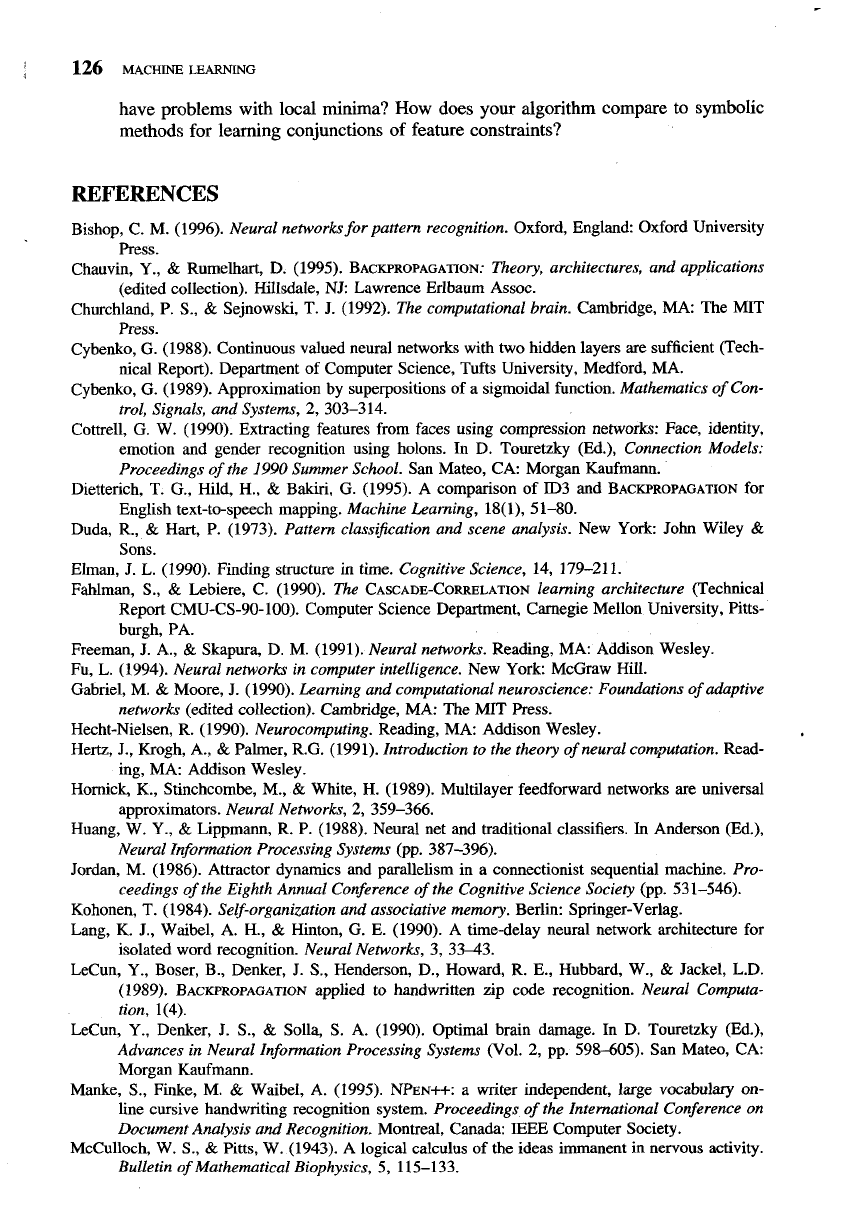
have problems with local minima? How does your algorithm compare to symbolic
methods for learning conjunctions of feature constraints?
REFERENCES
Bishop, C. M. (1996). Neural networks for pattern recognition. Oxford, England: Oxford University
Press.
Chauvin,
Y.,
&
Rumelhart, D. (1995). BACKPROPAGATION: Theory, architectures, and applications
(edited collection). Hillsdale,
NJ:
Lawrence Erlbaum Assoc.
