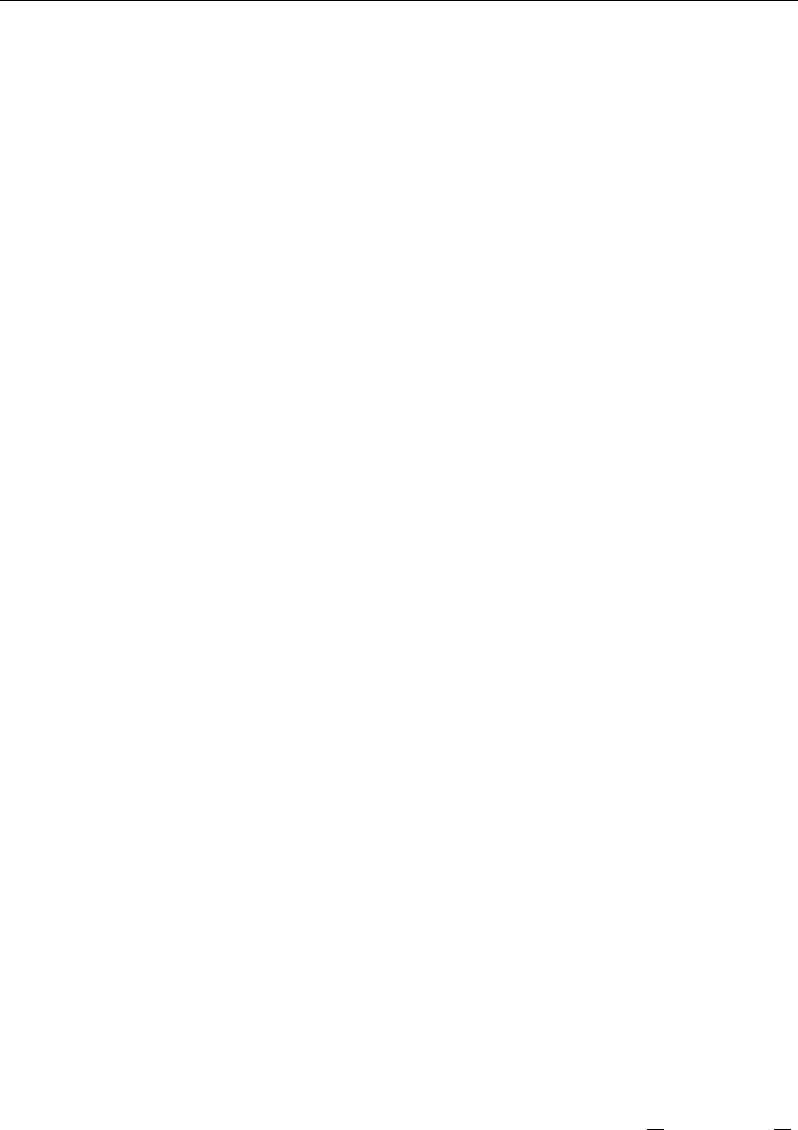
Nabil Semmar 46
IV.1.6.4. Graphical Interpretation of Factorial Plans
According to the factorial plan F1F2 of individuals (Figure 39), id1 and id2 show
opposition along F1. According to the variable plot, the variables M1 and M2 seem to be
opposite, and projected on the same sides than id2 and id1, respectively. Taking into account
the importance of variable M2 on F1, and the graphical proximity between M2 and id1, the
opposition of id1 to id2 can be explained by a high value of M2 in id1 and a low one in id2.
In fact, the initial dataset A shows values of 3 and -6 for M2 in id1 and id2, respectively.
Thus, the PCA helped to identify that the highest variability source in the dataset A consisted
of an important opposition between id1 and id2 for variable M2. In metabolomic terms, this
can correspond to a situation where some individuals are productive of a metabolite M2
whereas others are relatively deficient in M2.
For F2, the highest coordinate of corresponding eigenvector U2 concerns variable M1,
leading to deduce that the role of M1 on F2 is relatively more important than that of M2.
Graphically, the individual id2 projects closer to M1 than it is id1. This translates a higher
value of M1 in id2 than in id ; this can be checked in the initial dataset A. From this simplistic
example, variable M2 appears to play a separation role between individuals (profiles),
whereas the variable M1 seems to group the individuals according to a more or less affinity.
The fact that id1 and id2 are bot opposite alonf F2 can be attributed to their relatively close
positive values (2 and 3, respectively).
Apart from the dual analysis between rows (individuals) and columns (variables), the
interpretations in PCA can be focused on the variability of variables and individuals,
separately: on the plan F1F2 (Figure 39), the variables M1 and M2 seem to have mainly
opposite behaviours from their projections in two different parts of the plan. This opposition
is observed for individuals, and seems to indicate the presence of two trends in the initial
dataset A.
IV.1.6.5. Different Types of PCA
The variability of a dataset X (n×p) can be analysed by PCA on the basis of different
criteria by considering (Figure 40):
- The crude effects of variables leading to give more importance to the most dispersed
variables from the axes’ origin.
- The variations of data around their mean vector (centered PCA) leading to analyse
the variability of the dataset around its gravity centre GC.
- Standardized data obtained by homogenizing the variation scales of all the variables
through their weighting by their variances. This leads to analyse the variability of the
dataset around the gravity centre and within a unity scale space.
- Ranked data consisting in using the ranks of data rather than their values.
- These different PCA are performed from different square matrices (p × p):
- PCA on crude data is performed on the square matrix X’X.
- Centred PCA is performed on the square matrix C’C, with
XXC −=
, and where
is the mean vector of the different variables.