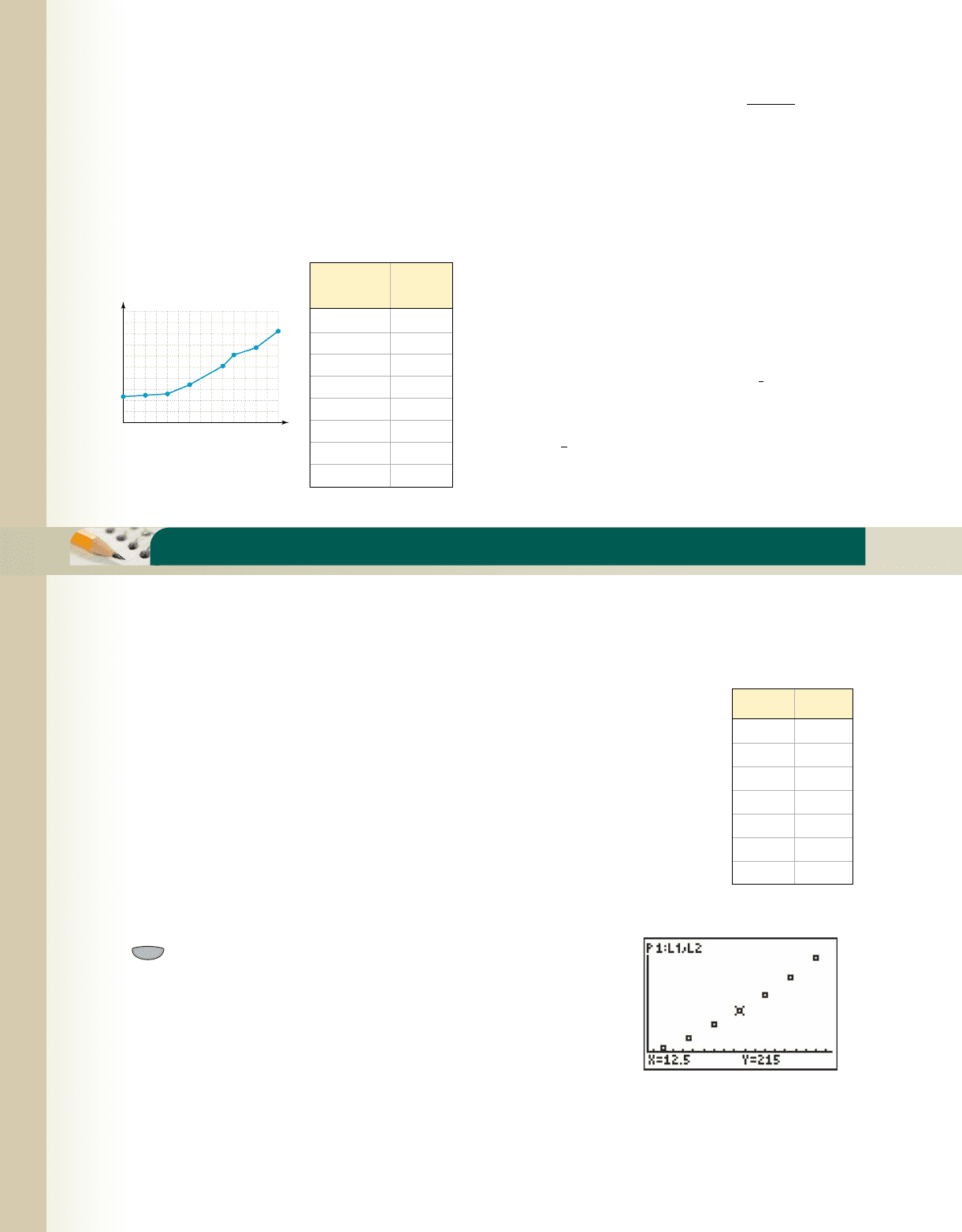
17. The graph and accompanying table show the number
N of new books published in the United States for
selected years t from 1990 to 2004. Find the average
rate of change for the years (a) 1992 to 1996 and
(b) 1996 to 1999. (c) In 1997, the number of new
books published actually fell to 65.8 (1000s). Using
this information, find the average rate of change for
the years 1996 to 1997, and 1997 to 1999.
