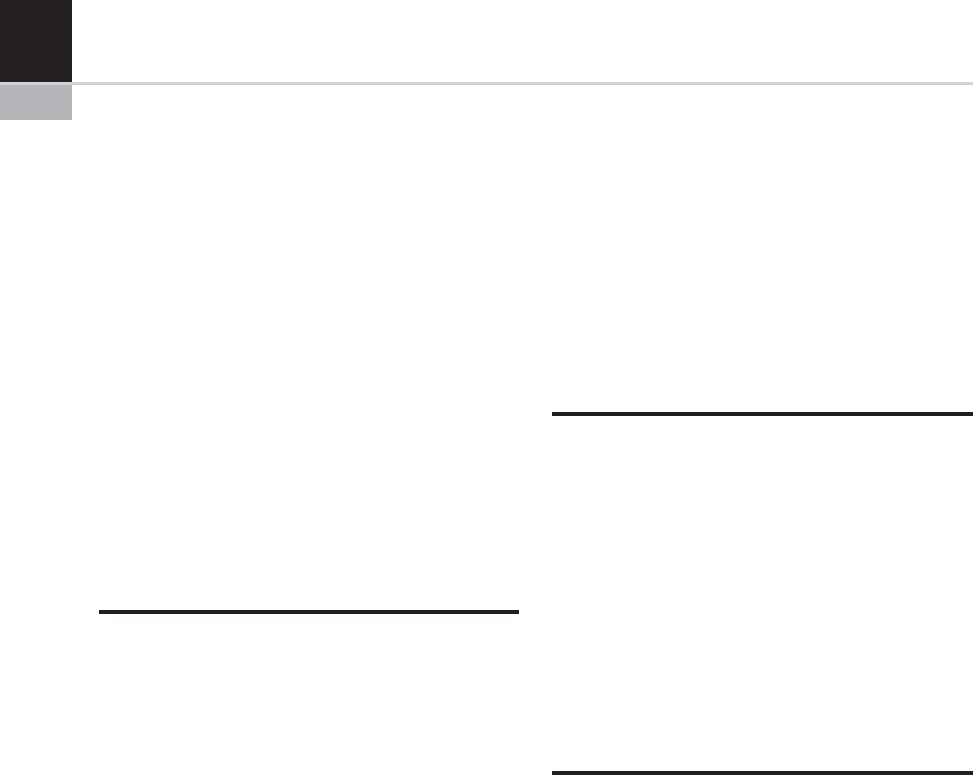
Figure 22-23 shows the applied load force
(dashed line) and its estimate (solid line). The
signal is modulated by the control signal on the
piezo-element. Figure 22-24 shows the estimation
of the piezoelectric coefficient (solid line) and its
true value (dashed line). The variation corre-
sponds to a fault which changes the coefficient
from 7 N/V to 5.25 N/V. As shown in the figure,
the convergence time to the second value is 0.3 s
because the mover is controlled by an ON/OFF
signal and the estimated curve tends to reach the
right value only when the control is ON. This
means that the system needs to be in an excited
state in order to enable possible fault detection.
Figure 22-25 shows the estimatio n of the stiff-
ness coefficient. The very low convergence time
(below 0.1 s) shows the high dynamic capa bility
of the Kalman estimator.
CONCLUSIONS
Testing for micro-manufacturing processes is
characterized by requirements on:
*
precision;
*
non-invasiveness;
*
being contactless;
*
minimization of the number of sensors;
*
low complexity.
Non-contact measurement techniques based
on laser sensors and precise movement systems
represent the best choice in order to achieve a
complete testing syst em based on direct measure-
ment. When a great number of signals have to be
acquired in order to improve the monitoring of
the normal working conditions of devices or com-
plex machines, model-based testing can be used to
reduce the number of direct measurements (with-
out a reduction of the number of interested sig-
nals).
Model-based testing and diagnosis for micro-
manufacturing may exploit advantages from the
application of estimations techniques in order to
extract features from modeled systems. The
reduction of the number of sensors is possible by
increasing the information flow from the tested
system. This information could be well repre-
sented by a proper system model. Estimation tech-
niques, such as the Kalman filters, can aid the
extraction of variables and parameters which can-
not be measured in a direct way, allowing a num-
ber of available features greater than the number
of signals measu red.
The application of model-based testing and
diagnostic procedures to the micro-/meso-devices
world represents a very efficient and powerful
way to increase qua lity and reliability, while at
the same time reducing system complexity.
ACKNOWLEDGMENTS
Special thanks to Professor Biagio Turchiano, Full
Professor of Automatic Control and Head of
Department, Dipartimento di Elettrotecnica ed
Elettronica, Politecnico di Bari, Italy, and to Pro-
fessor Mauro Onori, Associate Professor, Evolv-
able Production Systems Group, KTH, Stockholm,
Sweden. Thanks also to colleague Eng. Orlando
Petrone, Mechatronic Group leader of MASMEC
Research and Development Department. Many
issues included in this work are related to results
achieved by MASMEC Srl, Bari, Italy, for the
European Research Project MASMICRO.
REFERENCES
[1] J.S. Oakland, Statistical Process Control, Butter-
worth-Heinemann (1996).
[2] R. Isermann, Model-Based Fault Detection and Diag-
nosis – Status and Applications, IFAC (2004).
[3] Chen, C.H., Pau, L.F., Wang, P.S.P., (eds.), The
Handbook of Pattern Recognition and Computer
Vision (2nd Ed.), World Scientific Publishing Co
(1998) 207–248.
[4] D. Sarid, Scanning Force Microscopy, Oxford Series
in Optical and Imaging Sciences, Oxford University
Press, New York (1991).
[5] V. Venkatasubramanian, R. Rengaswamy, K. Yin,
S.N. Kavuri, A review of process fault detection and
diagnosis, Part I: Quantitative model-based methods,
Computers and Chemical Engineering 27 (2003)
293–311.
[6] L. Ljung, System Identification, Theory for the User,
(2nd ed)., Thomas, Kailath, (Ed.) Prentice Hall PTR
(1999).
[7] G. Bishop, G. Welch, An introduction to the Kalman
Filter, Technical report, Department of Computer
Science, University of North Carolina at Chapel Hill,
Chapel Hill, NC 27599-3175 (May 2003).
364 CHAPTER 22 Testing and Diagnosis for Micro-Manufacturing Systems