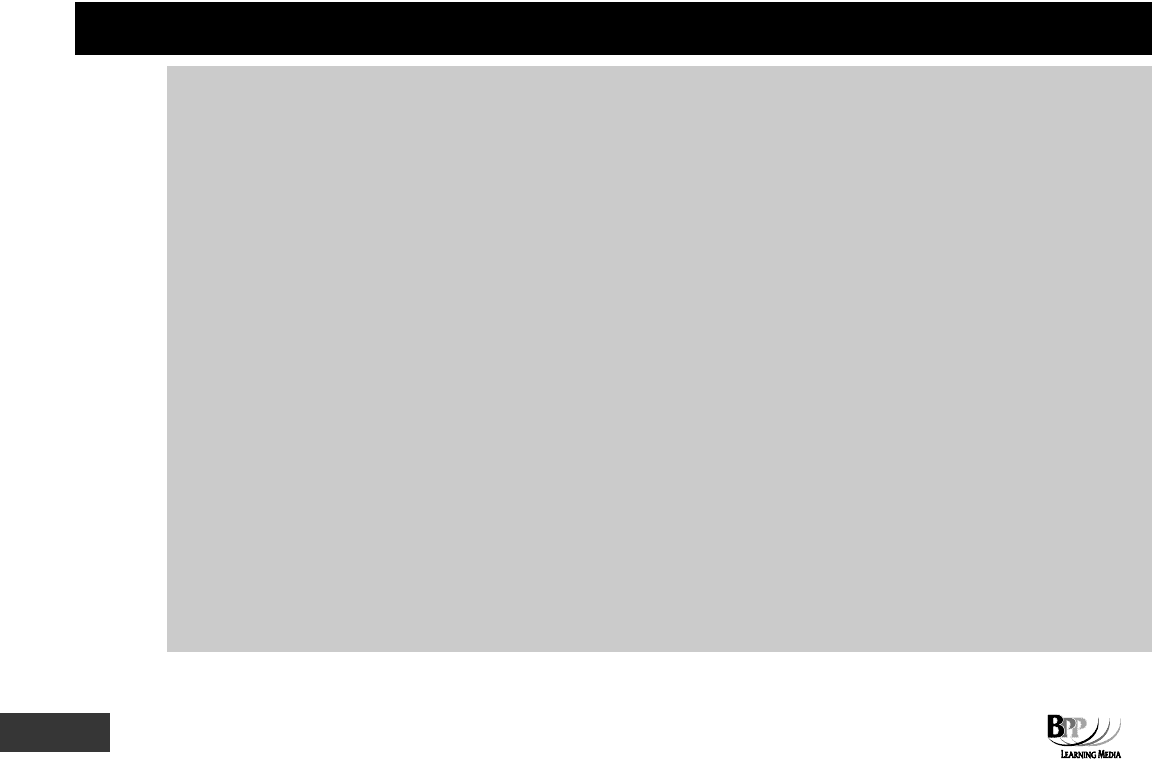
300 11: Forecasting ⏐ Part F Forecasting
(a) It assumes a linear relationship exists between the two variables (since linear regression analysis
produces an equation in the linear format) whereas a non-linear relationship might exist.
(b) It
assumes that the value of one variable, Y, can be predicted or estimated from the value of one
other variable, X
. In reality the value of Y might depend on several other variables, not just X.
(c) When it is used for forecasting,
it assumes that what has happened in the past will provide a
reliable guide to the future
.
(d) When calculating a line of best fit, there will be a range of values for X. In the example in Paragraph
6.2, Chapter 10, the line Y = 28 + 2.6X was predicted from data with output values ranging from X =
16 to X = 24. Depending on the degree of correlation between X and Y, we might safely use the
estimated line of best fit to predict values for Y in the future, provided that the value of X remains
within the range 16 to 24. We would be on less safe ground if we used the formula to predict a value
for Y when X = 10, or 30, or any other value outside the range 16 to 24, because we would have to
assume that the trend line applies outside the range of X values used to establish the line in the
first place
.
(e) As with any forecasting process,
the amount of data available is very important. Even if correlation
is high, if we have fewer than about ten pairs of values, we must regard any forecast as being
somewhat unreliable. (It is likely to provide more reliable forecasts than the scattergraph method,
however, since it uses all of the available data.)
(f)
The reliability of a forecast will depend on the reliability of the data collected to determine the
regression analysis equation
. If the data is not collected accurately or if data used is false, forecasts
are unlikely to be acceptable.
Chapter Roundup
• A time series is a series of figures or values recorded over time. Any pattern found in the data is then assumed to
continue into the future and an extrapolative forecast is produced.
• There are four components of a time series: trend, seasonal variations, cyclical variations and random
variations.
• The trend is the underlying long-term movement over time in the values of the data recorded.
• Seasonal variations are short-term fluctuations in recorded values, due to different circumstances which affect
results at different times of the year, on different days of the week or at different times of the day etc.
• Cyclical variations are medium-term changes in results caused by circumstances which repeat in cycles.
• One method of finding the trend is by the use of moving averages.
• When finding the moving average of an even number of results, a second moving average has to be calculated so
that trend values can relate to specific actual figures.
• Seasonal variations are the difference between actual and trend figures. An average of the seasonal variations
for each time period within the cycle must be determined and then adjusted so that the total of the seasonal
variations sums to zero. Seasonal variations can be estimated using the additive model (Y = T + S + R, with
seasonal variations = Y – T) or the multiplicative model (Y = T × S × R, with seasonal variations = Y/T).
• Forecasts can be made by extrapolating the trend and adjusting for seasonal variations.
• There are a number of factors which will affect the reliability of forecasts. Remember that all forecasts are subject
to error.