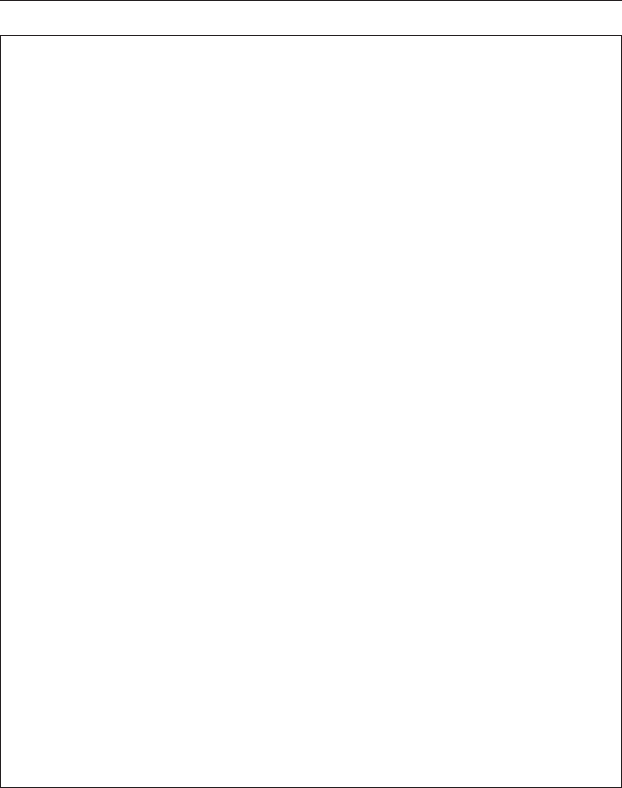
value of the F statistic will be approximately 1.0. As the slope of the line
increases (Figure 16.6(b), (c) and (d)), the numerator of Equation (16.9) will
become larger, so the value of F will also increase. As F increases, the
probability that the data have been taken from a population where the
slope of the regression line, β, is zero will decrease and will eventually be
less than 0.05. Most statistical packages will calculate the F statistic and give
the probability. There is an explanation for the number of degrees of free-
dom for the F ratio in Box 16.2.
Box 16.2 A note on the number of degrees of freedom in an
ANOVA of the slope of the regression line
The example in Section 16.6 includes an ANOVA table with an F statistic
and probability for the significance of the slope of the regression line.
Note that the “regression” mean square, which is equivalent to the
“treatment” mean square in a single-factor ANOVA, has only one degree
of freedom. This is the case for any regression analysis, despite the
sample size used for the analysis. In contrast, for a single-factor
ANOVA the number of degrees of freedom is one less than the number
of treatments. This difference needs explaining.
Forasingle-factorANOVA,allbutoneofthetreatmentmeansarefree
to vary, but the value of the “final” one is constrained because the grand
mean is a set value. Therefore, the number of degrees of freedom for the
treatment mean square is always one less than the number of treatments. In
contrast, for any regression line every value of
^
Y must (by definition) lie on
the line. For a regression line of known slope, once the first value of
^
Y has
been plotted the remainder are no longer free to vary because they must lie
on the line, so the regression mean square has only one degree of freedom.
The degrees of freedom for error in a single-factor ANOVA are the
sum of one less than the number within each of the treatments. Because a
degree of freedom is lost for every treatment, if there are a total of n
replicates (the sum of the replicates in all treatments) and k treatments,
the error degrees of freedom are n − k. In contrast, the degrees of freedom
for the residual (error) variation in a regression analysis are always n − 2.
This is because a regression line, which only ever has one degree of
freedom, is always only equivalent to an experiment with two treatments.
216 Linear regression