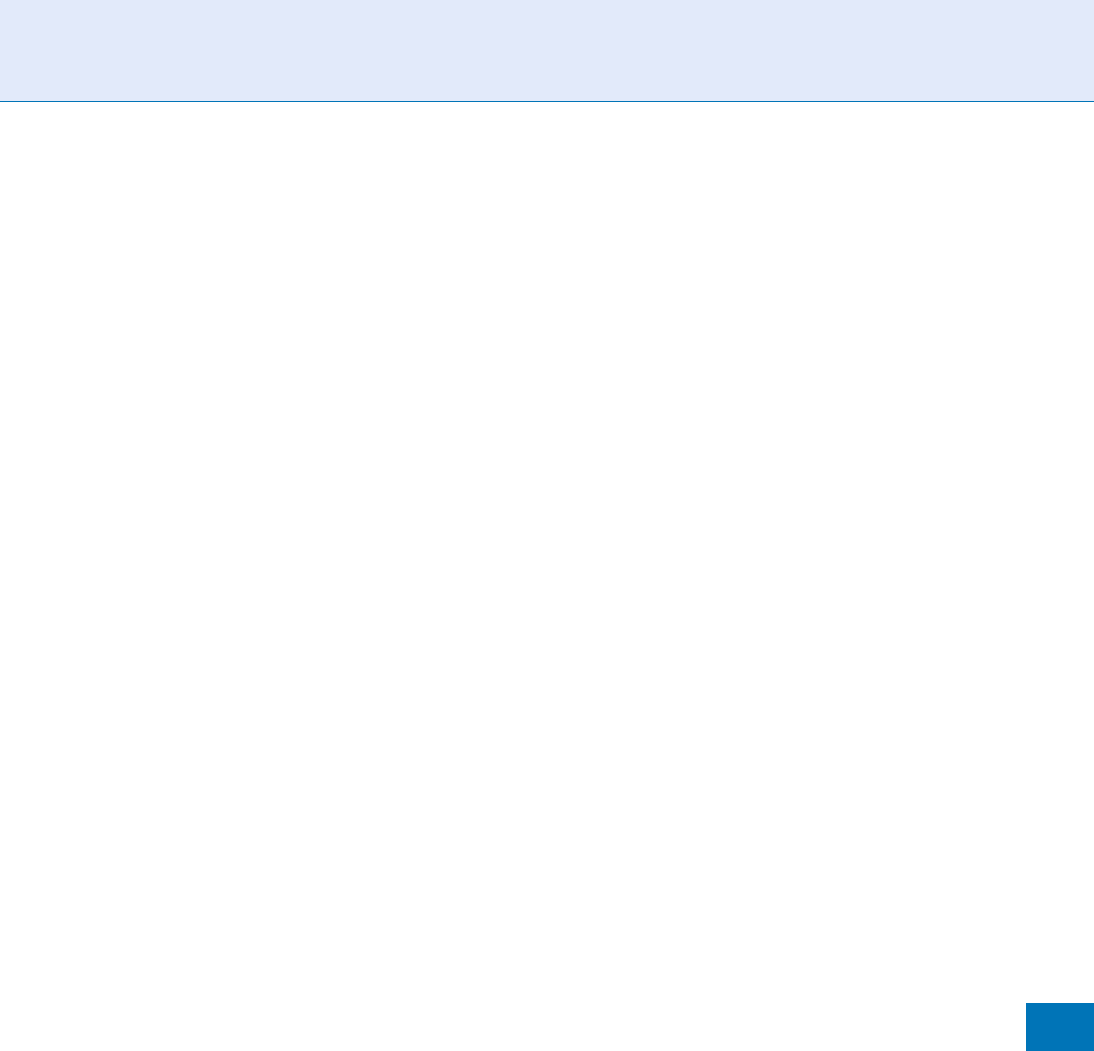
that involve multiple speakers. In this application, the
audio signal typically contains speech from different
speakers under different acoustic conditions. It is well
known that the performance of automatic speech recog-
nition can benefit greatly from speaker adaptation,
whether supervised or unsupervised. With the knowl-
edge of ‘‘who is speaking,’’ acoustic models for speech
recognition can be adapted to better match the environ-
mental conditions and the speakers. Furthermore, in the
speech-to-text conversion process, information about
speaker turns can also be used to avoid linguistic
discontinuity.
