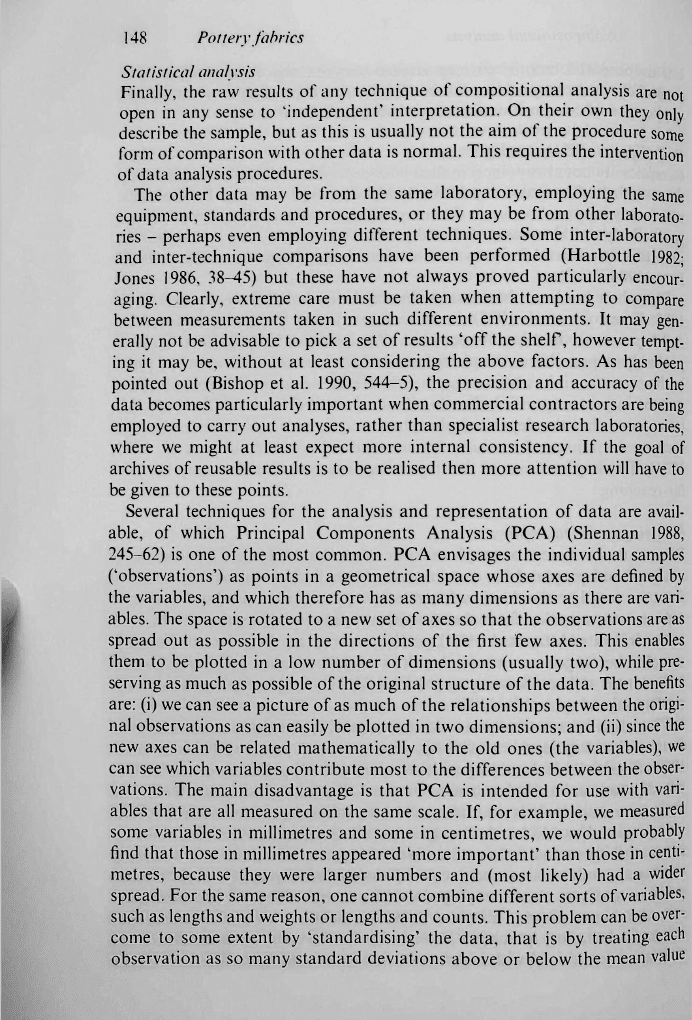
148 Pottery fabrics
Statistical analysis
Finally, the raw results of any technique of compositional analysis are not
open in any sense to 'independent' interpretation. On their own they only
describe the sample, but as this is usually not the aim of the procedure some
form of comparison with other data is normal. This requires the intervention
of data analysis procedures.
The other data may be from the same laboratory, employing the same
equipment, standards and procedures, or they may be from other laborato-
ries - perhaps even employing different techniques. Some inter-laboratory
and inter-technique comparisons have been performed (Harbottle 1982;
Jones 1986, 38-45) but these have not always proved particularly encour-
aging. Clearly, extreme care must be taken when attempting to compare
between measurements taken in such different environments. It may gen-
erally not be advisable to pick a set of results 'off the shelf, however tempt-
ing it may be, without at least considering the above factors. As has been
pointed out (Bishop et al. 1990, 544-5), the precision and accuracy of the
data becomes particularly important when commercial contractors are being
employed to carry out analyses, rather than specialist research laboratories,
where we might at least expect more internal consistency. If the goal of
archives of reusable results is to be realised then more attention will have to
be given to these points.
Several techniques for the analysis and representation of data are avail-
able, of which Principal Components Analysis (PCA) (Shennan 1988,
245-62) is one of the most common. PCA envisages the individual samples
('observations') as points in a geometrical space whose axes are defined by
the variables, and which therefore has as many dimensions as there are vari-
ables. The space is rotated to a new set of axes so that the observations are as
spread out as possible in the directions of the first few axes. This enables
them to be plotted in a low number of dimensions (usually two), while pre-
serving as much as possible of the original structure of the data. The benefits
are: (i) we can see a picture of as much of the relationships between the origi-
nal observations as can easily be plotted in two dimensions; and (ii) since the
new axes can be related mathematically to the old ones (the variables), we
can see which variables contribute most to the differences between the obser-
vations. The main disadvantage is that PCA is intended for use with vari-
ables that are all measured on the same scale. If, for example, we measured
some variables in millimetres and some in centimetres, we would probably
find that those in millimetres appeared 'more important' than those in centi^
metres, because they were larger numbers and (most likely) had a wider
spread. For the same reason, one cannot combine different sorts of variables,
such as lengths and weights or lengths and counts. This problem can be over-
come to some extent by 'standardising' the data, that is by treating each
observation as so many standard deviations above or below the mean value