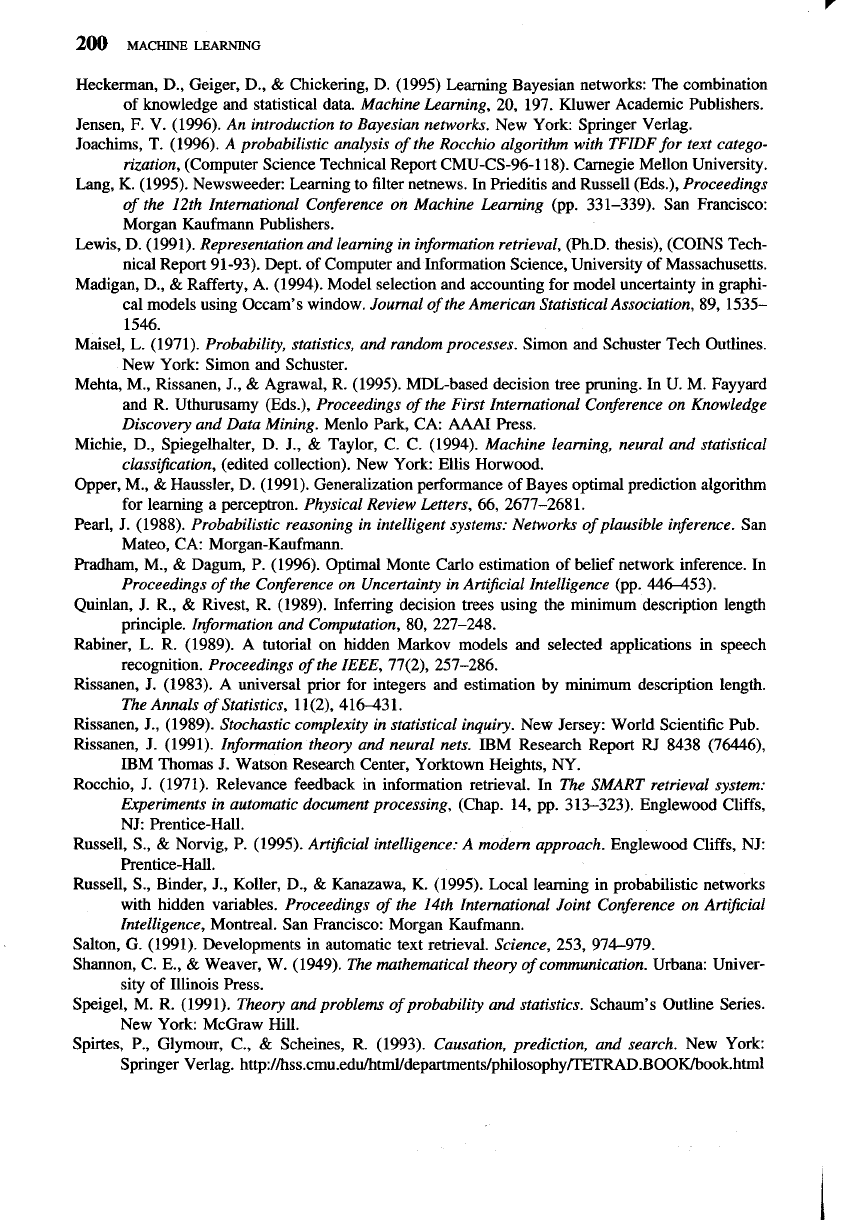
200
MACHINE
LEARNING
Heckerman,
D.,
Geiger, D.,
&
Chickering,
D.
(1995) Learning Bayesian networks: The combination
of knowledge and statistical data.
Machine Learning,
20, 197. Kluwer Academic Publishers.
Jensen, F. V. (1996).
An introduction to Bayesian networks.
New York: Springer Verlag.
Joachims, T. (1996).
A probabilistic analysis of the Rocchio algorithm with TFIDF for text catego-
rization,
(Computer Science Technical Report CMU-CS-96-118). Carnegie Mellon University.
