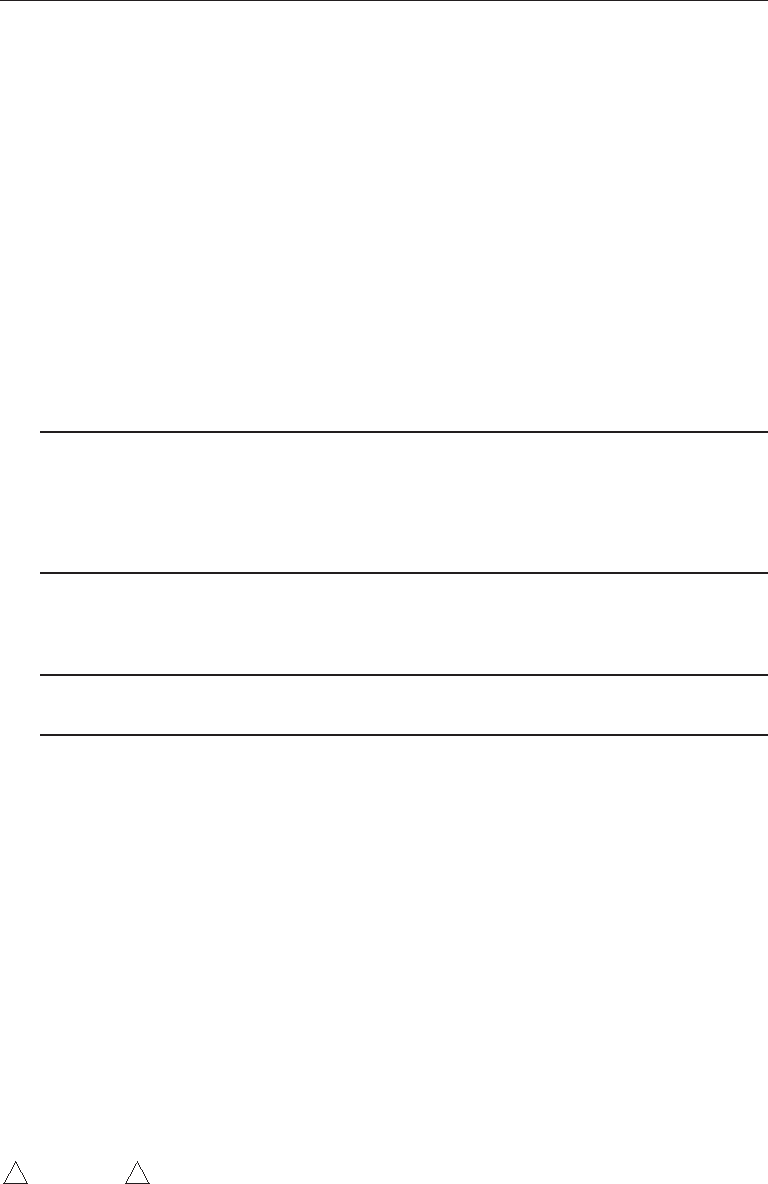
Mean Structures and Latent Growth Models 303
2. It is theoretically possible to analyze means of observed variables in a path anal-
ysis, but this is rarely done in practice. It is more common in SEM to estimate means of
latent variables (factors) represented in measurement models.
3. Special forms of maximum likelihood (ML) estimation for raw data files with
missing observations, including the expectation–maximization (EM) algorithm, esti-
mate both covariances and means. That is, they add a mean structure to the model.
Depending on how these special methods are implemented in a particular SEM com-
puter tool, it may or may not be necessary to explicitly specify a mean structure even if
the original model has only a covariance structure.
IdentIFICatIon oF Mean struCtures
The two principles listed next concern identification of mean structures:
The parameters of a model with a mean structure include (1) the (Rule 11.7)
means of the exogenous variables, (2) the intercepts of the
endogenous variables, and (3) the number of parameters in the
covariance portion of the model counted in the usual way for that
type of model.
A simple rule for counting the number of observations available to estimate the param-
eters of a model with both covariance and mean structures is stated next:
If v is the number of observed variables, then the number of (Rule 11.8)
observations equals v (v + 3)/2 when means are analyzed.
The value of the expression in Rule 11.8 gives the total number of variances, nonredun-
dant covariances, and means of observed variables. For instance, if there are three
observed variables, then there are 3(6)/2, or nine observations, including three means,
three variances, and three unique covariances (e.g., see the lower right side of Table
3.2).
In order for a mean structure to be identified, the number of its parameters can-
not exceed the total number of means of the observed variables. Also, the identifica-
tion status of a mean structure must be considered separately from that of the covari-
ance structure. For example, an overidentified covariance structure will not identify an
underidentified mean structure, and vice versa. If the mean structure is just-identified, it
has as many free parameters as observed means; therefore (1) the model-implied means
(total effects of the constant) will exactly equal the corresponding observed means; and
(2) the fit of the model with just the covariance structure will be identical to that of the
model with both the covariance structure and the mean structure.
For example, the mean structure of the model in Figure 11.1 has two parameters,
→ X and
→ Y (respectively, the mean of X, the intercept when regressing Y on