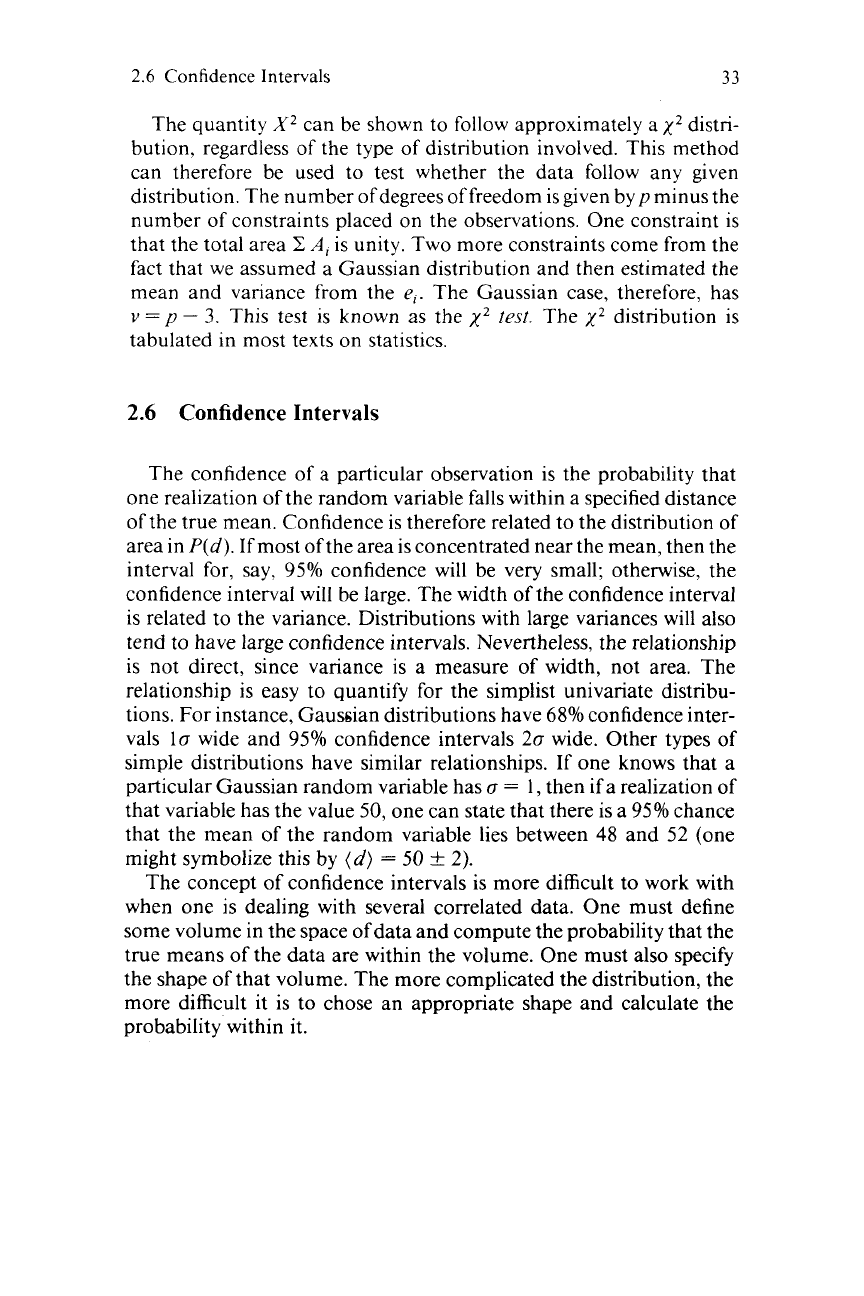
2.6
Confidence Intervals
33
The quantity
X2
can be shown to follow approximately a
x2
distri-
bution, regardless of the type of distribution involved. This method
can therefore be used to test whether the data follow any given
distribution. The number ofdegrees
of
freedom is given byp minus the
number of constraints placed on the observations. One constraint is
that the total area
C
A,
is unity. Two more constraints come from the
fact that we assumed a Gaussian distribution and then estimated the
mean and variance from the
el.
The Gaussian case, therefore, has
v
=
p
-
3.
This test is known as the
x2
test.
The
x2
distribution is
tabulated in most texts on statistics.
2.6
Confidence
Intervals
The confidence of a particular observation is the probability that
one realization of the random variable falls within a specified distance
of the true mean. Confidence is therefore related to the distribution of
area in
P(d).
If
most ofthe area is concentrated near the mean, then the
interval for, say,
95%
confidence will be very small; otherwise, the
confidence interval will be large. The width of the confidence interval
is related to the variance. Distributions with large variances will also
tend to have large confidence intervals. Nevertheless, the relationship
is not direct, since variance is a measure
of
width, not area. The
relationship is easy to quantify for the simplist univariate distribu-
tions.
For
instance, Gaussian distributions have
68%
confidence inter-
vals
la
wide and
95%
confidence intervals
20
wide. Other types of
simple distributions have similar relationships.
If
one knows that a
particular Gaussian random variable has
a
=
1,
then if a realization of
that variable has the value
50,
one can state that there is a
95%
chance
that the mean of the random variable lies between
48
and
52
(one
might symbolize this
by
(d)
=
50
f
2).
The concept of confidence intervals is more difficult to work with
when one is dealing with several correlated data. One must define
some volume in the space of data and compute the probability that the
true means of the data are within the volume. One must also specify
the shape of that volume. The more complicated the distribution, the
more difficult it is to chose an appropriate shape and calculate the
probability within it.