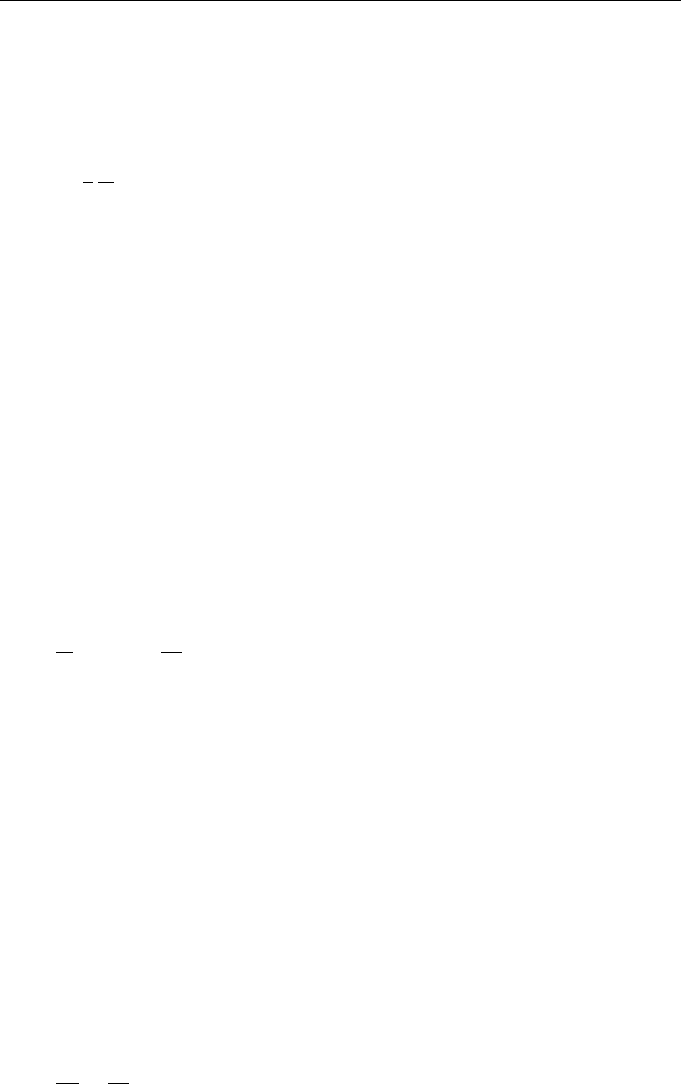
158 Lectures on Dynamics of Stochastic Systems
and all multi-time statistics of random functions v(t) and r(t) will be described in
terms of statistical characteristics of process f (t). In particular, the one-time probabi-
lity density of particle velocity v(t) is the Gaussian probability density with variance
v
i
(t)v
j
(t)
= B
ij
(0), and the spatial diffusion coefficient is
D =
1
2
d
dt
D
r
2
(t)
E
=
∞
Z
0
dτ B
ii
(τ ) = τ
0
B
ii
(0).
As we have seen earlier, in the case of process f (t) such that it can be correctly
described in the delta-correlated approximation (i.e., if λτ
0
1), the approximate
equality (6.53) appears inappropriate to determine statistical characteristics of process
v(t). Nevertheless, Eq. (6.52) with the same diffusion tensor remains as before valid
for the one-time statistical characteristics of process r(t), which follows from the fact
that Eq. (6.48) is valid for any parameter λ and arbitrary probability density of random
process f(t).
Above, we considered several types of stochastic ordinary differential equations
that allow obtaining closed statistical description in the general form. It is clear that
similar situations can appear in dynamic systems formulated in terms of partial diffe-
rential equations.
6.2.2 Partial Differential Equations
First of all, we note that the first-order partial differential equation
∂
∂t
+ z(t)g(t)
∂
∂x
F(x)
ρ(x, t) = 0, (6.54)
is equivalent to the system of ordinary differential equations (6.10), page 144 and, con-
sequently, also allows the complete statistical description for arbitrary given random
process z(t).
Solution ρ(r, t) to Eq. (6.54) is a functional of random process z(t) of the form
ρ(x, t) = ρ[x, t; z(τ)] = ρ(x, T[t; z(τ )]),
with functional
T[t; z(τ )] =
t
Z
0
dτ z(τ )g(τ ), (6.55)
so that Eq. (6.54) can be rewritten in the form
∂
∂T
+
∂
∂x
F(x)
ρ(x, T) = 0.