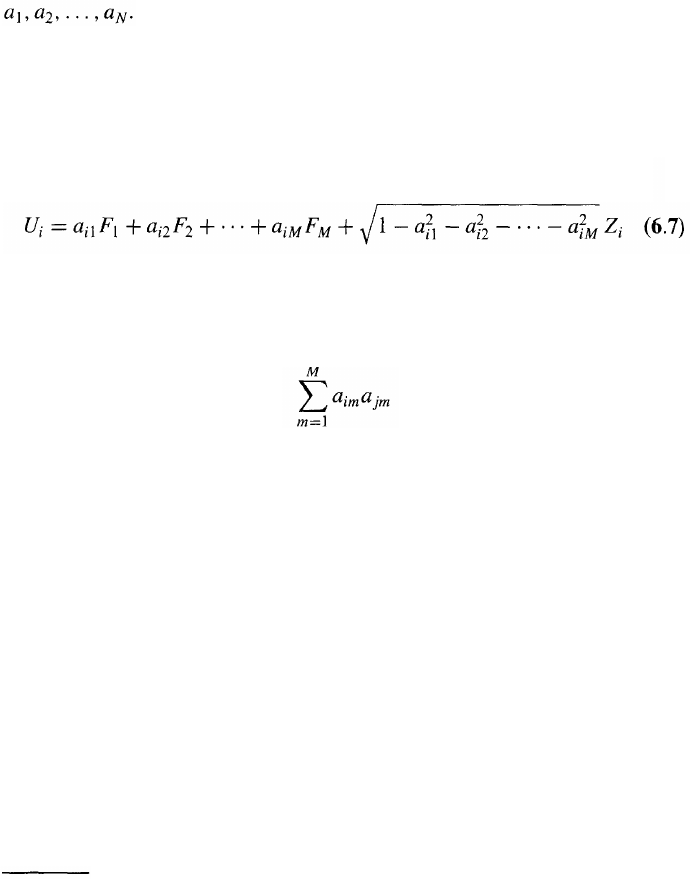
152
Chapter 6
The advantage of a one-factor model is that it imposes some structure
on the correlations. Without assuming a factor model the number of
correlations that have to be estimated for the N variables is N(N — l)/2.
With the one-factor model we need only estimate N parameters:
An example of a one-factor model from the world of
investments is the capital asset pricing model, where the return on a stock
has a component dependent on the return from the market and an
idiosyncratic (nonsystematic) component that is independent of the return
on other stocks (see Section 1.1).
The one-factor model can be extended to a two-, three-, or M-factor
model. In the M-factor model,
The factors F
1
, F
2
,..., F
M
have uncorrelated standard normal distribu-
tions and the Z
i
are uncorrelated both with each other and with the F's.
In this case the correlation between U
i
and U
j
is
6.4 COPULAS
Consider two correlated variables V\ and V
2
.The marginal distribution of
V
1
(sometimes also referred to as the unconditional distribution) is its
distribution assuming we know nothing about V
2
; similarly, the marginal
distribution of V
2
is its distribution assuming we know nothing about V
1
.
Suppose we have estimated the marginal distributions of V
1
and V
2
. How
can we make an assumption about the correlation structure between the
two variables to define their joint distribution?
If the marginal distributions of V
1
and V
2
are normal, an assumption
that is convenient and easy to work with is that the joint distribution of
the variables is bivariate normal.
4
Similar assumptions are possible for
some other marginal distributions. But often there is no natural way of
defining a correlation structure between two marginal distributions. This
is where copulas come in.
4
Although this is a convenient assumption it is not the only one that can be made. There
are many other ways in which two normally distributed variables can be dependent on
each other. See, for example, Problem 6.11.