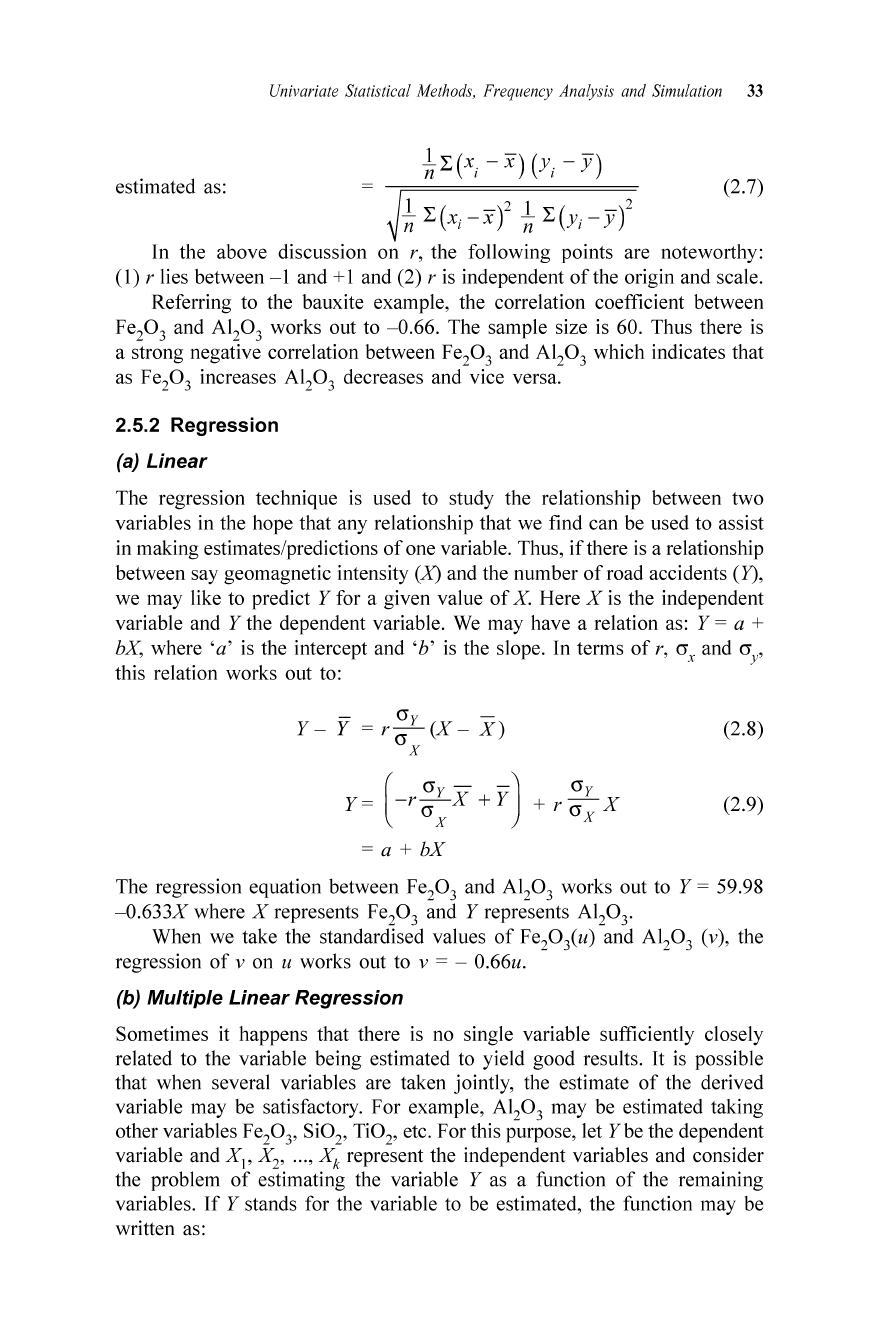
Univ
ariate Statistical Methods, Frequency Analysis and
Simu
lation 33
(2.7)
estimated as:
~*
L(X
i
-XY
*L(Yi -
yf
In the above discussion on r, the following points are noteworthy:
(I)
r lies between - I and +I and (2) r is independent
of
the origin and scale.
Referring to the bauxite example, the correlation coefficient between
Fe
20
3
and AI
20
3
works out to - 0.66. The sample size is 60. Thus there is
a strong negative correlation between Fe
20
3
and AI
20
3
which indicates that
as Fe
20
3
increases AI
20
3
decreases and vice versa.
2.5.2 Regression
(a) Linear
The regression technique is used to study the relationship between two
variables in the hope that any relationship that we find can be used to assist
in making estimates/predictions
of
one variable. Thus, ifthere is a relationship
between say geomagnetic intensity (X) and the number
of
road accidents (Y),
we may like to predict Y for a given value
of
X. Here X is the independent
variable and Y the dependent variable. We may have a relation as: Y = a +
bX
, where 'a' is the intercept and ' b' is the slope. In terms
of
r, a and a ,
x y
this relation works out to:
- a
y
-
Y - Y =
r-(X
-
X)
ax
(2.8)
(
a -
-)
Y -
-r-
y
X
+Y
- ax
= a +
bX
a
y
+ rcr
X
x
(2.9)
The regression equat ion between Fe
20
3
and AI
20
3
works out to Y = 59.98
- 0.633X where X represents
Fep
3 and Y represents
A1
20
3
.
When we take the standardised values
of
Fe
20iu)
and Al
20
3
(v), the
regression
of
v on u works out to v = - 0.66u.
(b) Multiple Linear Regression
Sometimes it happens that there is no single variable sufficiently closely
related to the variable being estimated to yield good results. It is possible
that when several variabl es are taken jointly, the estimate
of
the derived
variable may be satisfactory. For example , AI
20
3
may be estimated taking
other variables
Fep3
'
Si0
2
,
Ti0
2
,
etc. For this purpose, let
Ybe
the dependent
variable and X!' X
2
'
...
, X
k
represent the independent variables and consider
the problem
of
estimating the variable Y as a function
of
the remaining
variables. If Y stands for the variable to be estimated, the function may be
written as: