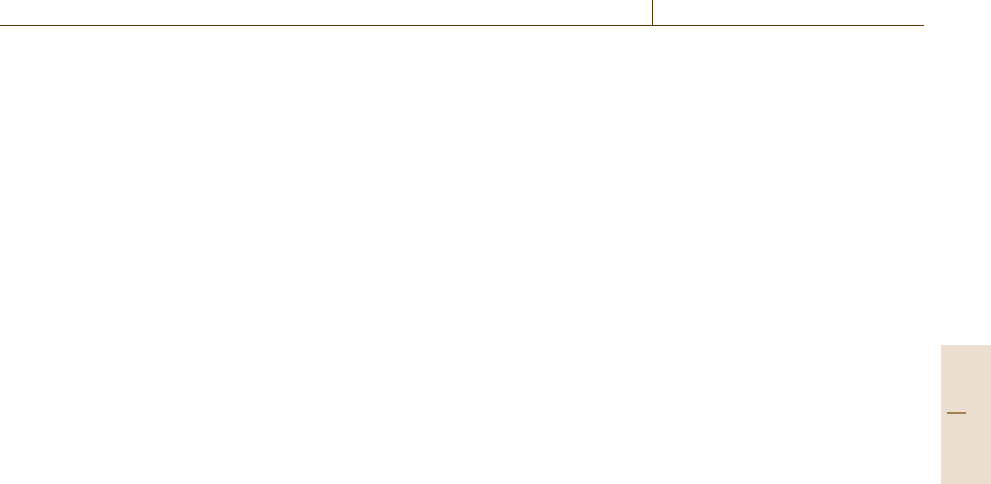
Automation in Hospitals and Healthcare 77.2 The Role of Medical Informatics 1385
ical ontologies are needed to explicitly articulate the
relationships.
The second example is rule writing for decision
support or reporting. While electronic medical records
systems have allowed institutions to write rules for de-
cision support utilization of rules has been low. In part,
this is due to the difficulty of writing rules without an
ontology. Consider writing a rule that would generate
a list of all patients with lung disease who are also on
blood pressure medication. With ontology this is a sim-
ple task; without one, it requires compiling exhaustive
lists of all possible lung diseases and all possible blood
pressure medications, and then mapping this back to
specific field names in the medical record. These lists
would have to be maintained as new drugs come on the
market or new conditions identified.
77.2.4 Knowledge Generation
Knowledge generation uses information technology to
augment pulling information from the clinicians’ under-
standing of the medical literature. The daily activities of
patient care generate new information about individual
patients and entire populations, but the sheer quantity of
data is overwhelming. Once data has been digitized, IT
systems can synthesize even massive amounts of infor-
mation into usable insight through two key routes: data
analysis and visualization.
Other industries rely on data analysis and data min-
ing as a significant source of insight. In healthcare,
where an operating margin of 2–4% is considered ex-
ceptional, many institutions have implemented some
level of financial data analysis. With respect to clinical
data, however, the lack of digitized information has hin-
dered the use of data analysis to generate insight about
clinical practices outside the academic research setting.
Data analysis can enable clinicians to make in-
quiries about the efficacy of daily practice in the same
waythat knowledge canbe derivedfrom controlled clin-
ical trials. A review of data at the population level can
reveal patterns that are not observed in isolation. In this
way the process of managing patients leads to greater
insight about the best way to manage those patients
going forward.
For example, it is widely recognized that induc-
ing labor before the 39th week of pregnancy can have
deleterious effects that compromise the health of the
newborn, yet early inductions still occur with relatively
high frequency. In a one-on-one interaction between pa-
tient and physician, it is easy to find reasons to induce
this patient’s labor early: late stages of pregnancy are
increasingly uncomfortable for the woman, the doctor
will be out of town, or other calendar issues come into
play. With each individualdecision, neitherprovider nor
patient is looking at the bigger picture.
At Intermountain Healthcare, an integrated delivery
network headquartered in Salt Lake City, data analysis
of almost 40000 delivers deliveries in 2000–2001 re-
vealed an increased rate of neonatal intensive care unit
(NICU) admission for babies induced before week 39
(Fig.77.5). The research revealed a one to two weeks
delay could have avoided as many as 220 NICU ad-
missions. Once this data was available, the institution
developed and implemented an evidence-based triage
protocol that set a higher threshold for elective induc-
tions. The rate of early elective inductions dropped
to 5%, significantly reducing unnecessary NICU ad-
missions. Thus, the generation of knowledge through
population data analysis also led to insight that moti-
vated providers to change their practice.
Looking for trends also enables institutions to
improve management of patient care. Ideally, these
insights will extend beyond clinical knowledge to en-
compass the process of care. Electronic medical records
enable institutions to capture information about cost,
clinical data resource utilization, and process times. Us-
ing this information, they can begin to understand the
consequences of practice patterns and resource alloca-
tions, and assess the value of the care they deliver. As
institutions often focus on cutting costs without know-
ing the impact on quality, the analysis can demonstrate
ways to provide the right kind of care while actually
driving down per capita cost. Many examples exist
where institutions deliver the highest quality of care
with the lowest per capita rates, as consists of com-
plicated processes, resource allocations and practice
patterns with multi-fauceted practice patterns attribute.
This analysis will become essential as more third-party
payers adopt pay-for-performance programs that base
reimbursement on quality rather than quantity of care.
With paper records, physicians write summaries that
effectively filter out much of the granular information
about a patient’s condition. Other information may be
stored in archives, or simply filed in some other part of
the healthcare organization where they cannot be im-
mediately accessed. One of the paradoxes of digitizing
information is that the amount of accessible informa-
tion quickly becomes so overwhelming that clinicians
can no longer sift through all of it to find the relevant
information they need.
Data analysis can also be used to help providers
synthesize the vast amounts of information that are
Part H 77.2