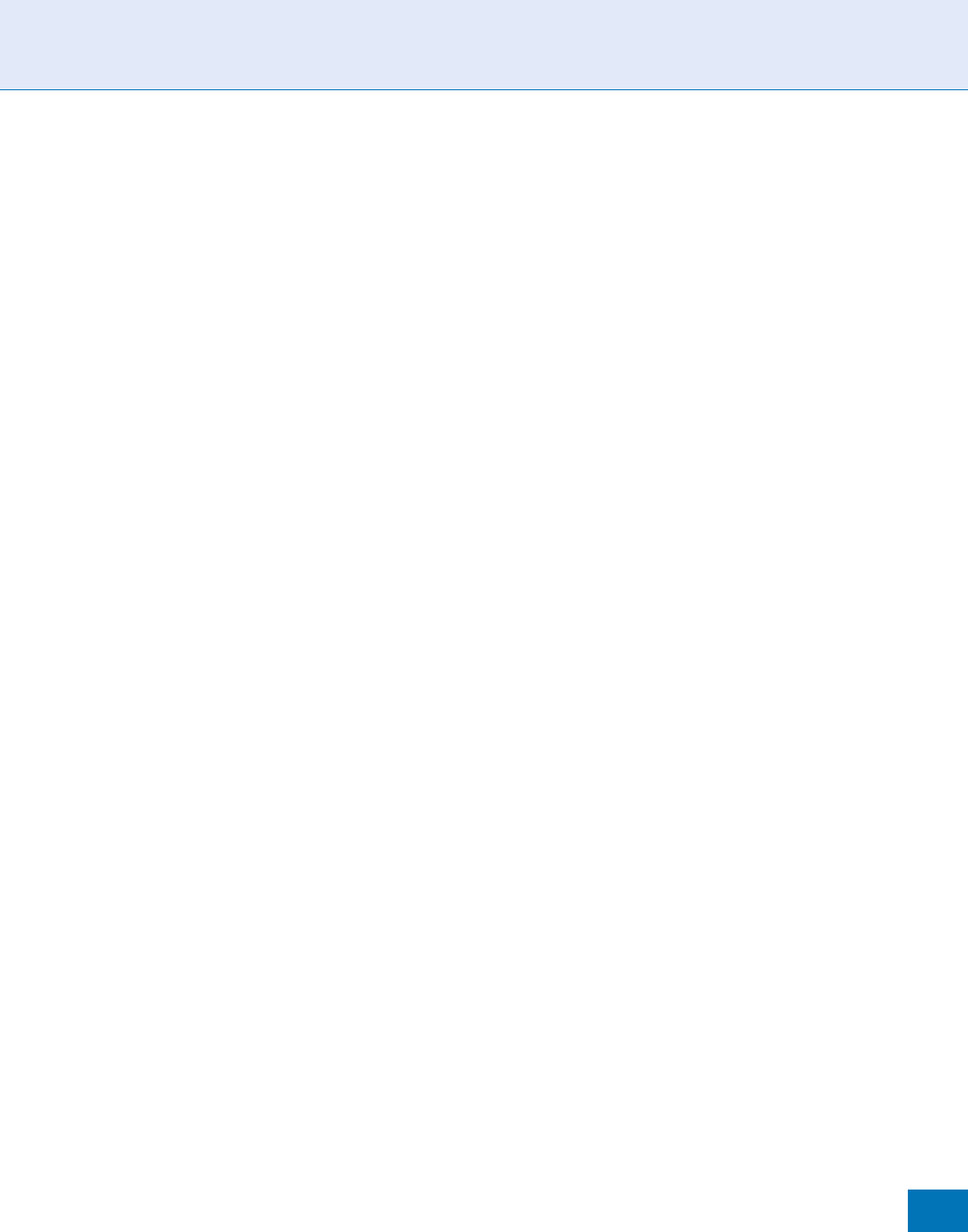
timed (p. 643). [3] used signatures that ‘‘on casual
visual inspection would pass as authentic’’ (p. 201).
[20] provides three characteristics of forgery: the
random forgery defined as one that belongs to a differ-
ent writer of the signature model; simple forgery
represented by a similar shape consistency with the
genuine signers shape; and the skilled forgery (p. 2).
[8] described two classes, the skilled forgery and
the random or zero effort forgery. For the skilled
forger, it is ‘‘signed by a person who has access to a
genuine signature for practice’’. In addition, it is
important to understand the handedness of the
genuine signer, and sometimes, the gender. Again,
the literature sometimes provides insights into the
impostor, and to some extent, the genuine data-
sets, all to varying degrees. For example, Lee, Berger,
Aviczer [19] collected 5,603 genuine signatures from
105 people, including 22 women and 5 individuals
who were left handed.
