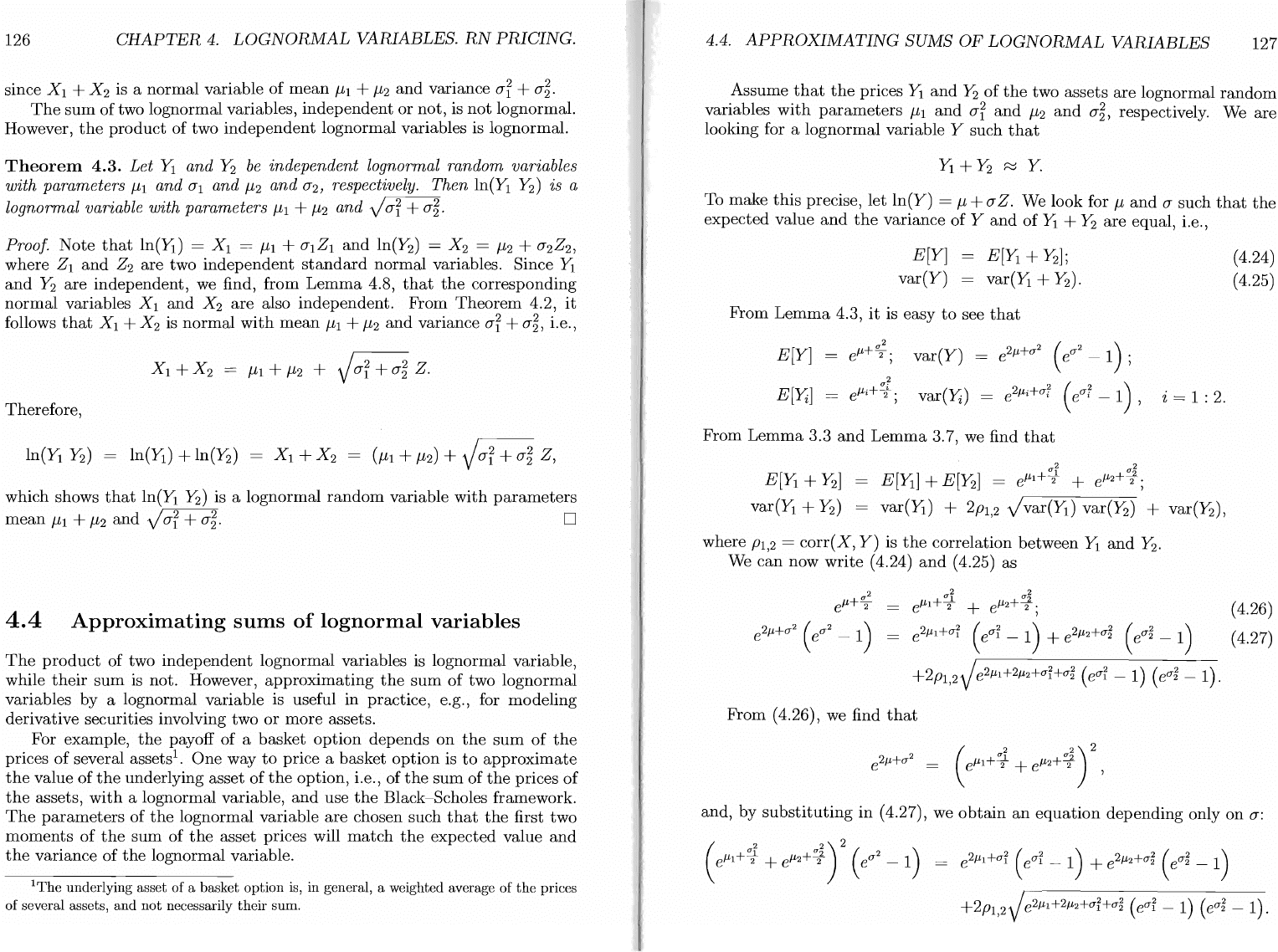
126
CHAPTER
4.
LOGNORMAL
VARIABLES.