Churchland, P. S.,
&
Sejnowski, T. J. (1992). The computational brain. Cambridge, MA: The MIT
Press.
Cyhenko, G. (1988). Continuous valued neural networks with two hidden layers are sufficient (Tech-
nical Report). Department of Computer Science, Tufts University, Medford, MA.
Cybenko, G. (1989). Approximation by superpositions of a sigmoidal function.
Mathematics of Con-
trol, Signals, and Systems,
2, 303-3 14.
Cottrell, G. W. (1990). Extracting features from faces using compression networks: Face, identity,
emotion and gender recognition using holons. In D. Touretzky
(Ed.),
Connection Models:
Proceedings of the
1990
Summer School. San Mateo, CA: Morgan Kaufmann.
Dietterich, T. G., Hild, H.,
&
Bakiri, G. (1995). A comparison of ID3 and BACKPROPAGATION for
English text-to-speech mapping.
Machine Learning, 18(1), 51-80.
Duda, R.,
&
Hart, P. (1973). Pattern class@cation and scene analysis. New York: John Wiley
&
Sons.
Elman, J.
L.
(1990). Finding structure in time. Cognitive Science, 14, 179-21 1.
Fahlman, S.,
&
Lebiere, C. (1990). The CASCADE-CORRELATION learning architecture (Technical
Report CMU-CS-90-100). Computer Science Department, Carnegie Mellon University, Pitts-
burgh, PA.
Freeman, J.
A.,
&
Skapura,
D.
M. (1991). Neural networks. Reading, MA: Addison Wesley.
Fu, L. (1994).
Neural networks in computer intelligence. New York: McGraw Hill.
Gabriel, M.
&
Moore, J. (1990). Learning and computational neuroscience: Foundations of adaptive
networks
(edited collection). Cambridge, MA: The MIT Press.
Hecht-Nielsen, R. (1990).
Neurocomputing. Reading, MA: Addison Wesley.
Hertz, J., Krogh, A.,
&
Palmer, R.G. (1991). Introduction to the theory of neural computation. Read-
ing, MA: Addison Wesley.
Homick, K., Stinchcombe, M.,
&
White, H. (1989). Multilayer feedforward networks are universal
approximators.
Neural Networks, 2, 359-366.
Huang, W. Y.,
&
Lippmann, R. P. (1988). Neural net and traditional classifiers.
In
Anderson (Ed.),
Neural Information Processing Systems (pp. 387-396).
Jordan, M. (1986). Attractor dynamics and parallelism in a connectionist sequential machine.
Pro-
ceedings of the Eighth Annual Conference of the Cognitive Science Society
(pp. 531-546).
Kohonen, T. (1984).
Self-organization and associative memory. Berlin: Springer-Verlag.
Lang, K.
J.,
Waibel, A. H.,
&
Hinton, G. E. (1990). A time-delay neural network architecture for
isolated word recognition.
Neural Networks,
3,
3343.
LeCun,
Y.,
Boser, B., Denker, J. S., Henderson, D., Howard, R. E., Hubbard, W.,
&
Jackel,
L.D.
(1989). BACKPROPAGATION applied to handwritten zip code recognition. Neural Computa-
tion,
l(4).
LeCun,
Y.,
Denker,
J.
S.,
&
Solla, S. A. (1990). Optimal brain damage. In D. Touretzky (Ed.),
Advances in Neural Information Processing Systems (Vol. 2, pp. 598405). San Mateo, CA:
Morgan Kaufmann.
Manke, S., Finke, M.
&
Waibel, A. (1995). NPEN++: a writer independent, large vocabulary on-
line cursive handwriting recognition system.
Proceedings of the International Conference on
Document Analysis
and
Recognition. Montreal, Canada: IEEE Computer Society.
McCulloch,
W.
S.,
&
Pitts, W. (1943). A logical calculus of
the
ideas immanent in nervous activity.
Bulletin
of
Mathematical Biophysics,
5,
115-133.