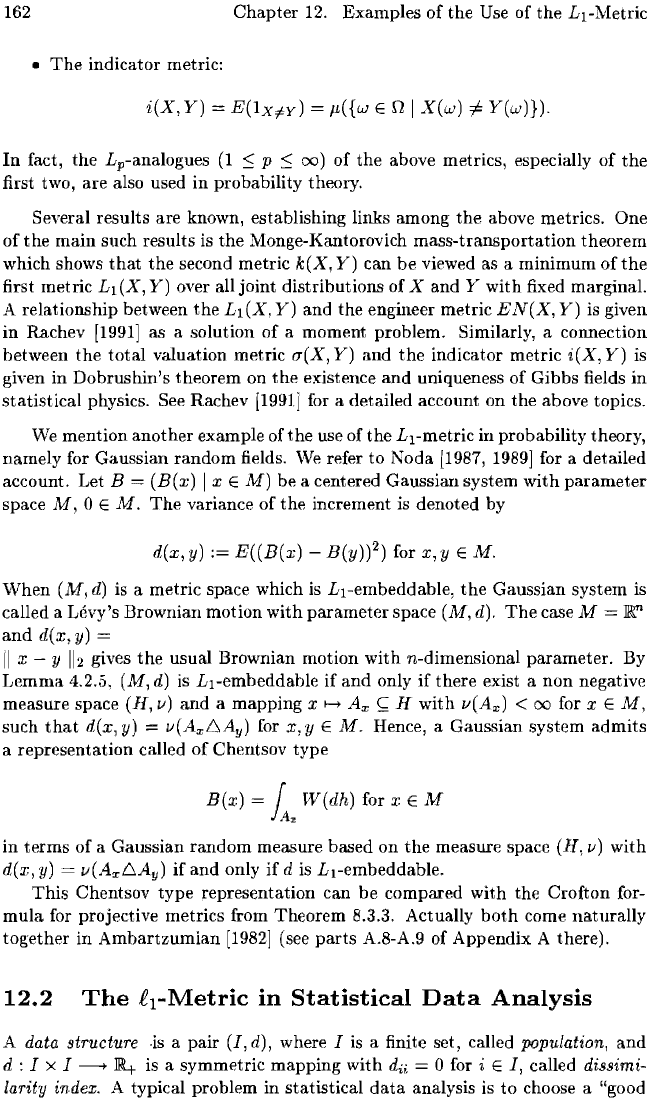
162
Chapter
12.
Examples of the Use of
the
L
1
-Metric
•
The
indicator metric:
·i(X,
Y)
=
E(lxfY)
=
11-(
{w
E n I X(w)
=1=
Yew)}).
In
fact, the Lp-analogues
(1
:::;
P
:::;
(0) of
the
above metrics, especially of
the
first two,
are
also used in probability theory.
Several results are known, establishing links among
the
above metrics. One
of
the
main such results is
the
Monge-Kantorovich mass-transportation theorem
which shows
that
the
second metric
k(X,
Y)
can
be
viewed as a minimum
of
the
first metric
L1
(X,
Y)
over all joint distributions of X and Y with fixed marginal.
A relationship between
the
L1(X,
Y)
and the engineer metric
EN
(X,
Y)
is given
in Rachev
[1991]
as a solution
of
a moment problem. Similarly, a connection
between
the
total
valuation metric O'(X,
Y)
and
the
indicator metric
i(X,
Y)
is
given in Dobrushin's theorem on
the
existence and uniqueness
of
Gibbs fields
in
statistical
physics. See Rachev
[1991]
for a detailed account on
the
above topics.
We
mention another example
of
the
use of the L1-metric in probability theory,
namely for Gaussian random fields.
We
refer to Noda [1987,
1989]
for a detailed
account. Let
B =
(B
(x) I x E
M)
be a centered Gaussian system
with
parameter
space
1\1,
0 E
M.
The
variance of the increment is denoted by
d(x,y)
E«B(x)
- B(y))2) for
x,y
EM.
When
(M,
d)
is a metric space which
is
L
1
-embeddable,
the
Gaussian system is
called a Levy's Brownian motion with
parameter
space (AI, d).
The
case M
JllI.n
and
d(x,y)
II
x - y
112
gives
the
usual Brownian motion with n-dimensional parameter. By
Lemma
4.2.5, (AI,
d)
is L1-embeddable if
and
only if there exist a non negative
measure space
(H,
v)
and a mapping x
I->
Ax
~
H with
v(Ax)
<
00
for x E
M,
such
that
d(x,y)
v(Ax6Ay)
for
x,y
E
M.
Hence, a Gaussian system
admits
a representation called
of
Chentsov type
B(x)
= r
W(dh)
for x E M
JAz
in
terms of a Ganssian
random
measure based
on
the
measure space (H, v) with
d(x,
y)
v(Ax6Ay)
if and only
if
dis
Ll-embeddable.
This
Chentsov type representation
can
be
compared
with
the Crofton for-
mula for projective metrics from Theorem 8.3.3. Actually
both
come naturally
together in Ambartzumian
[1982]
(see
parts
A.8-A.9 of Appendix A there).
12.2
The
iI-Metric
in
Statistical
Data
Analysis
A data structure
·is
a pair
(I,
d), where I is a finite set, called population,
and
d : I x I
--+
1I4
is a symmetric mapping with dii 0 for i E
I,
called dissimi-
larity index.
A typical problem in statistical
data
analysis is to choose a "good