Lang, K. (1995). Newsweeder: Learning to filter netnews. In Prieditis and Russell (Eds.),
Proceedings
of the 12th International Conference on Machine Learning
(pp. 331-339). San Francisco:
Morgan Kaufmann Publishers.
Lewis, D. (1991).
Representation and learning in information retrieval,
(Ph.D. thesis), (COINS Tech-
nical Report 91-93). Dept. of Computer and Information Science, University of Massachusetts.
Madigan,
D.,
&
Rafferty, A. (1994). ~odel selection and accounting for model uncertainty in graphi-
cal models using Occam's window.
Journal of the American Statistical Association,
89, 1535-
1546.
Maisel,
L.
(1971).
Probability, statistics, and random processes.
Simon and Schuster Tech Outlines.
New York: Simon and Schuster.
Mehta, M., Rissanen, J.,
&
Agrawal, R. (1995). MDL-based decision tree pruning. In
U.
M. Fayyard
and R. Uthurusamy (Eds.),
Proceedings of the First International Conference on Knowledge
Discovery and Data Mining.
Menlo Park, CA: AAAI Press.
Michie,
D.,
Spiegelhalter,
D.
J.,
&
Taylor, C. C. (1994).
Machine learning, neural and statistical
classification,
(edited collection). New York: Ellis Horwood.
Opper, M.,
&
Haussler,
D.
(1991). Generalization performance of Bayes optimal prediction algorithm
for learning a perceptron.
Physical Review Letters,
66, 2677-2681.
Pearl, J. (1988).
Probabilistic reasoning in intelligent systems: Networks of plausible inference.
San
Mateo, CA: Morgan-Kaufmann.
Pradham, M.,
&
Dagum, P. (1996). Optimal Monte Carlo estimation of belief network inference. In
Proceedings of the Conference on Uncertainty in Artijicial Intelligence
(pp. 44-53).
Quinlan, J. R.,
&
Rivest, R. (1989). Inferring decision trees using the minimum description length
principle.
Information and Computation,
80, 227-248.
Rabiner,
L.
R. (1989).
A
tutorial on hidden Markov models and selected applications in speech
recognition.
Proceedings of the IEEE,
77(2), 257-286.
Rissanen, J. (1983). A universal prior for integers and estimation by minimum description length.
The Annals of Statistics,
11(2), 41-31.
Rissanen, J., (1989).
Stochastic complexity in statistical inquiry.
New Jersey: World Scientific Pub.
Rissanen, J. (1991).
Information theory and neural nets.
IBM Research Report
RJ
8438 (76446),
IBM Thomas J. Watson Research Center, Yorktown Heights, NY.
Rocchio, J. (1971). Relevance feedback in information retrieval. In
The SMART retrieval system:
Experiments in automatic document processing,
(Chap. 14, pp. 313-323). Englewood Cliffs,
NJ: Prentice-Hall.
Russell, S.,
&
Nomig, P. (1995).
Artificial intelligence:
A
modem approach.
Englewood Cliffs, NJ:
Prentice-Hall.
Russell, S., Binder, J., Koller,
D.,
&
Kanazawa, K. (1995). Local learning in probabilistic networks
with hidden variables.
Proceedings of the 14th International Joint Conference on Artificial
Intelligence,
Montreal. San Francisco: Morgan Kaufmann.
Salton, G. (1991). Developments in automatic text retrieval.
Science,
253, 974-979.
Shannon, C. E.,
&
Weaver,
W.
(1949).
The mathematical theory of communication.
Urbana: Univer-
sity of Illinois Press.
Speigel, M. R. (1991).
Theory and problems of probability and statistics.
Schaum's Outline Series.
New York: McGraw Hill.
Spirtes, P., Glymour, C.,
&
Scheines, R. (1993).
Causation, prediction, and search.
New York:
Springer Verlag.
http://hss.cmu.edu/htmUdepartments/philosophy~~D.BOO~ook.h~