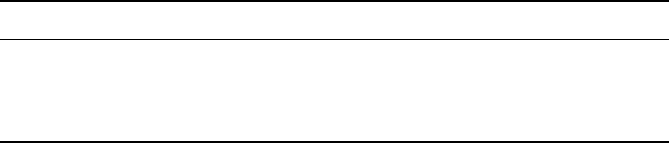
that removal of the trend has lost more of the variance than REML has done.
The REML variogram of the residuals from the quadratic trend, Figure 9.2(c),
has a larger sill variance as has the REML variogram computed on the residuals
from the relation between sand and EC
a
, Figure 9.2(d).
We mentioned above that there is a dearth of software in the public domain
for spatial prediction by REML. Readers, having seen Figure 9.2(a) in which
the experimental sequence of semivariances follows what looks like a
spherical form to about 300 m, and having also seen in Chapter 8 that only
data close to a target point carry significant weight, might wonder whether
in this case they could use ordinary kriging with the raw data. Let us see
what happens if we take this approach. A spherical function, the solid line in
Figure 9.2(a), fits the experimental variogram well to 300 m. Table 9.2 gives its
parameters. We used this model with the raw data to estimate values at the
nodes of the 5 m 5 m grid by ordinary punctual kriging. Figure 9.3(a) shows
the resulting map, which looks little different from those made with REML. The
map of the kriging variances, however, shows that the kriging errors are
greater.
We can summarize the four outcomes of the procedures. All four estimators
are unbiased, and because kriging is so robust the estimates themselves are
similar. They differ substantially in their variances, which are summarized in
Table 9.3. Ordinary kriging is the least precise, with a median variance of
53.1.
Universal kriging by REML reduces the variance to a median of 41.6. Kriging
with external drift has a very similar median variance, 41.3. In both, making
use of the additional information, either in the trend or in the subsidiary
correlated variable, EC
a
, improves the precision of the predictions. Note,
however, that the kriging variances from the KED have a smaller standard
deviation; they are less variable than those from universal kriging, and from the
other two techniques. The median kriging variance of the OLS residuals of 41.6
is remarkably similar to those of the REML predictions in this instance. Note,
however, that the OLS kriging variances underestimate the true kriging
variances of that method because the errors arising from the OLS fitting of
the trend are not taken into account. The fact that they are so similar to the
REML variances is fortuitous.
Table 9.3 Summary statistics of variances for four forms of kriging.
Kriging Mean Median Std dev.
Raw data 63.2 53.1 21.0
OLS residuals 52.0 41.6 21.7
REML, universal 53.5 41.6 26.3
REML, external drift 48.2 41.3 14.6
Case Study 211