Therefore, when assessing the impostor datasets for
dynamic signature verification, there are probably
many different levels from ‘‘zero-effort’’ at the lowest
level through to trained and skilled forger. This all
depends on the level of information given to the im-
postor. For example, level 0 would be the ‘‘zero-effort’’
where ‘‘B’’ has no relevant knowledge of ‘‘A’’. The
knowledge is zero; there is no knowledge of name:
verbal, printed, or signed. The next level is that ‘‘B’’
knows ‘‘A’’’s name – this information is revealed ver-
bally. Therefore, the forger might mistake ‘‘Stephen’’
for ‘‘Steven’’ or ‘‘Steve,’’ depending on the formality or
ceremony of the signature. Level 2 would be that ‘‘B’’
has seen a static image of ‘‘A’’’s signature prior to the
impostor attempt. Level 3 is that ‘‘B’’ can see a static
image of ‘‘A’’’s signature at the time of signing. Level 4
is that ‘‘B’’ is a ble to trace a sample of ‘‘A’’’s signature.
Level 5 is where ‘‘B’’ has recently witnessed a sample of
‘‘A’’’s signing, and level 6 is where ‘‘B’’ has repeatedly
witnessed ‘‘A’’ signing. Even this methodology takes
into consideration neither the Perceived Signature
Strength (PSS) of the original signature nor the moti-
vation of the impostor. The PSS is a concept that
indicates that an ‘‘opportunistic forger’’ will not forge
a signature that is ‘‘hard’’ to forge, as their success at
the point-of-sale may be not as high as an ‘‘easy’’
signature. This is more of an ‘‘opportunistic’’ forgery
as opposed to a more sophisticated attack on the
signature as outlined in previous research. For the
case of this study, an opportunistic forger is analogous
to an ‘‘opportunistic thief ’’ – working on their own
without any equipment [16].
References
1. Mansfield, A and National Physical Laboratory (Great Britain):
Best practices in testing and reporting performance of biometric
devices (2002)
2. Hamilton, D., et al.: Low cost dynamic signature verification
system. European Convention on Security and Detection,
pp. 202–206 (1995)
3. Nelson, W., Kishon, E., Use of dynamic features for signature
verification. Decision Aiding for Complex Systems, Conference
Proceedings, 1991 IEEE International Conference on Systems,
Man and Cybernetics, vol.1, pp. 201–205 (1991)
4. Vielhauer, C., Steinmetz, R., Mayerhofer, A.: Biometric hash
based on statistical features of online signatures. Proceedings
of the 16th International Conference on Pattern Recognition,
Quebec City, Canda, vol. 1, pp. 123–126 (2002)
5. Leclerc, F., Automatic signature verification: the state of the
Art–1989–1993. Int. J. Pattern Recog. Artif. Intell. 8, 643–660
6. Elliott S., Hunt, A.: Dynamic Signature Forgery Assessment and
Signature Strength Perception, Aeros. Electron. Syst. Mag. 23,
13–18 (2008)
7. Fairhust, M., Ng, S.: Management of access through biometric
control: A case study based on automatic signature verification,
Universal Access in the Information Society, vol. 1, June 2001,
pp. 31–39
8. Kholmatov, A., Yanikoglu, B.: Biometric authentication using
online signatures. Computer and Information Sciences (ISCIS)
2004, pp. 373–380; http://www.springerlink.com/content/
a7q4keh1dv8d1u5f
9. Nalwa, V.: Automatic on-line signature verification, Computer
Vision – ACCV’98, 1997, pp. 10–15; http://dx.doi.org/10.1007/
3–540–63930–6_98
10. Ohishi, T.: et al.: Pen-input on-line signature verification
with position, pressure, inclination trajectories, Parallel and
Distributed Processing Symposium, Proceedings 15th Interna-
tional, 2001, pp. 1757–1763
11. Elliott, S., Hunt, A.: An assessement of dynamic signature forg-
ery and perception of signature strength. Procedding of the 40th
Annual IEEE International Carnahan Conference on Security
Technolgy, pp. 186–190 (2006)
12. Komiya Y., Matsumoto, T.: On-line pen input signature verifica-
tion PPI (pen-Position/pen-Pressure/pen-Inclination), IEEE
SMC ‘99 Conference Proceedings. IEEE International Confer-
ence on Systems, Man, and Cyberbetics, vol. 4. pp. 41–46 (1999)
13. Schmidt, C., Kraiss, K.: Establishment of personalized templates
for automatic signature verification. Proceedings of the Fourth
International Conference on Document Analysis and Recogni-
tion, vol. 1, pp. 263–267 (1997)
14. Lee, W.-S., Mohankrishnan, N., Paulik, M.: Improved Segmen-
tation through dyanmic time warping for signature verification
Zero Effort Forgery
Z
1413
Z