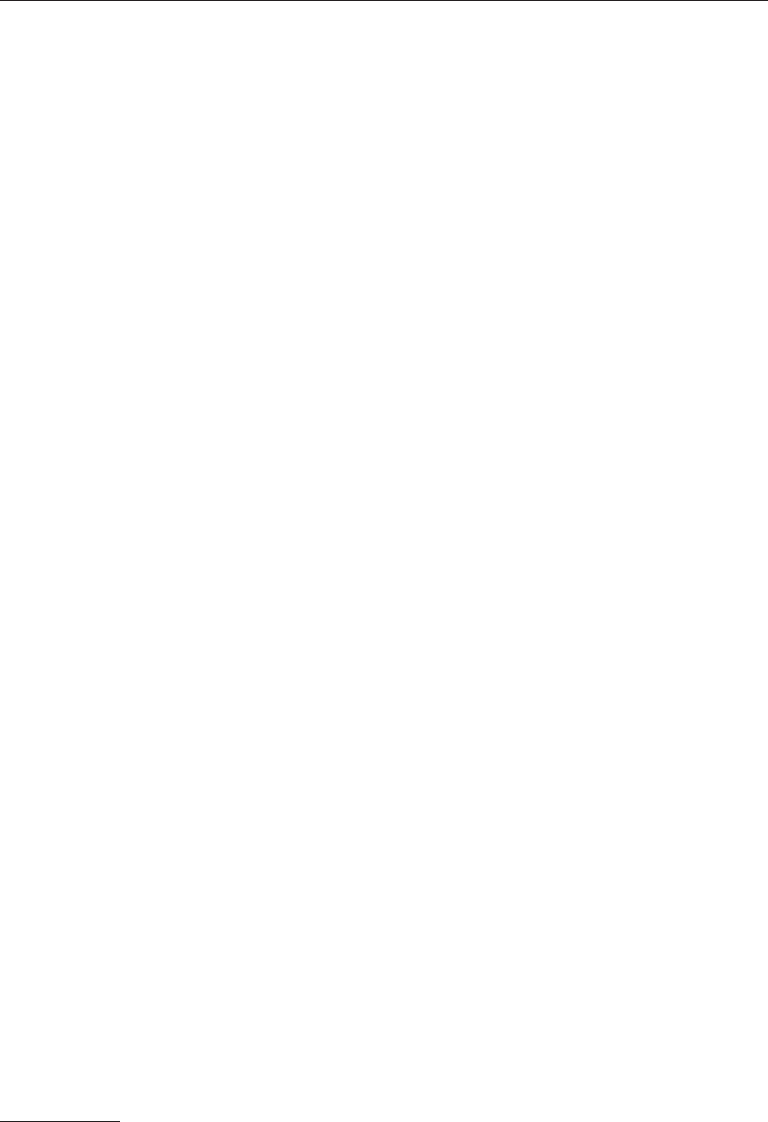
Estimation 155
continuous or if their distributions are severely non-normal, then an alternative estima-
tion method is needed.
Most forms of ML estimation in SEM are simultaneous, which means that the esti-
mates of model parameters are calculated all at once. Thus, ML estimation is a full-
information method. When all statistical requirements are met and the model is cor-
rectly specified, ML estimates in large samples are asymptotically unbiased, efficient,
and consistent.
1
In this sense, ML estimation has an advantage under these ideal condi-
tions over partial-information methods that analyze only a single equation at a time.
An example of the latter is two-stage least squares (TSLS), which was used in the late
1970s to estimate nonrecursive path models before the advent of programs such as LIS-
REL. Nowadays, ML estimation is generally used to analyze nonrecursive models. How-
ever, the TSLS method is still relevant for SEM—see Topic Box 7.1. Implications of the
difference between full- versus partial-information methods when there is specification
error are considered later in this chapter.
The criterion minimized in ML estimation, or the fit function, is related to the
discrepancy between sample covariances and those predicted by the researcher’s model.
The mathematics of ML estimation are complex, and it is beyond the scope of this sec-
tion to describe them in detail—see Nunnally and Bernstein (1994, pp. 147–155), Ferron
and Hess (2007), or Mulaik (2009, chap. 7) for more information. There are points of
contact between ML estimation and more standard methods. For example, ordinary least
squares (OLS) and ML estimates of coefficients in multiple regression (MR) analyses are
basically identical. Estimates of error variances may differ slightly in small samples, but
the two methods yield similar results in large samples.
sample variances
One difference between ML estimation and more standard statistical techniques con-
cerns estimation of the population variance σ
2
. In standard techniques, σ
2
is estimated
in a single sample as s
2
= SS/df where the numerator is the total sum of squared devia-
tions from the mean and the denominator is the overall within-group degrees of free-
dom, or N – 1. In ML estimation, σ
2
is estimated as S
2
= SS/N. In small samples, S
2
is
a negatively biased estimator of σ
2
. In large samples, however, values of s
2
and S
2
are
similar, and they are asymptotic in very large samples.
The implementations of ML estimation in some SEM computer programs, such as
Amos and Mplus, calculate sample variances as S
2
, not s
2
. Thus, variances calculated
as s
2
using a computer program for general statistical analyses, such as SPSS, may not
exactly equal those calculated in an SEM computer program as S
2
for the same data.
Check the documentation of your SEM computer tool to avoid possible confusion about
this issue.
1
A consistent estimator is one where increasing the sample size increases the probability that the estimator
is close to the population parameter, and an efficient estimator has a low error variance among results from
random samples.