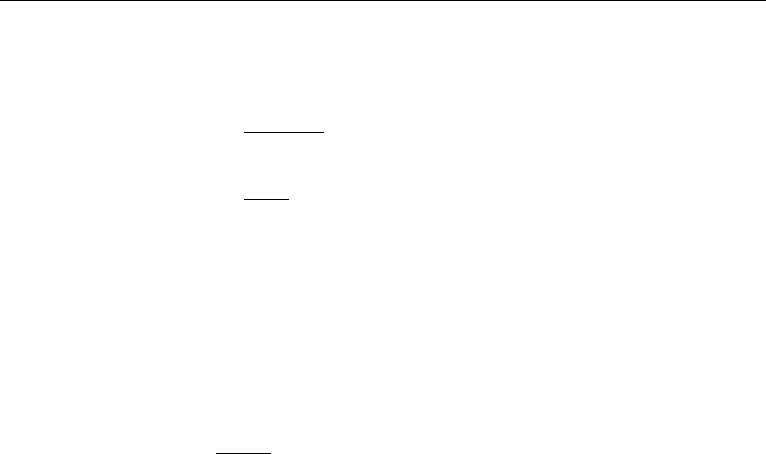
2. The next question is: What is the probability that one out of these 10 accidents will
lead to a spill greater than 100,000 tons? This can be seen as a binomial situation:
pðxÞ¼
n!
x!ðn xÞ!
p
x
ð1 pÞ
nx
pð1Þ¼
10!
1! 9!
0:00626
1
ð1 0:00626Þ
9
¼ 0:059
The risk of the catastrophic scenario on an annual basis is 6%. In other words this
is a situation that is fairly probable or at least far from being improbable! This
conclusion may therefore lead to an improvement in the operation.
3. The last point was not quite correct as there is a remote probability that even more
than one accident may lead to a spill of at least 100,000 tons. The probability that all
10 accidents give a catastrophic spill can be written:
pð10Þ¼1
10!
0! 10!
0:0026
0
ð1 0:0026Þ
10
¼ 1 0:9387 ¼ 0:061
The result is almost identical for the simple reason that having more than one
catastrophic spill is a very remote outcome.
Given the probability of 0.06 for this disaster scenario, we may estimate the
return period:
T
R
¼ 1=ð0:06Þ¼16:7 years
It can be concluded that this estimate gives a much lower return period than the first one
(26 years).
4.5.6 Extreme V alue Estimation
In many situations the risk manager is, as already pointed out, more concerned with the
worst-case situation rather than the average loss number. It may then be more feasible to
focus on the extreme values in each observation period rather than using the whole set
of data.
Table 4.8 reports the most serious single accident measured by the number of fatalities
for the offshore sector in the years 1973–80. It can be observed that the variation is quite
large and is best illu strated by the last two years where the number went from 1 fatality to
123 fatalities as a result of the Alexander L. Kielland loss.
It is possible to estimate a so-called extreme value distribution on the ba sis of such a
sample set. The approach is basically the same as described in the preceding section with a
few modifications.
114 CH APTER 4 STATISTICAL RISK MO N IT O RI N G