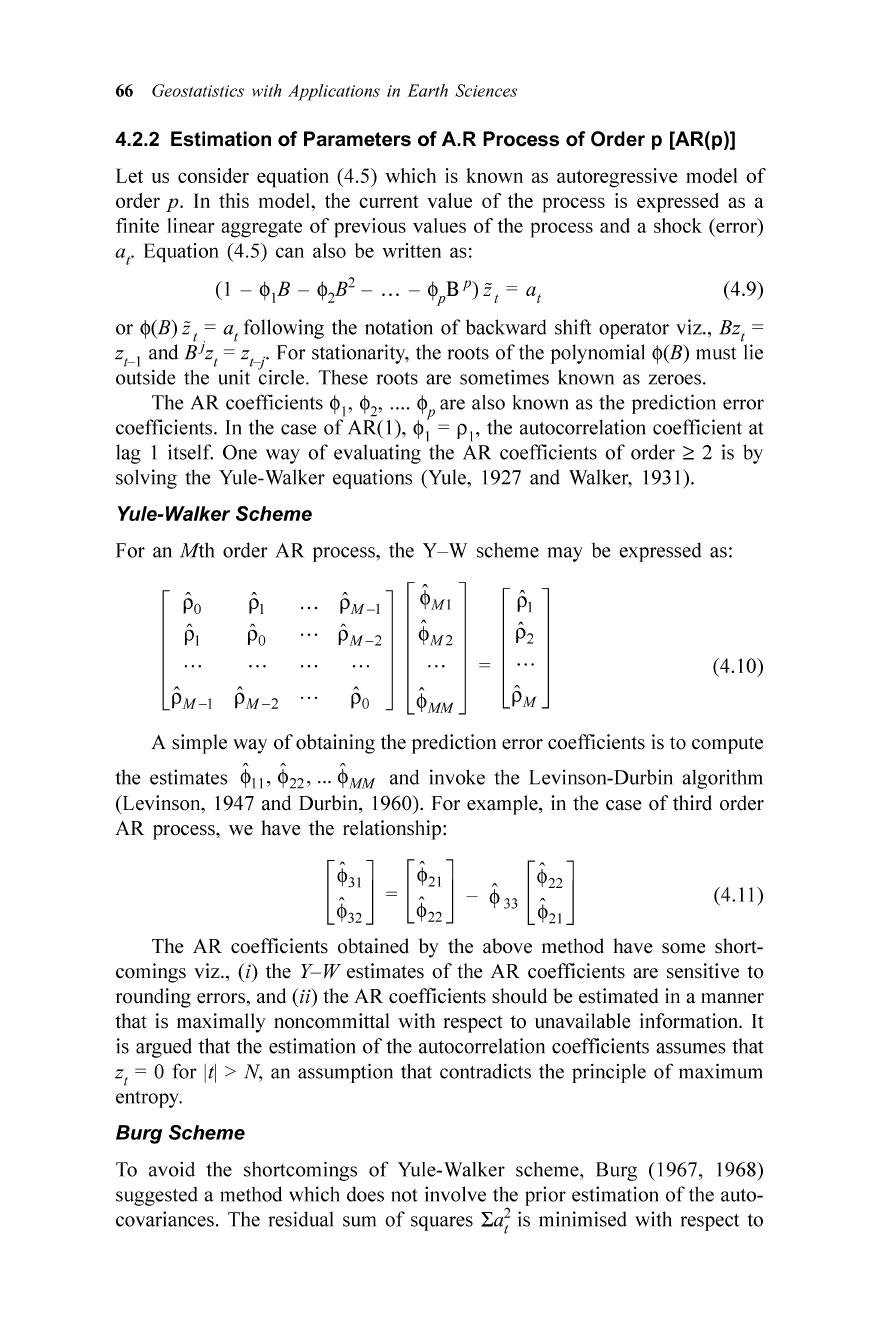
(4.11)
66 Geostatistics with Applications in Earth Sciences
4.2.2 Estimation of Parameters of A.R Process of Order p [AR(p)]
Let us consider equation (4.5) which is known as autoregressive model
of
order p . In this mode l, the current value
of
the process is expressed as a
finite linear aggregate
of
previous values
of
the process and a shock (error)
at" Equation (4.5) can also be written as:
( I -
<p,B
-
<P2B2
- •.• -
<ppBP)Zt
= at (4.9)
or
<p(B)
Zt = at following the notation
of
backward shift operator viz., BZ
t
=
Z
t_1
and B jzt = Zt
_j"
For stationarity, the roots
of
the polynomial
<p(B)
must lie
outside the unit circle. These roots are sometimes known as zeroes.
The AR coefficients
<PI'
<P
2'
....
<P
p
are also known as the prediction error
coefficients. In the case
of
AR(I),
<PI
= PI' the autocorrelation coefficient at
lag I itself. One way
of
eva luating the AR coefficients
of
order
~
2 is by
solving the Yule-Walker equations (Yule, 1927 and Walker, 193 1).
Yule-Walker Scheme
For an Mth order AR process, the Y
-W
scheme may be expressed as:
Po
P,
...
~M-I
]
<PM
1
~
[
f
~]
PI
Po
.
..
PM-2
<PM2
(4.10)
PM
-'
PM
-2
Po
<PMM
A simple way
of
obtaining the prediction error coefficients is to compute
A A A
the estimates
<PI
"
<P22
' ...
<PMM
and invoke the Levinson-Durbin algorithm
(Levinson, 1947 and Durbin, 1960). For example, in the case
of
third order
AR process, we have the relationship:
[
~::
]
~
[
~
:]
-
~
33 [t:
:]
The AR coefficients obtained by the above method have some short-
comings viz.,
(i) the Y
-W
estimates
of
the AR coefficients are sensitive to
rounding errors, and
(ii)
the AR coefficients should be estimated in a manner
that is maximally noncommittal with respect to unavailable information. It
is argued that the estimation
of
the autocorrelation coefficients assumes that
Zt = 0 for ItI > N, an assumption that contradicts the principle
of
maximum
entropy.
Burg Scheme
To avoid the shortcomings
of
Yule-Walker scheme, Burg (1967 , 1968)
suggested a method which does not invo lve the prior estimation
of
the auto-
covariances. The residual sum
of
squares
La;
is minimised with respect to