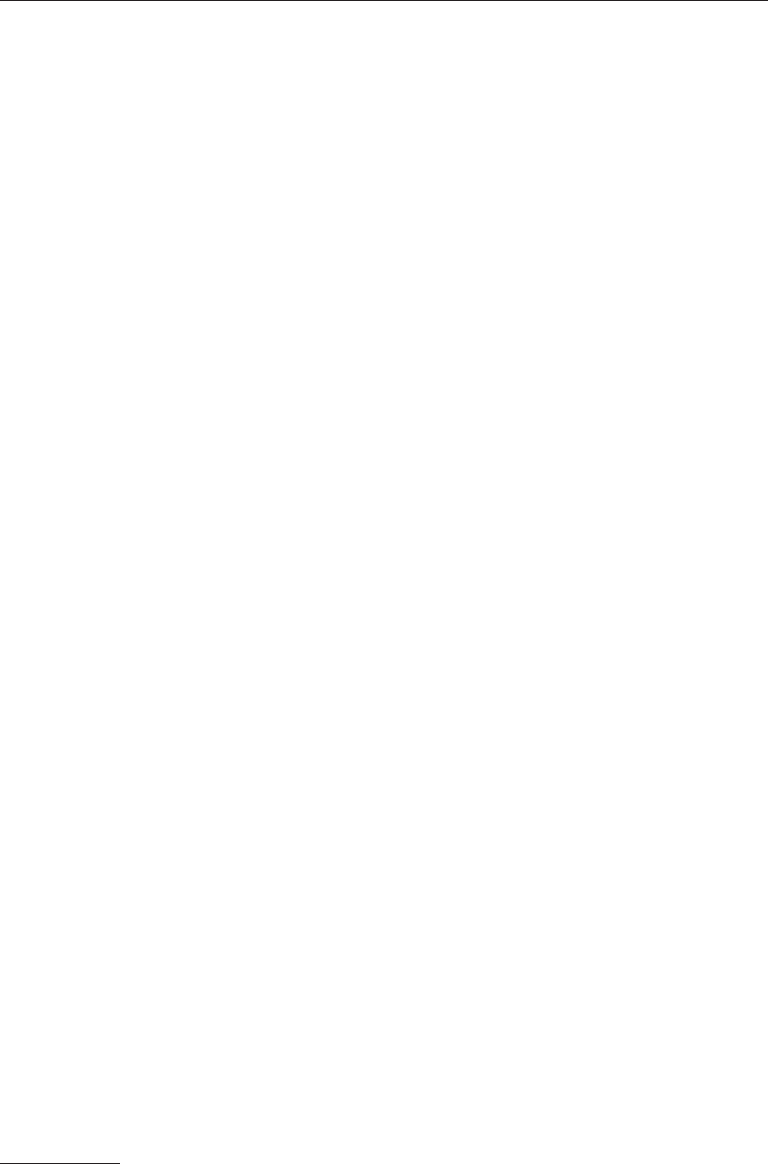
180 CORE TECHNIQUES
ous indicators of underlying latent variables in a measurement model, such as in a CFA
model or when analyzing a structural regression (SR) model (e.g., Figures 5.6, 5.8). But
parceling is controversial. The reasons why are outlined later in this chapter.
Special WLS Methods for Ordinal Outcomes
Muthén (e.g., 1984) describes an approach to estimating models with any combina-
tion of dichotomous, ordinal, or continuous outcome variables known as continuous/
categorical variable methodology (CVM). In CVM, bivariate associations among ob-
served variables are estimated with polychoric correlations, which assume that a normal,
continuous process underlies each observed variable (Flora & Curran, 2004). The model
is then estimated with a form of WLS, and values of corrected test statistics are provided.
In the CVM approach described by Muthén and Asparouhov (2002) that is imple-
mented in Mplus, each observed ordinal indicator is associated with an underlying
latent response variable, which is the underlying amount of a continuous and normally
distributed trait or characteristic that is required to respond in a certain category of
the corresponding observed ordinal item. When the observed indicator is dichotomous,
such as for items with a true–false response format, this amount, or threshold, is the
point on the latent response variable where one answer is given (e.g., true) when the
threshold is exceeded. It is also the point where the other response is given (e.g., false)
when the threshold is not exceeded (Brown, 2006). Dichotomous items have a single
threshold, but the number of thresholds for items with ≥ 3 response categories is the
number of categories minus one. Each latent response variable is in turn represented as
the continuous indicator of the underlying substantive factor that corresponds to a hypo-
thetical construct. The data matrix analyzed in this approach is an asymptotic correla-
tion matrix of the latent response variables. For dichotomous indicators, this estimated
matrix will be a tetrachoric correlation matrix; for items with at least three response
categories, the data matrix will be an estimated polychoric correlation matrix.
The arbitrary and elliptical estimators described earlier (e.g., ADF), which do not
assume normality, are also members of the WLS family of estimators.
8
The WLS estima-
tor can be applied to either continuous or ordinal outcomes because it does not assume
a particular distributional form. In general, WLS estimation is just as computationally
complex as ADF estimation, requires very large samples, and is subject to technical
problems in the analysis (e.g., Finney & DiStefano, 2006, pp. 281–288), such as the
failure of the computer to derive the inverse of the weight matrix. Muthén, du Toit, and
Spisic (1997) describe forms of robust WLS estimation that deal with problems of
using WLS when the sample size is not very large. These robust methods use somewhat
simpler matrix calculations compared with WLS estimation.
In Mplus, two of these robust estimators are designated as mean-adjusted weighted
least squares (WLSM) and mean- and variance-adjusted weighted least squares
8
The GLS estimator is also a member of this family, but it assumes multivariate normality.