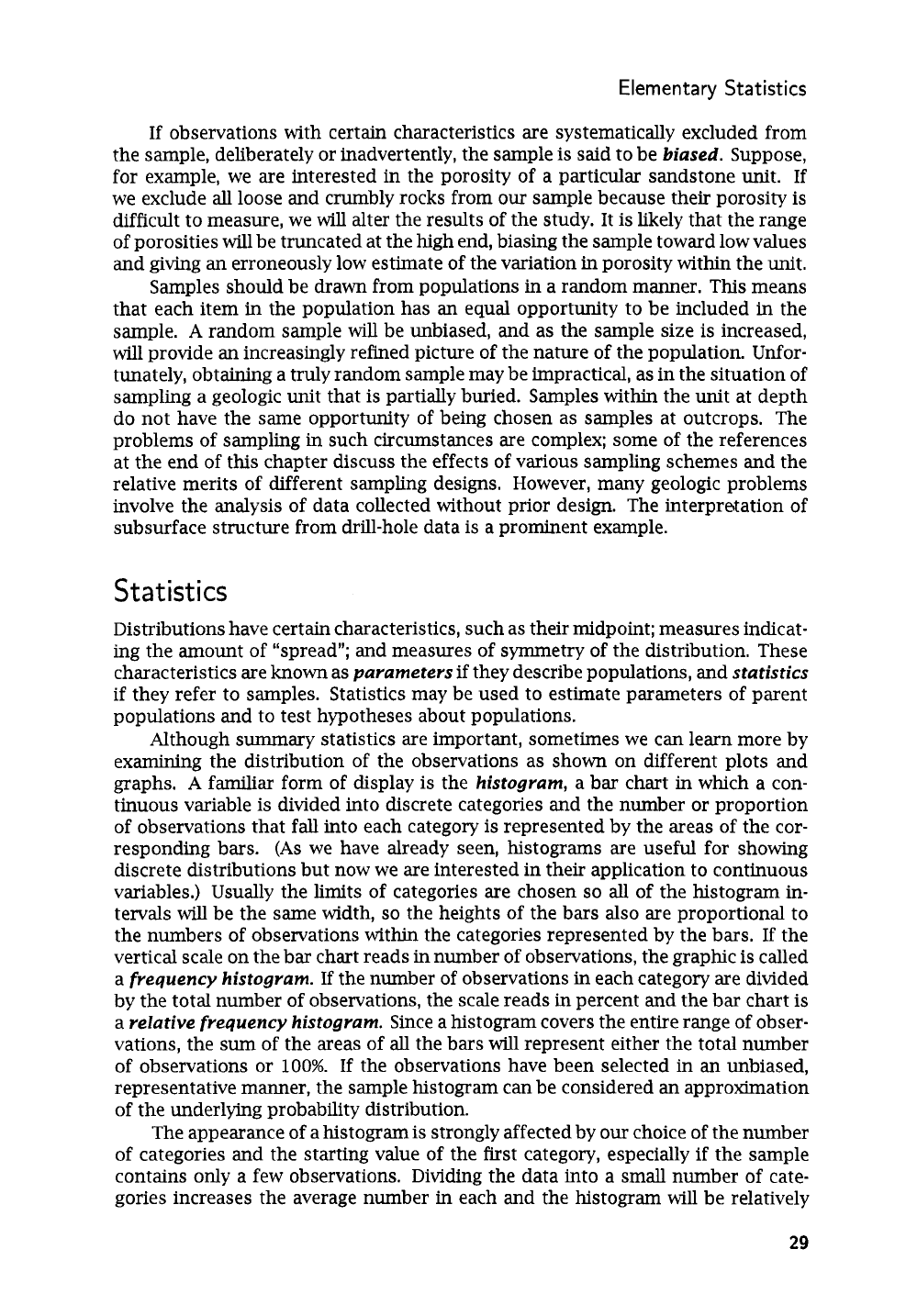
Elementary Statistics
If
observations with certain characteristics are systematically excluded from
the sample, deliberately or inadvertently, the sample
is
said to be
biased.
Suppose,
for example, we are interested in the porosity of a particular sandstone unit.
If
we exclude
all
loose and crumbly rocks from our sample because their porosity is
difficult to measure, we will alter the results of the study. It
is
likely that the range
of porosities
will
be truncated at the high end, biasing the sample toward low values
and giving
an
erroneously low estimate of the variation in porosity within the unit.
Samples should be drawn from populations in a random manner. This means
that each item
in
the population has
an
equal opportunity to be included
in
the
sample.
A
random sample
will
be unbiased, and as the sample size
is
increased,
will provide an increasingly refined picture
of
the nature of the population. Unfor-
tunately, obtaining a truly random sample may be impractical, as in the situation of
sampling a geologic unit that is partially buried. Samples within the unit at depth
do not have the same opportunity of being chosen as samples at outcrops. The
problems of sampling
in
such circumstances are complex; some of the references
at the end of this chapter discuss the effects of various sampling schemes and the
relative merits of different sampling designs. However, many geologic problems
involve the analysis of data collected without prior design. The interpretation of
subsurface structure from drill-hole data
is
a prominent example.
Statistics
Distributions have certain characteristics, such as their midpoint; measures indicat-
ing the amount of "spread"; and measures of symmetry of the distribution. These
characteristics
are
known
as
parameters
if they describe populations, and
statistics
if
they refer to samples. Statistics may be used to estimate parameters of parent
populations and to test hypotheses about populations.
Although summary statistics are important, sometimes we can learn more by
examining the distribution of the observations as shown on different plots and
graphs.
A
familiar form of display
is
the
histogram,
a bar chart in which a con-
tinuous variable
is
divided into discrete categories and the number or proportion
of observations that fall into each category is represented by the areas of the cor-
responding
bars.
(As
we have already seen, histograms are useful for showing
discrete distributions but now we are interested in their application to continuous
variables.) Usually the limits of categories are chosen
so
all of the histogram
in-
tervals
will
be the same width,
so
the heights of the bars also are proportional to
the numbers of observations within the categories represented by the bars.
If
the
vertical scale on the bar chart reads in number of observations, the graphic
is
called
a
frequency histogram.
If
the number of observations
in
each category are divided
by the total number of observations, the scale reads
in
percent and the bar chart is
a
relative frequency histogram.
Since a histogram covers the entire range of obser-
vations, the sum of the areas of
all
the bars
will
represent either the total number
of observations or
100%.
If
the observations have been selected in
an
unbiased,
representative manner, the sample histogram can be considered an approximation
of the underlying probability distribution.
The appearance of a histogram
is
strongly affected by our choice of the number
of categories and the starting value of the first category, especially
if
the sample
contains
only
a few observations. Dividing the data into a small number of cate-
gories increases the average number in each and the histogram
will
be relatively
29