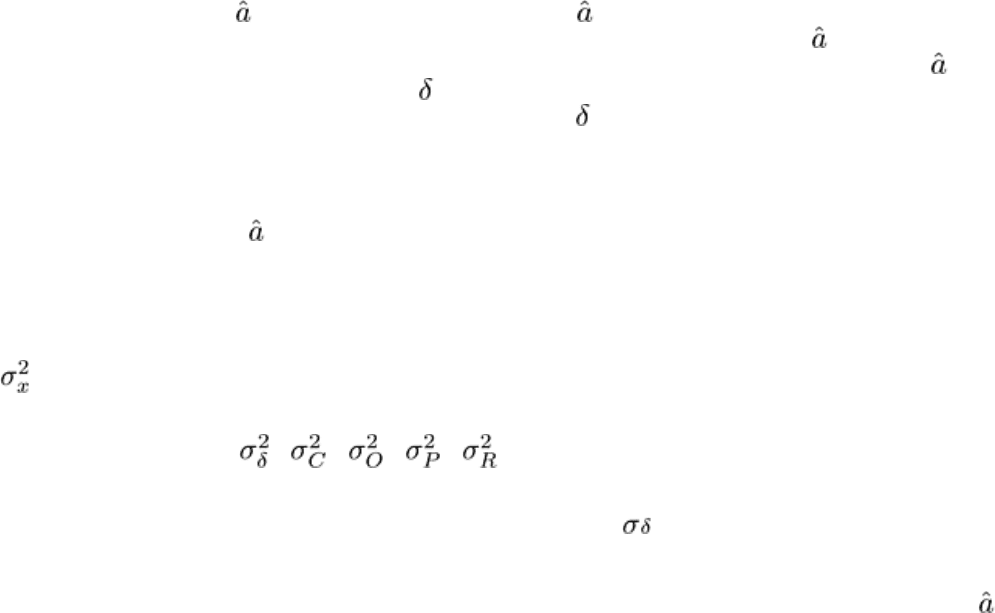
The generality of the capability characterization is limited to the application for which the experiment is representative.
Either important factors must be typical of the application or random samples must be chosen from the population of
interest and repeat inspections performed for these factors. For example, if a single inspector is used to characterize a
fluorescent penetrant inspection, it must be assumed that this inspector is typical of all the inspectors in the shop. An
alternative might be to choose a random sample of inspectors from the total pool and have each of the selected inspectors
perform the experiment.
Depending on the application of the results of the experiment, stratified sampling may be required to obtain a
representative sample. For instance, if the capability will apply to two facilities and one of them inspects twice as many
components as the other, then that facility should have twice as many inspectors in the experiment. An alternative method
is to characterize each facility independently. Care is then required in combining the results for the joint characterization.
Factorial Experiments for Hit/Miss Data. The analysis for the hit/miss data requires that all factors be balanced in
any one analysis. When practical, this can be most easily achieved by performing complete factorial experiments. For
example, Table 1 contains the results of a two-factor experiment, with the factors being cracks and inspectors. These data
can be analyzed as one data set with three inspections per crack. The resulting POD(a) function and its confidence bound
would be representative of the population of inspectors from which the sample was drawn.
If the effect of a third factor, for example, different lots of penetrants, were to be included, the entire experiment would be
repeated for each of the lots chosen at random from the population of all lots. If three lots were sampled, a total of nine
inspections would be performed on each of the flawed specimens, and the resulting POD(a) would apply to the entire
inspection process. Suppose, however, that the second and third samples of penetrant were used only by Inspector A. In
this case, the two additional sets of inspection data cannot be combined with the other three in a single analysis, because
the triple representation of Inspector A would bias the resulting POD(a) function toward his specific capability. The three
sets of inspection results for Inspector A can be combined, but the range of applicability of the answer is limited to
Inspector A (unless it can be shown or assumed that Inspector A is representative of the entire population).
When many factors must be considered, the number of possible combinations in a factorial experiment can easily become
prohibitive. More sophisticated experimental designs (fractional replications, for example) may then be required. In such
cases, the assistance of a professional statistician is recommended.
Experimental Design for Data. Inspection-result data in the form contain considerably more information than
hit/miss data and, as a consequence, permit more flexibility in the design of the experiment. In analysis, the parameters
of the POD(a) function are estimated from the slope, intercept, and standard deviation of residuals of the ln ( ) versus ln
(a) relation, as given by Eq 9, 12, and 13. In Eq 9, can be considered to be the sum of random effects, and experiments
can be designed to estimate the components of the total variation in . For example, operators, probes, and repeatability
can be jointly evaluated in a factorial experiment and their effects accounted for in the estimate of POD(a). The statistical
model for this experiment would be:
ln ( ) = β
0
+ β
1
ln (a) + C
i
+ O
j
+ P
k
+ R
l
+ (interaction terms)
(Eq 14)
where C
i
, O
j
, P
k
, and R
l
are the random effects due to cracks, operators, probes, and repeats, respectively. The random
term, δ of Eq 9, is the sum of all random effects. It can be assumed that the mean and variance of random effect X are zero
and , respectively. Then:
= + + + + . . .
(Eq 15)
Therefore, β
0
and β
1
can be estimated from a regression analysis, and can be estimated from the components of
variance using the expected mean squares for the random effects.
In principle, any statistical design from which the components of variance can be estimated can be used in an NDE
reliability experiment. However, the analysis methods would be specific to the particular design, and it is beyond the
scope of this article to address the general problem. In the section "Signal Response Analysis" in this article, it will be