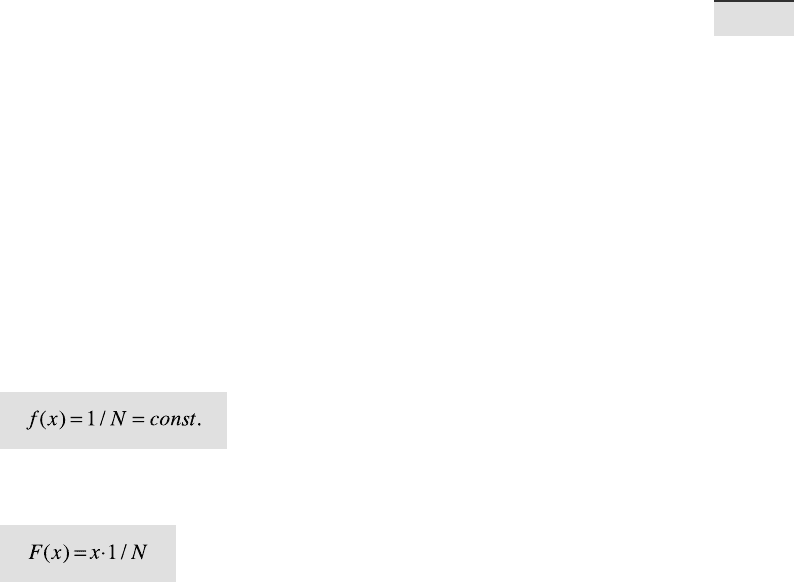
3.4 THEORETICAL DISTRIBUTIONS 51
3 UNIVARIATE STATISTICS
ans =
17.7569
e most signi cant change observed is in the mean (16.3411), which is sub-
stantially lower due to the presence of the outlier. is example clearly dem-
onstrates the sensitivity of the mean to outliers. In contrast, the median of
16.9722 is relatively una ected.
3.4 Theoretical Distributions
We have now described the empirical frequency distribution of our sample.
A histogram is a convenient way to depict the frequency distribution of the
variable x. If we sample the variable su ciently o en and the output ranges
are narrow, we obtain a very smooth version of the histogram. An in nite
number of measurements N→ ∞ and an in nitely small class width produce
the random variable’s probability density function (PDF). e probability
distribution density f(x) de nes the probability that the variable has a value
equal to x. e integral of f(x) is normalized to unity, i. e., the total number
of observations is one. e cumulative distribution function (CDF) is the
sum of the frequencies of a discrete PDF or the integral of a continuous PDF.
e cumulative distribution function F(x) is the probability that the vari-
able will have a value less than or equal to x.
As a next step, we need to nd appropriate theoretical distributions that
t the empirical distributions described in the previous section. is sec-
tion therefore introduces the most important theoretical distributions and
describes their application.
Uniform Distribution
A uniform or rectangular distribution is a distribution that has a constant
probability (Fig. 3.4). e corresponding probability density function is
where the random variable x has any of N possible values. e cumulative
distribution function is
e probability density function is normalized to unity