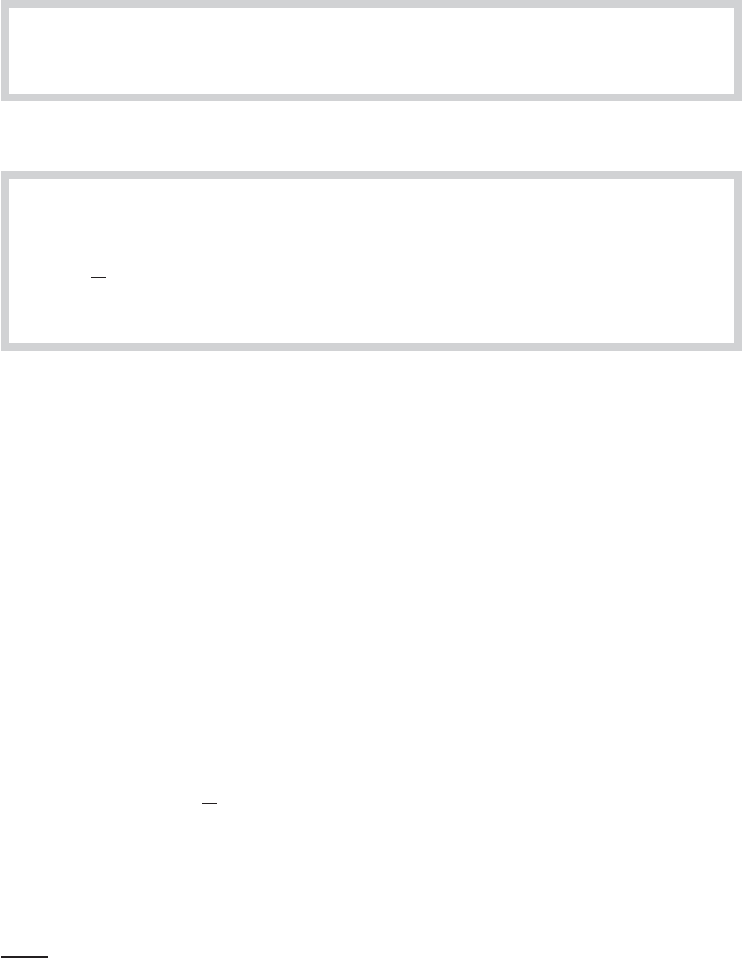
916
PART V
✦
Time Series and Macroeconometrics
DEFINITION 20.5
Martingale Difference Sequence
A vector sequence z
t
is a martingale difference sequence if E [z
t
|z
t−1
, z
t−2
,...]=0.
With Definition 20.5, we have the following broadly encompassing result:
THEOREM 20.3
Martingale Difference Central Limit Theorem
If z
t
is a vector valued stationary and ergodic martingale difference sequence, with
E [z
t
z
t
] =, where is a finite positive definite matrix, and if
¯
z
T
=(1/ T)
T
t=1
z
t
,
then
√
T
¯
z
T
d
−→ N[0,]. [For discussion, see Davidson and MacKinnon (1993,
Sections. 4.7 and 4.8).]
7
Theorem 20.3 is a generalization of the Lindeberg–Levy central limit theorem. It is not
yet broad enough to cover cases of autocorrelation, but it does go beyond Lindeberg–
Levy, for example, in extending to the GARCH model of Section 20.13.3. [Forms of
the theorem that surpass Lindeberg–Feller (D.19) and Liapounov (Theorem D.20) by
allowing for different variances at each time, t, appear in Ruud (2000, p. 479) and White
(2001, p. 133). These variants extend beyond our requirements in this treatment.] But,
looking ahead, this result encompasses what will be a very important application. Sup-
pose in the classical linear regression model, {x
t
}
t=∞
t=−∞
is a stationary and ergodic mul-
tivariate stochastic process and {ε
t
}
t=∞
t=−∞
is an i.i.d. process—that is, not autocorrelated
and not heteroscedastic. Then, this is the most general case of the classical model that
still maintains the assumptions about ε
t
that we made in Chapter 2. In this case, the
process {w
t
}
t=∞
t=−∞
={x
t
ε
t
}
t=∞
t=−∞
is a martingale difference sequence, so that with suffi-
cient assumptions on the moments of x
t
we could use this result to establish consistency
and asymptotic normality of the least squares estimator. [See, e.g., Hamilton (1994,
pp. 208–212).]
We now consider a central limit theorem that is broad enough to include the case
that interested us at the outset, stochastically dependent observations on x
t
and auto-
correlation in ε
t
.
8
Suppose as before that {z
t
}
t=∞
t=−∞
is a stationary and ergodic stochastic
process. We consider
√
T
¯
z
T
. The following conditions are assumed:
9
1. Asymptotic uncorrelatedness: E [z
t
|z
t−k
, z
t−k−1
,...] converges in mean square to
zero as k →∞. Note that is similar to the condition for ergodicity. White (2001)
demonstrates that a (nonobvious) implication of this assumption is E [z
t
] = 0.
7
For convenience, we are bypassing a step in this discussion—establishing multivariate normality requires that
the result first be established for the marginal normal distribution of each component, then that every linear
combination of the variables also be normally distributed (See Theorems D.17 and D.18A.). Our interest at
this point is merely to collect the useful end results. Interested users may find the detailed discussions of the
many subtleties and narrower points in White (2001) and Davidson and MacKinnon (1993, Chapter 4).
8
Detailed analysis of this case is quite intricate and well beyond the scope of this book. Some fairly terse
analysis may be found in White (2001, pp. 122–133) and Hayashi (2000).
9
See Hayashi (2000, p. 405) who attributes the results to Gordin (1969).