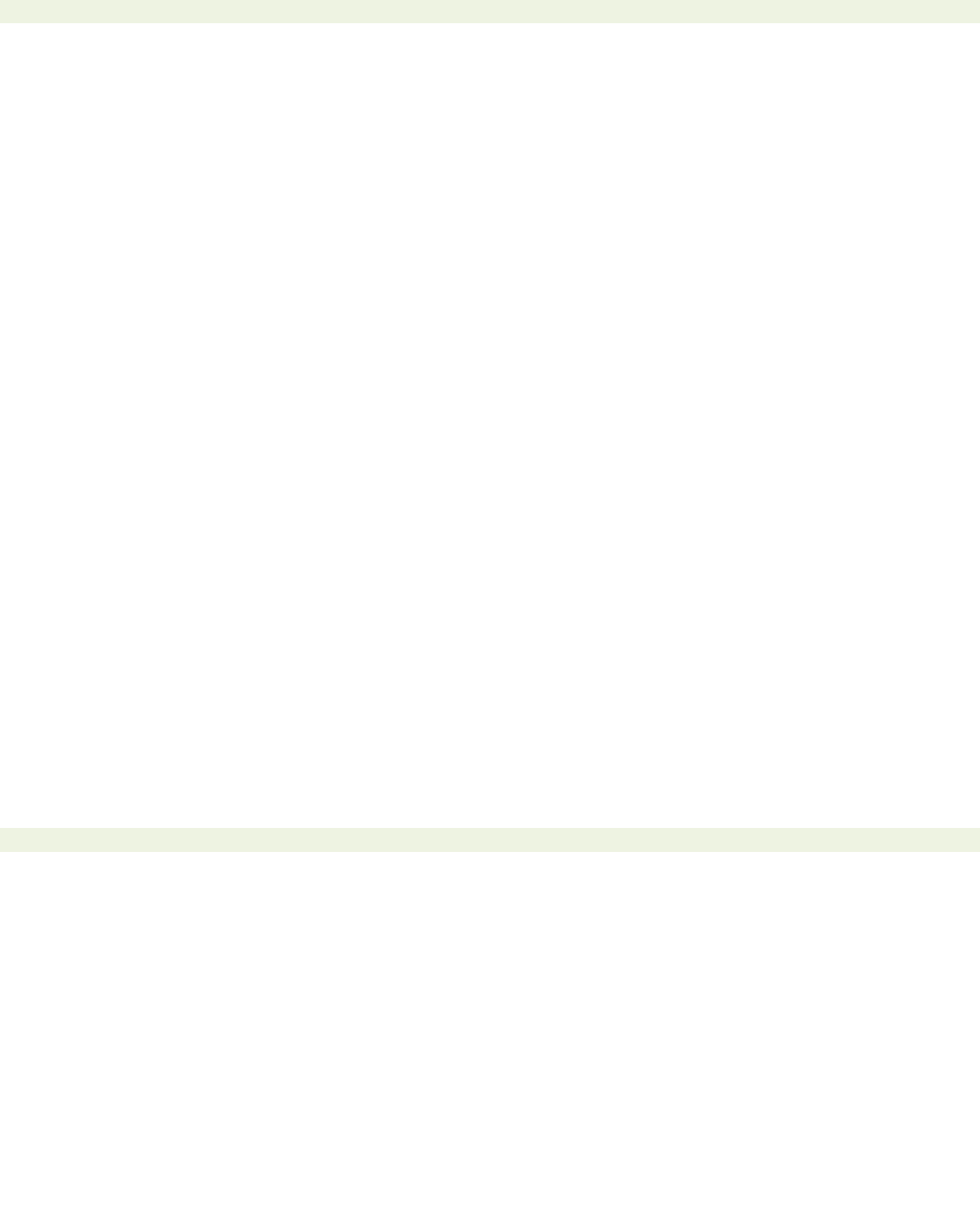
page_viii
file:///G|/%5EDoc%5E/_new_new/got/020349878X/files/page_viii.html[22/02/2009 23:50:52]
< previous page page_viii next page >
Page viii
suitably chosen, then
uN
is a good approximation to the unknown solution
u
. As we shall see,
M
will be
defined such that
uN
is just the partial sum of the first
N
terms of the infinite series traditionally
associated with the method of separation of variables.
The reader may recognize this view as identical to the setting of the finite element, collocation, and
spectral methods that have been developed for the numerical solution of differential equations. All these
methods differ in how the subspace
M
is chosen and in what sense the original problem is
approximated. These choices dictate how hard it is to compute the approximate solution
uN
and how
well it approximates the analytic solution
u
.
Given the almost universal applicability of numerical methods for the solution of partial differential
equations, the question arises whether separation of variables with its severe restrictions on the type of
equation and the geometry of the problem is still a viable tool and deserves further exposition. The
existence of this text reflects our view that the method of separation of variables still belongs to the
core of applied mathematics. There are a number of reasons.
Closed form (approximate) solutions show structure and exhibit explicitly the influence of the problem
parameters on the solution. We think, for example, of the decomposition of wave motion into standing
waves, of the relationship between driving frequency and resonance in sound waves, of the influence of
diffusivity on the rate of decay of temperature in a heated bar, or of the generation of equipotential and
stream lines for potential flow. Such structure and insight are not readily obtained from purely numerical
solutions of the underlying differential equation. Moreover, optimization, control, and inverse problems
tend to be easier to solve when an analytic representation of the (approximate) solution is available. In
addition, the method is not as limited in its applicability as one might infer from more elementary texts
on separation of variables. Approximate solutions are readily computable for problems with time-
dependent data, for diffusion with convection and wave motion with dissipation, problems seldom seen
in introductory textbooks. Even domain restrictions can sometimes be overcome with embedding and
domain decomposition techniques. Finally, there is the class of singularly perturbed and of higher
dimensional problems where numerical methods are not easily applied while separation of variables still
yields an analytic approximate solution.
Our rationale for offering a new exposition of separation of variables is then twofold. First, although
quite common in more advanced treatments (such as [15]), interpreting the separation of variables
solution as an eigenfunction expansion is a point of view rarely taken when introducing the method to
students. Usually the formalism is based on a product solution for the partial differential equation, and
this limits the applicability of the method to homogeneous partial differential equations. When source
terms do appear, then a reformulation of problems for the heat and wave equation with the help of
Duhamel’s superposition principle and an approximation of the source term in the potential equation
with the help of an eigenfunction approximation become necessary. In an exposition based from the
beginning on an eigenfunction expansion, the presence of source terms in the differential equation is
only a technical, but not a conceptual
< previous page page_viii next page >