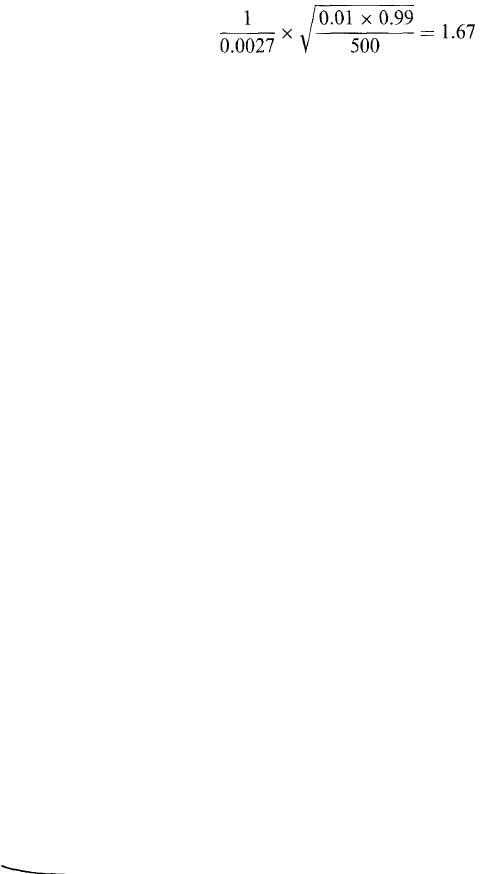
VaR: Historical Simulation Approach 221
If the estimate of the 0.01 quantile using historical simulation is $25 million,
a 95% confidence interval is from 25 — 1.96 x 1.67 to 25 + 1.96 x 1.67, that
is, from $21.7 million to $28.3 million.
As Example 9.1 illustrates, the standard error of a VaR estimated using
historical simulation tends to be quite high. It increases as the VaR
confidence level is increased. For example, if in Example 9.1 the VaR
confidence level had been 95% instead of 99%, the standard error would
be $0.95 million instead of $1.67 million. The standard error declines as
the sample size is increased—but only as the square root of the sample
size. If we quadrupled the sample size in Example 9.1 from 500 to 2,000
(i.e., from approximately two to approximately eight years of data), the
standard error halves from $1.67 million to about $0.83 million.
Additionally, we should bear in mind that historical simulation assumes
that the joint distribution of daily changes in market variables is stationary
through time. This is unlikely to be exactly true and creates additional
uncertainty about the value of VaR.
9.3 EXTENSIONS
In this section we cover a number of extensions of the basic historical
simulation methodology that we discussed in Section 9.1.
Weighting of Observations
The basic historical simulation approach assumes that each day in the past
is given equal weight. More formally, if we have observations for n day-to-
day changes, each of them is given a weighting of \/n. Boudoukh et al.
suggest that more recent observations should be given more weight
because they are more reflective of current volatilities and current macro-
economic variables.
4
The natural weighting scheme to use is one where
4
See J. Boudoukh, M. Richardson, and R. Whitelaw, "The Best of Both Worlds: A
Hybrid Approach to Calculating Value at Risk," Risk, 11 (May 1998), 64-67.
standard distribution. Suppose that the approximate empirical distribution is
normal with mean zero and standard deviation $10 million. Using Excel, the
0.01 quantile is NORMINV(0.01,0, 10), or 23.26. The value of f(x) is
NORMDIST(23.26, 0, 10, FALSE), or 0.0027. The standard error of the
estimate that is made is