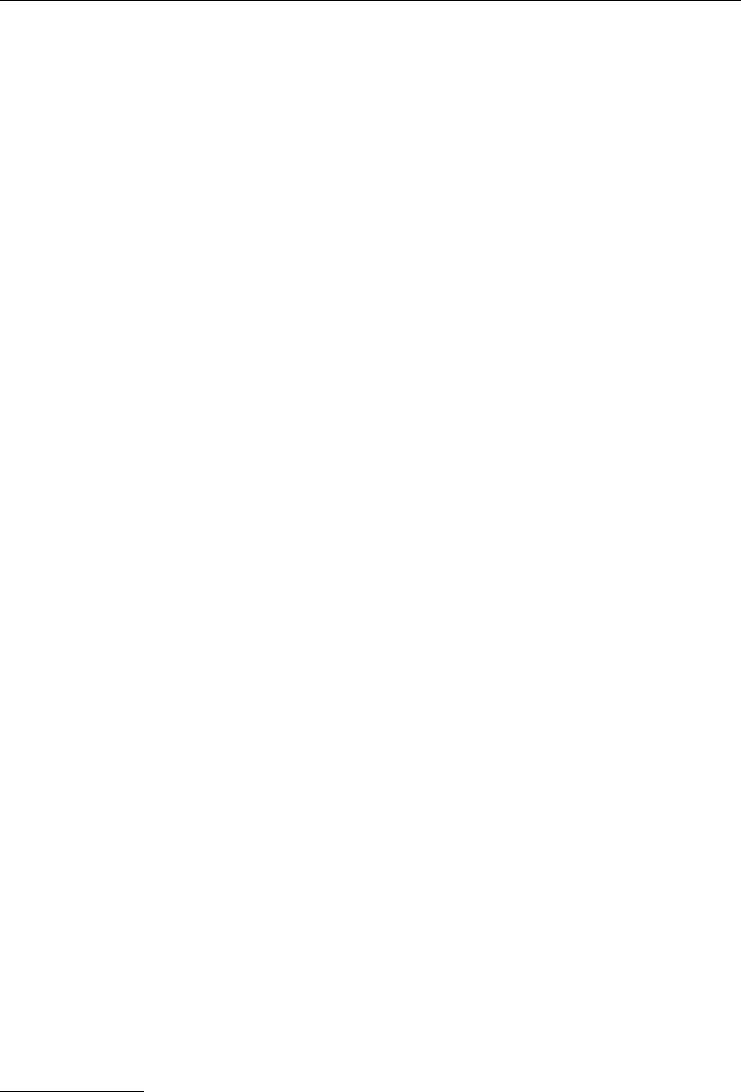
104 Investment Risk Management
r
Second – there is the goal of detecting consistent patterns of loss, and extrapolating from
the data to predict the likely level of future business losses.
The ultimate objective is to reduce their level of losses and increase the predictability of the
remaining losses. The downside risk of this project is an expensive business and an IT white
elephant that does not meet business expectations.
A large global bank can have an expensive loss database, both in terms of number and
value of loss items, plus the huge project costs of creating the database. They cannot afford
to get it wrong because to do so would be both costly and embarrassing. Backing out a failed
loss database project from all global branches would also be a high-profile noticeable loss
(compare: Reputational risk).
An operational loss database, driven by the desire for good management or by the regulators,
represents a large investment. An empowered band of financial specialists can reap real rewards
for the company, supported by IT systems staff to “drill-down” within the loss database. This
data-mining involves finding out lines of causality for:
r
who
r
when
r
how much was lost
r
how much could have been lost
r
why it all happened in the first place.
Loss databases will have to prove themselves against resilience-based approaches. These
data will be analysed time and time again under different data-mining angles. The real test will
be that of continual testing and review for cost-benefit analysis.
The loss database is a potentially good corporate risk management tool, but, it is likely to fail
where it attracts little support within the corporation. Loss data are input for risk management
decision making, and it needs a lot of massaging into acceptable reports before it can help
to formulate director-level actions. The initiative stands or falls on whether top management
supports and funds it.
The benefits are easier to predict than the costs. An advanced-certified operational risk-
managed bank will have lower Basel II regulatory capital charges because its risk management
processes are highly developed and evaluated as a lower overall risk. From previous regulatory
examples within credit risk, a bank could find its regulatory capital reserve falling by some
6%.
4
How much this will translate into similar savings for OpRisk is to be decided by the regulators
interpreting the Basel II guidelines.
5
Risk appetite becomes more directly linked to risk offer, but risk appetite is also covered
by Basel II regulatory capital. Risk support systems alert the danger of capital becoming
inadequate to cover expected losses.
The loss database business rationale may be a search for lower risk ratings and knowledge
data-mining, forced on them by the regulator. The compliance “Big stick” approach of the
regulator may be better at explaining the need for the database, instead of the more complex
business cost-benefit analysis.
Losing money has never been in the interests of a bank, nor of its clients. Yet banks and
investment funds continue to lose money without knowing where or why. There is some hope
4
Quantitative Impact Study 3, Basel Committee for Banking Supervision, May 2003.
5
Enterprise Risk Management, Professional Risk Management Association (PRMIA), London, 13 November 2002.