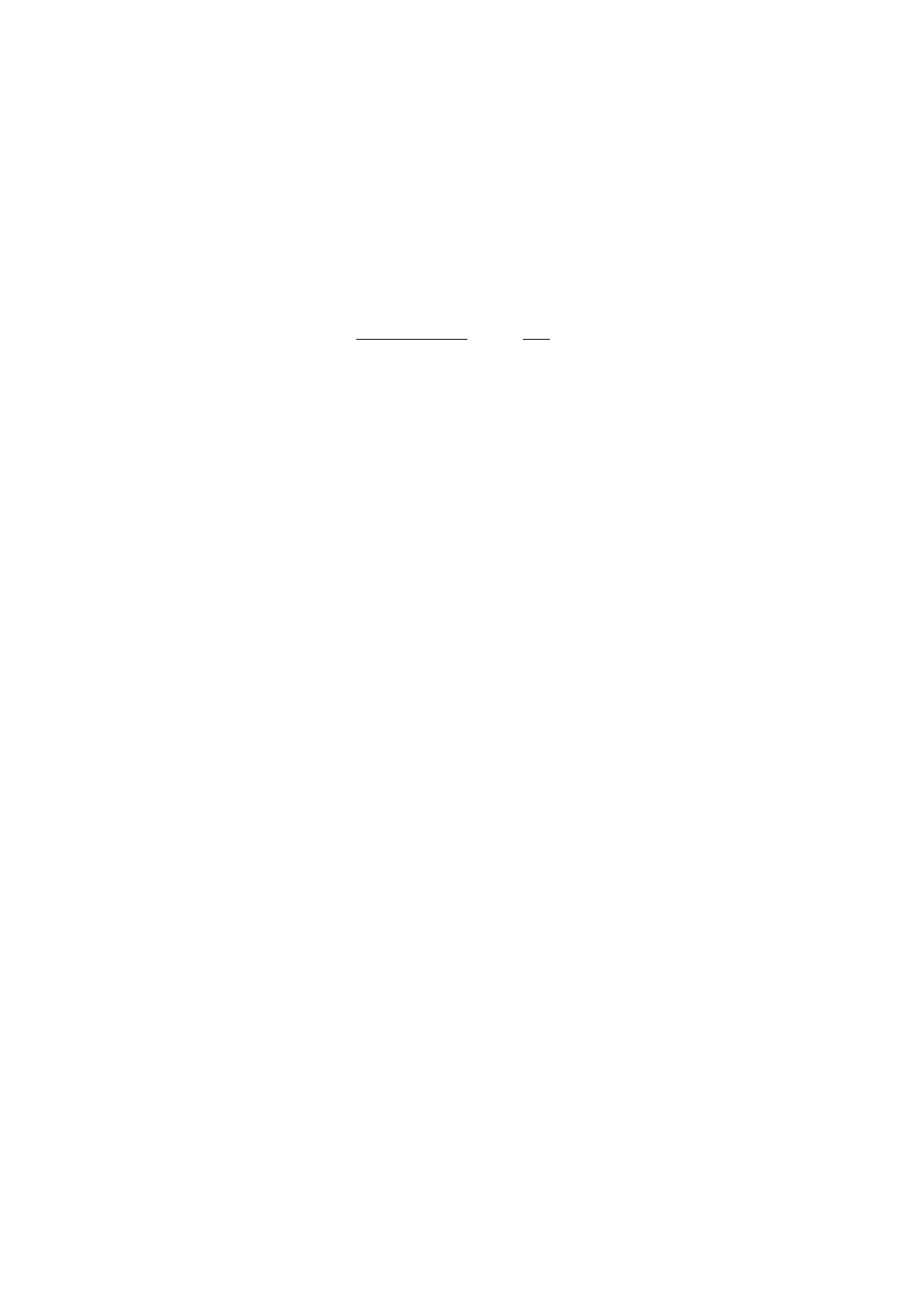
STOCHASTIC REGRESSORS AND MEASUREMENT ERRORS
3
Consistency
We know that, in general, plim (A/B) is equal to plim(A)/plim(B), where A and B are any two
stochastic quantities, provided that both plim(A) and plim(B) exist and that plim(B) is nonzero (see the
Review; "plim" simply means "the limiting value as the sample size becomes large"). We also know
that sample expressions tend to their population counterparts as the sample size becomes large, so plim
Cov(X, u) is the population covariance of X and u and plim Var(X) is
2
X
σ
, the population variance of
X. If X and u are independent, the population covariance of X and u is 0 and hence
2
2
222
0
)(Var plim
),(Cov plim
plim
σ
=+=+=
X
X
uX
b (9.5)
9.2 The Consequences of Measurement Errors
It frequently happens in economics that, when you are investigating a relationship, the variables
involved have been measured defectively. For example, surveys often contain errors caused by the
person being interviewed not remembering properly or not understanding the question correctly.
However, misreporting is not the only source of inaccuracy. It sometimes happens that you have
defined a variable in your model in a certain way, but the available data correspond to a slightly
different definition. Friedman's critique of the conventional consumption function, discussed in
Section 9.3, is a famous case of this.
Measurement Errors in the Explanatory Variable(s)
Let us suppose that a variable Y depends on a variable Z according to the relationship
Y
i
=
β
1
+
β
2
Z
i
+ v
i
(9.6)
where v is a disturbance term with mean 0 and variance
2
v
σ
, distributed independently of Z. We shall
suppose that Z cannot be measured absolutely accurately, and we shall use X to denote its measured
value. In observation i, X
i
is equal to the true value, Z
i
, plus the measurement error, w
i
:
X
i
= Z
i
+ w
i
(9.7)
We shall suppose that w has mean 0 and variance
2
w
σ
, that Z has population variance
2
Z
σ
, and that w is
distributed independently of Z and v.
Substituting from (9.7) into (9.6), we obtain
Y
i
=
β
1
+
β
2
(
X
i
– w
i
) + v
i
=
β
1
+
β
2
X
i
+ v
i
–
β
2
w
i
(9.8)