18. Given and ,
determine and its domain.
19. Monthly sales volume for a successful new company
is modeled by , where S(t) represents
sales volume in thousands in month t (
corresponds to January 1). (a) Would you expect
the average rate of change from May to June to be
greater than that from June to July? Why?
(b) Calculate the rates of change in these intervals
to verify your answer. (c) Calculate the difference
quotient for S(t) and use it to estimate the sales
volume rate of change after 10, 18, and 24 months.
20. A snowball increases in size as it rolls downhill. The
snowball is roughly spherical with a radius that can
be modeled by the function where t is
time in seconds and r is measured in inches. The
volume of the snowball is given by the function
Use a composition to (a) write V
directly as a function of t and (b) find the volume
of the snowball after 9 sec.
V1r2⫽
4
3
r
3
.
r1t2⫽ 1t
,
t ⫽ 0
S1t2⫽ 2t
2
⫺ 3t
1f ⴰ g21x2
g1x2⫽ 13x ⫺ 1
f 1x2⫽ x
2
⫹ 2
376 CHAPTER 3 Quadratic Functions and Operations on Functions 3–96
College Algebra G&M—
Residuals, Correlation Coefficients, and Goodness of Fit
When using technology to calculate a regression equation, we must avoid relying on the correlation coefficient
as the sole indicator of how well a model fits the data. It is actually possible for a regression to have a high r-value
(correlation coefficient) but fit the data very poorly. In addition, regression models are often used
to predict future values, extrapolating well beyond the given set of data. Sometimes the model
fails miserably when extended—even when it fits the data on the specified interval very well.
This fact highlights (1) the importance of studying the behavior of the toolbox functions and
other graphs, as we often need to choose between two models that seem to fit the given data;
(2) the need to consider the context of the data; and (3) the need for an additional means to
evaluate the “goodness of fit.” For the third item, we investigate something called a residual.
As the name implies, we are interested in the difference between the outputs generated by the
equation model, and the actual data: equation value data value residual. Residuals that are
fairly random and scattered indicate the equation model has done a good job of capturing the
curvature of the data. If the residuals exhibit a detectable pattern of some sort, this often indicates
trends in the data that the equation model did not account for.
As a simplistic illustration, enter the data from Table 3.5 in L1 and L2,
then graph the scatterplot. Most graphing calculators offer a window option
specifically designed for scatterplots that will plot the points in an “ideal”
window: 9:ZoomStat (Figure 3.82). Upon inspection, it appears the data
could be linear with positive slope, quadratic with , or some other toolbox
function with increasing behavior. Many of these forms of regression will give a
correlation coefficient in the high 90s. This is where an awareness of the context
is important. (1) Do we expect the data to increase steadily over time (linear
data)? (2) Do we expect the rate of change (the growth rate) to increase over
time (quadratic data)? At what rate will values increase? (3) Do we expect the
growth to increase dramatically with time (power or exponential regression)?
Running a linear regression (LinReg L1, L2, Y
1
) gives the equation in Figure 3.83, with a very high r-value. This
model appears to fit the data very well, and will reasonably approximate the data points within the interval. But could
we use this model to accurately predict future values (do we expect the outputs to grow indefinitely at a linear rate)?
a 7 0
ZOOM
⫽⫺
21.5
570.07
⫺61.07
3.5
Figure 3.82
Table 3.5
xy
519
7.5 75
10 140
12.5 215
15 297
17.5 387
20 490
CALCULATOR EXPLORATION AND DISCOVERY
Year Books
(1000s)
0 46.7
2 49.2
4 51.7
6 68.2
9 102.0
10 122.1
12 135.1
14 165.8
11990 S 02
0
40
Years (1990 → 0)
148642
80
120
200
160
180
140
100
60
20
10 12
Books published (1000s)
cob19545_ch03_371-380.qxd 8/10/10 8:11 PM Page 376