RN
PRICING.
since
Xl
+ X
2
is a
normal
variable
of
mean
""1
+
""2
and
variance
CTt
+
CT~.
The
sum
of two lognormal variables,
independent
or
not,
is
not
lognormal.
However,
the
product
of
two
independent
lognormal variables is lognormal.
Theorem
4.3.
Let
Yi
and
Y2
be
independent lognormal random variables
with parameters
""1
and
CTI
and
""2
and
CT2J
respectively. Then In(Yi
Y2)
'is
a
lognormal variable with parameters
""1
+
""2
and V
ut
+
CT~.
Proof.
Note
that
In(Yi) =
Xl
=
""1
+
UI
Z
I
and
In(Y2)
= X
2
=
""2
+
U2Z2,
where
ZI
and
Z2
are two
independent
standard
normal
variables. Since
Yi
and
Y2
are independent, we find, from
Lemma
4.8,
that
the
corresponding
normal
variables
Xl
and
X
2
are
also independent. From
Theorem
4.2,
it
follows
that
Xl
+ X
2
is
normal
with
mean
""1
+
""2
and
variance
ut
+
CT~,
i.e.,
Therefore,
which shows
that
In(Yi
Y2)
is a lognormal
random
variable
with
parameters
mean
""1
+
""2
and
vut
+
u~.
0
4.4
Approximating
sums
of
lognormal
variables
The
product
of two
independent
lognormal variables is lognormal variable,
while
their
sum
is not. However,
approximating
the
sum
of
two lognormal
variables
by
a lognormal variable is useful
in
practice, e.g., for modeling
derivative securities involving two
or
more
assets.
For example,
the
payoff
of
a
basket
option
depends
on
the
sum
of
the
prices
of
several assets
1
.
One
way
to
price a
basket
option
is
to
approximate
the
value of
the
underlying asset of
the
option, i.e., of
the
sum
of
the
prices
of
the
assets,
with
a lognormal variable,
and
use
the
Black-Scholes framework.
The
parameters
of
the
lognormal variable
are
chosen such
that
the
first two
moments
of
the
sum
of
the
asset prices will
match
the
expected value
and
the
variance
of
the
lognormal variable.
underlying asset of a basket
option
is, in general, a weighted average of
the
prices
of
several assets,
and
not
necessarily
their
sum.
4.4.
APPROXIMATING
SUMS
OF
LOGNORMAL
VARIABLES
127
Assume
that
the
prices
Yi
and
Y2
of
the
two assets
are
lognormal
random
variables
with
parameters
""1
and
CTt
and
""2
and
u~,
respectively. We are
looking for a lognormal variable Y such
that
Yi+Y2
~
y
To
make
this
precise,
let
In(Y) =
""
+ u
Z.
We look for
""
and
u such
that
the
expected value
and
the
variance
of
Y
and
of
Yi
+
Y2
are
equal, i.e.,
E[Y]
var(Y)
E[Yi +
Y2];
var(Yi +
Y2).
From
Lemma
4.3,
it
is easy
to
see
that
E[Y]
E[Yi]
From
Lemma
3.3
and
Lemma
3.7, we find
that
0"2 0"2
E[Yi] +
E[Y2]
=
e/l
1
+-+
+
e/l
2
+-f;
i =
1:
2.
E[Yi +
Y2]
var(Yi +
Y2)
var(Yi) +
2P1,2
vvar(Yi)
var(Y2) + var(Y2),
where
P1,2
=
corr(X,
Y)
is
the
correlation between
Yi
and
Y2.
We
can
now
write
(4.24)
and
(4.25) as
O"I
O"~
e/l1+T
+
e/l
2
+
T
;
e2/l1
+ar
(ear -
1)
+
e2/l2+a~
(ea~
- 1 )
+
2P1,2!
e
2
/l
1
+2/l2+ar+a~
(ear
-
1)
(ea~
-
1).
From (4.26), we find
that
(4.24)
( 4.25)
( 4.26)
(4.27)
and, by
substituting
in
(4.27), we
obtain
an
equation
depending
only on
CT: