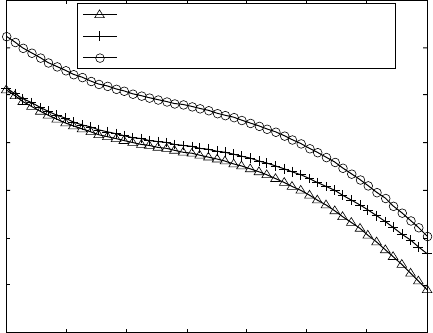
Neural network models and applications in property studies 379
On the other hand, an increase in temperature results in significant increasing
of the elongation (especially above 600 °C, see Fig. 15.6c), the reduction of
area (Fig. 15.6d), and the impact toughness (Fig. 15.6e).
At sub-zero temperatures, naturally, the influence is the opposite. The
strength properties are increased (Fig. 15.6a,b,f), while the plasticity and the
toughness of the alloys are significantly decreased (Fig. 15.6c,d,e). The
effect of the temperature calculated from the trained neural network on the
mechanical properties is consistent with what is expected from a metallurgical
point of view.
The predicted temperature dependency of the different mechanical properties
matches well with the experimental data.
Further examples are demonstrated for use of the model to predict
mechanical properties in different alloys and conditions. Quantitative
simulations of the Ti–Al–Fe system are generated (Fig. 15.7). The model
simulations show decreasing of the tensile strength and increasing of the
elongation with the temperature increase. There is a critical temperature at
around 450–500 °C; above that, the temperature influence in these directions
is stronger. The tendency in the results for these alloys is similar to earlier
modelled outcome (Fig. 15.6). The results are also consistent with a knowledge
of the influence of temperature on the Young’s modulus.
Heat treatment: Alpha+beta annealing
Ti-5Al-2.5Fe
Ti-6.4Al-1.2Fe RMI low-cost alloy
Ti-8Al-2Fe
0 100 200 300 400 500 600 700
T
(°C)
(a)
Tensile strength (MPa)
1400
1200
1000
800
600
400
200
0
15.7
Neural network predictions of the influence of the temperature
on (a) tensile strength, (b) elongation, and (c) Young’s modulus for
Ti-5Al-2.5Fe, Ti-6.4Al-1.2Fe and Ti-8Al-2Fe alloys.