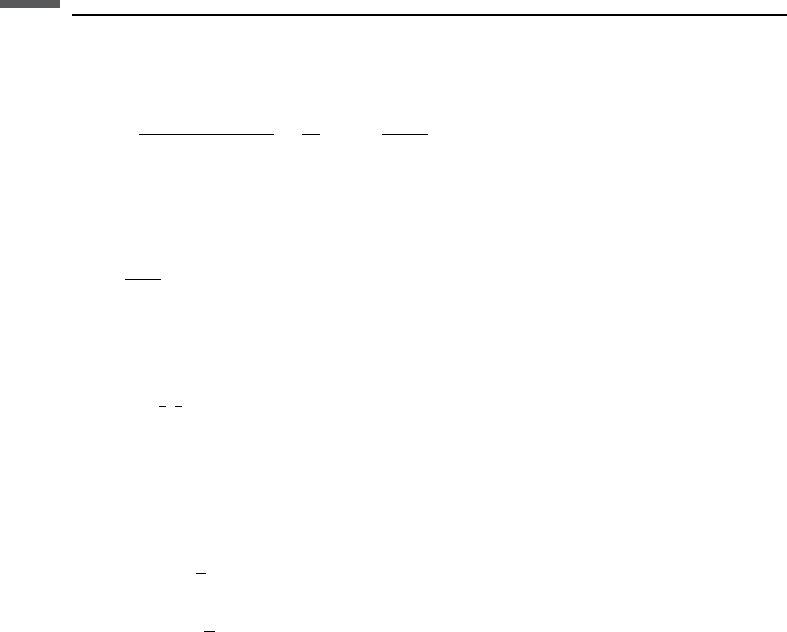
The logistic distribution is a continuous distribution with probability density
function given by
PxðÞ¼
e
xmðÞ=s
s 1 þ e
xmðÞ=s
½
2
¼
1
4s
sech
2
x m
2s
The mean m and variance s
2
of the logistic distribution are given by
m ¼m
2
¼
p
2
s
2
3
The Weibull distribution is a continuous distribution with probability density
function
PðxÞ¼
k
l
x
l
k1
e
x=lðÞ
k
; x 0
0; x < 0
(
where k > 0istheshape parameter and l > 0isthescale parameter for the distribution.
The mean m and variance s
2
of the logistic distribution are given by
m ¼l 1 þ
1
k
2
¼l
2
1 þ
2
k
m
2
where G is the gamma function. The Weibull distribution is often used to describe
failure rates. If the failure rate decreases over time, k < 1; if the failure rate is constant
k ¼1; and if the failure rate increases over time, k > 1. When k ¼3, the Weibull
distribution is a good approximation to the normal distribution, and when k ¼1, the
Weibull distribution reduces to the exponential distribution.
Monte Carlo simulations
Statistical simulation is a powerful numerical method for tackling many probabilistic
problems. One of the steps is to draw samples X
i
from a desired probability distribu-
tion function F(x). This procedure is often called Monte Carlo simulation, a term
made popular by physicists working on the bomb during the Second World War.
In general, Monte Carlo simulation can be a very difficult problem, especially when
X is multivariate with correlated components, and F(x) is a complicated function.
For the simple case of a univariate X and a completely known F(x) (either analytically
or numerically), drawing X
i
amounts to first drawing uniform random variates
U
i
between 0 and 1, and then evaluating the inverse of the desired cumulative distribution
function (CDF) at these U
i
: X
i
¼F
–1
(U
i
). The inverse of the CDF is called the quantile
function. When F
1
(X) is not known analytically, the inversion can be easily done by
15 1.3 Statistics and probability