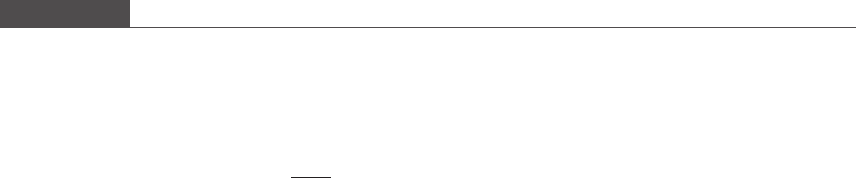
Alternatively, we can test the null hypothesis by constructing a confidence interval for the sample mean
(see Section 3.5.5 for a discussion on defining confidence intervals using the t statistic).
The 95% confidence interval for the mean population absorbance is given by
0:7 t
0:975;9
0:146
ffiffiffiffiffi
10
p
:
Using the MATLAB tinv function we get t
0:975;9
¼ 2:262.
The 95% confidence interval for the population mean absorbance is 0.596, 0.804. Since the interval
does not include 0.85, it is concluded that the hypothesized mean is significantly different from the
population mean from which the sample was drawn.
Note that the confidence interval gives us a quantitative measure of how far the hypothesized mean lies
from the confidence interval. The p value provides a probabilistic measure of observing such a difference, if
the null hypothesis is true.
Many experimental designs generate data in pairs. Paired data are produced when a
measurement is taken either from the same experimental unit twice or from a pair of
similar or identi cal units. For example, the effects of two competing drug candidates
or personal care products (e.g. lotions, sunscreens, acne creams, and pain relief
creams) can be compared by testing both products on the same individual and
monitoring various health indicators to measure the performance of each product.
If two drugs are being compared, then the experi mental design protocol usually
involves administering one drug for a certain period of time, followed by a wash-out
period to remove the remnant effects of the first drug before the second drug is
introduced. Sometimes, the potency of a drug candidate may be contrasted with the
effect of a placebo using a paired experimental design. In this way, the individuals or
experimental units also serve as their own con trol. In cross-sectional observational
studies, repeated measurements on the same individual are not possible. Pairing in
such studies usually involves finding matched controls.
Paired designs have a unique advantage over using two independent samples to
test a hypothesis regarding a treatment effect. When two measurements x
1
and x
2
are
made on the same individual under two different conditions (e.g. administration of
two different medications that are meant to achieve the same purpose, measurement
of breathing rate at rest and during heavy exercise) or at two different times (i.e.
progress in wound healing under new or old treatment methods), one measurement
can be subtracted from the other to obtain a difference that measures the effect of the
treatment. Many extraneous factors that arise due to differences among individuals,
which can potentially confound the effect of a treatment on the measured variable,
are eliminated when the difference is taken. This is because, for each individual, the
same set of extraneous variations equally affects the two measurements x
1
and x
2
.
Ideally, the difference d ¼ x
1
x
2
exclusively measur es the real effect of the treat-
ment on the individual. The observed variations in d from individual to individual
are due to biological (genetic and environmental) differences and various other
extraneous factors such as diet, age, and exercise habits.
Many extraneous factors that contribute to variations observed in the population
are uncontrollabl e and often unknown (e.g. genetic influences). When pairing data,
it is the contributions of these entrenched extraneous factors that cancel each other
and are thereby negated. Since each d value is a true measure of the treatment effect
and is minimally influenced by confounding factors, the paired design is more
efficient and lends greater precision to the experimental result than the independent
246
Hypothesis testing