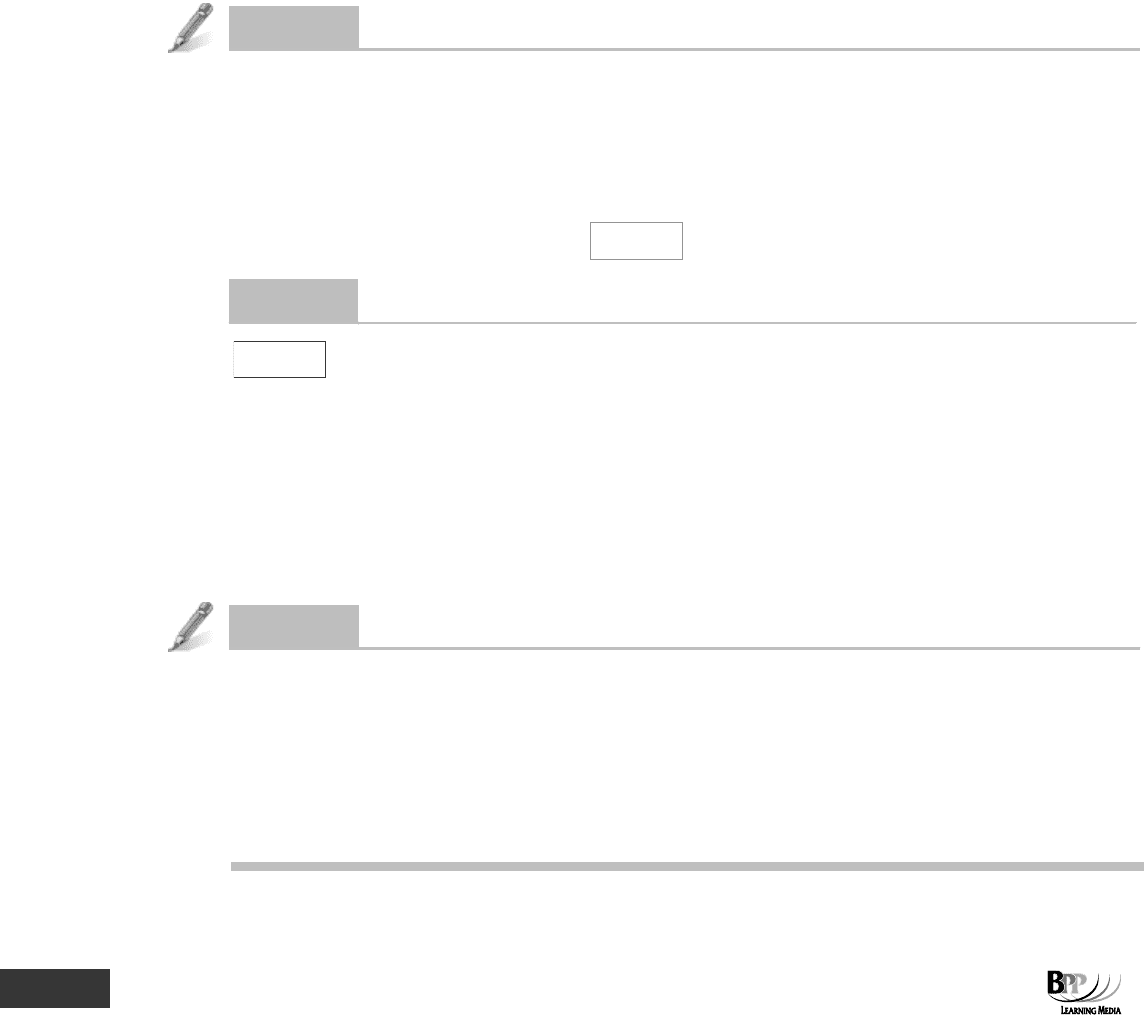
298 11: Forecasting ⏐ Part F Forecasting
4.3 Forecasting using linear regression analysis
Correlation exists in a time series if there is a relationship between the period of time and the recorded value for
that period of time. Time is the X variable and
simplified values for X are used instead of year numbers.
For example, instead of having a series of years 20X1 to 20X5, we could have values for X from 0 (20X1) to 4
(20X5).
Using linear regression analysis, a trend line is found to be y = 20 – 2.2X where X = 0 in 20X1 and Y = sales level in
thousands of units. Using the trend line, predicted sales in 20X6 (X = 5) would be:
20 – (2.2
× 5) = 9 ie 9,000 units
Predicted sales in 20X7 (year 6) would be:
20 – (2.2
× 6) = 6.8 ie 6,800 units
Question
Forecast sales
Suppose that a trend line, found using linear regression analysis, is Y = 300 – 4.7X where X is time (in quarters)
and Y = sales level in thousands of units. Given that X = 0 represents 20X0 quarter 1 and that the seasonal
variations are as set out below.
Q
1
Q
2
Q
3
Q
4
Seasonal variations ('000 units) –20 –8 +4 +15
The forecast sales level for 20X5 quarter 4 is
units
Answe
206,900
units
Working
X = 0 corresponds to 20X0 quarter 1
∴ X = 23 corresponds to 20X5 quarter 4
Trend sales level = 300 – (4.7
× 23) = 191.9 ie 191,900 units
Seasonally-adjusted sales level = 191.9 + 15 = 206.9 ie 206,900 units
Question
Forecasting
Over a 36-month period, sales have been found to have an underlying linear trend of Y = 14.224 + 7.898X, where Y
is the number of items sold and X represents the month. Monthly deviations from trend have been calculated and
month 37 is expected to be 1.28 times the trend value.
The forecast number of items to be sold in month 37 is approximately
A 389 C 391
B 390 D 392