Also, capturing the speaker change in a given audio
stream could be very useful in military and forensic as
well as commercial applications. In forensic applica-
tions it is often required to process speech recorded by
means of microphones installed in a room where a
group of speakers conduct a conversation. Questions
such as how many speakers are present, at what time a
new person has joined (left) the conversation and
others are often asked. It is also often required to
determine the true identity of the speakers, or some
of them, using available templates of known suspects.
For this, one needs to segment the recorded signal into
the various speakers and then use conventional speaker
identification or verification methods.
Summary
There are a number of relevant applications that may
benefit from a speaker segmentation module. Among
them, ASR (rich transcription), video tracking, movie
analysis, etc. Defining and extracting meaningful char-
acteristics from an audio stream aim at obtaining a
more or less structured representation of the audio
document, thus facilitating content-based access or
search by similarity.
In par ticular, speaker detection, tracking, clustering
as well as speaker change detection are key issues in
order to provide metadata for multimedia documents
and are an essential preprocess stage of multimedia
document retrieval. Speaker characteristics, such as
the gender, the approximate age, the accent or the
identity, are also key indices for the indexing of
spoken documents. It is also important information
concerning, the presence or not of a given speaker in a
document, the speaker changes, the presence of speech
from multiple speakers, etc.
Related Entries
▶ Gaussian Mixture Models
▶ Hidden Markov Models
▶ Pattern Recognition
▶ Speech Analysis
▶ Speaker Features
▶ Session Effects on Speaker Modeling
▶ Speaker Recognition, Overview
References
1. Docio-Fernandez, L., Garcia-Mateo, C.: Speaker segmentation,
detection and tracking in multi-speaker long audio recordings.
In: Third COST 275 Workshop: Biometrics on the Internet,
pp. 97–100. Hatfield, UK (2005)
2. Chen, S.S., Gopalakrishnan, P.: Clustering via the bayesian in-
formation criterion with applications in speech recognition. In:
Proceedings of IEEE International Conference on Acoustics,
Speech and Signal Processing, vol. 2, pp. 645–648. Seattle, WA
(1998)
3. Delacourt, P., Wellekens, C.J.: DISTBIC: a speaker-based seg-
mentation for audio data indexing. Speech Commun. 32(1–2),
111–126 (2000)
4. Lu, L., Zhang, H.J.: Speaker change detection and tracking in
real-time news broadcasting analysis. In: ACM International
Conference on Multimedia, pp. 602–610. Quebec, QC, Canada
(2002)
5. Campbell, J.P.: Speaker recognition: a tutorial. Proc. IEEE 85(9),
1437–1462 (1997)
6. Gauvain, J.L., Lamel, L., Adda, G.: Partitioning and transcription
of broadcast news data. In: Proceedings of International Confer-
ence on Speech and Language Processing, vol. 4, pp. 1335–1338.
Sidney, Australia (1998)
7. Kemp, T., Schmidt, M., Westphal, M., Waibel, A.: Strategies for
automatic segmentation of audio data. In: Proceedings of the
IEEE International Conference on Acoustics, Speech and Signal
Processing, pp. 1423–1426. Istanbul, Turkey (2000)
8. Gauvain, J.L., Lamel, L., Adda, G.: The LIMSI broadcast news
transcription system. Speech Commun. 37(1–2), 89–108 (2002)
9. Moraru, D., Meignier, S., Fredouille, C., Besacier, L., Bonastre, J.F.:
The ELISA consortium approaches in broadcast news speaker
segmentation during the NIST 2003 rich transcription evaluation.
In: Proceedings of IEEE ICASSP’04, pp. 223–228. Montreal,
Canada (2004)
10. Lu, L., Li, S.Z., Zhang, H.J.: Content-based audio segmentation
using support vector machines. ACM Multimedia Syst. J. 8(6),
482–492 (2001)
11. Kim, H.G., Ertelt, D., Sikora, T.: Hybrid speaker-based segmen-
tation system using model-level clustering. In: Proceedings of the
IEEE International Conference on Acoustics, Speech and Signal
Processing, vol. 1, pp. 745–748. Philadelphia, PA (2005)
12. Vescovi, M., Cettolo, M., Rizzi, R.: A DP algoritm for speaker
change detection. In: Proceedings of Eurospeech03. (2003)
Speaker Segmentation
S
1283
S