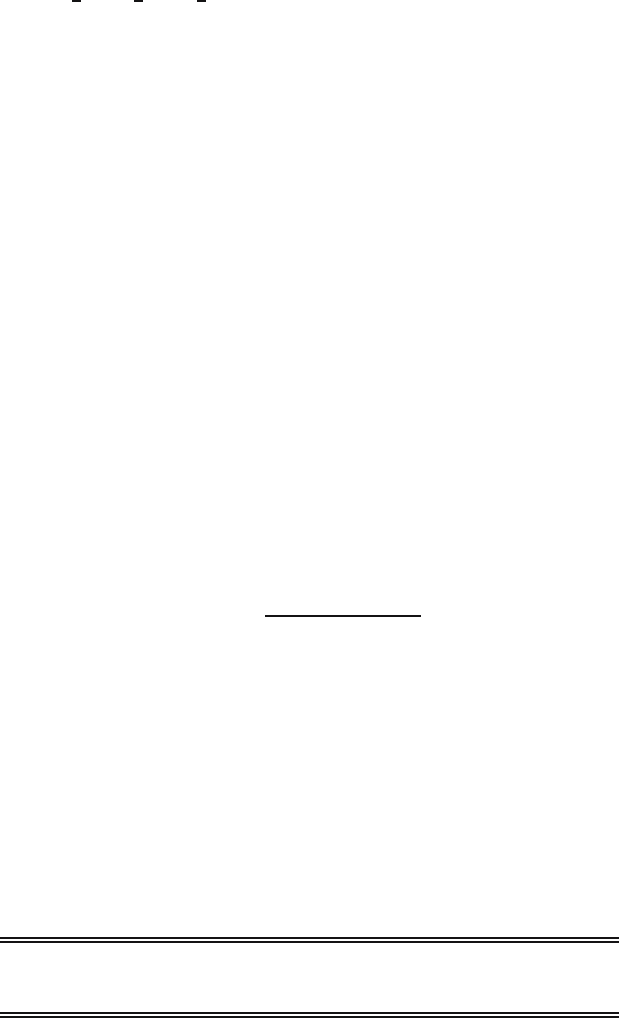
19.6 Exercises 295
a. Is S =
1
2
X
1
+
1
3
X
2
+
1
6
X
3
an unbiased estimator for µ?
b. Under what conditions on constants a
1
,a
2
,...,a
n
is
T = a
1
X
1
+ a
2
X
2
+ ···+ a
n
X
n
an unbiased estimator for µ?
19.3 Suppose the random variables X
1
,X
2
,...,X
n
have the same expec-
tation µ. For which constants a and b is
T = a(X
1
+ X
2
+ ···+ X
n
)+b
an unbiased estimator for µ?
19.4 Recall Exercise 17.5 about the number of cycles to pregnancy. Suppose
the dataset corresponding to the table in Exercise 17.5 a is modeled as a
realization of a random sample X
1
,X
2
,...,X
n
from a Geo(p) distribution,
where 0 <p<1 is unknown. Motivated by the law of large numbers, a
natural estimator for p is
T =1/
¯
X
n
.
a. Check that T is a biased estimator for p and find out whether it has
positive or negative bias.
b. In Exercise 17.5 we discussed the estimation of the probability that a
woman becomes pregnant within three or fewer cycles. One possible esti-
mator for this probability is the relative frequency of women that became
pregnant within three cycles
S =
number of X
i
≤ 3
n
.
Show that S is an unbiased estimator for this probability.
19.5 Suppose a dataset is modeled as a realization of a random sample
X
1
,X
2
,...,X
n
from an Exp(λ) distribution, where λ>0 is unknown. Let
µ denote the corresponding expectation and let M
n
denote the minimum of
X
1
,X
2
,...,X
n
. Recall from Exercise 8.18 that M
n
has an Exp(nλ) distribu-
tion. Find out for which constant c the estimator
T = cM
n
is an unbiased estimator for µ.
19.6 Consider the following dataset of lifetimes of ball bearings in hours.
6278 3113 5236 11584 12628 7725 8604 14266 6125 9350
3212 9003 3523 12888 9460 13431 17809 2812 11825 2398
Source: J.E. Angus. Goodness-of-fit tests for exponentiality based on a loss-
of-memory type functional equation. Journal of Statistical Planning and In-
ference, 6:241-251, 1982; example 5 on page 249.