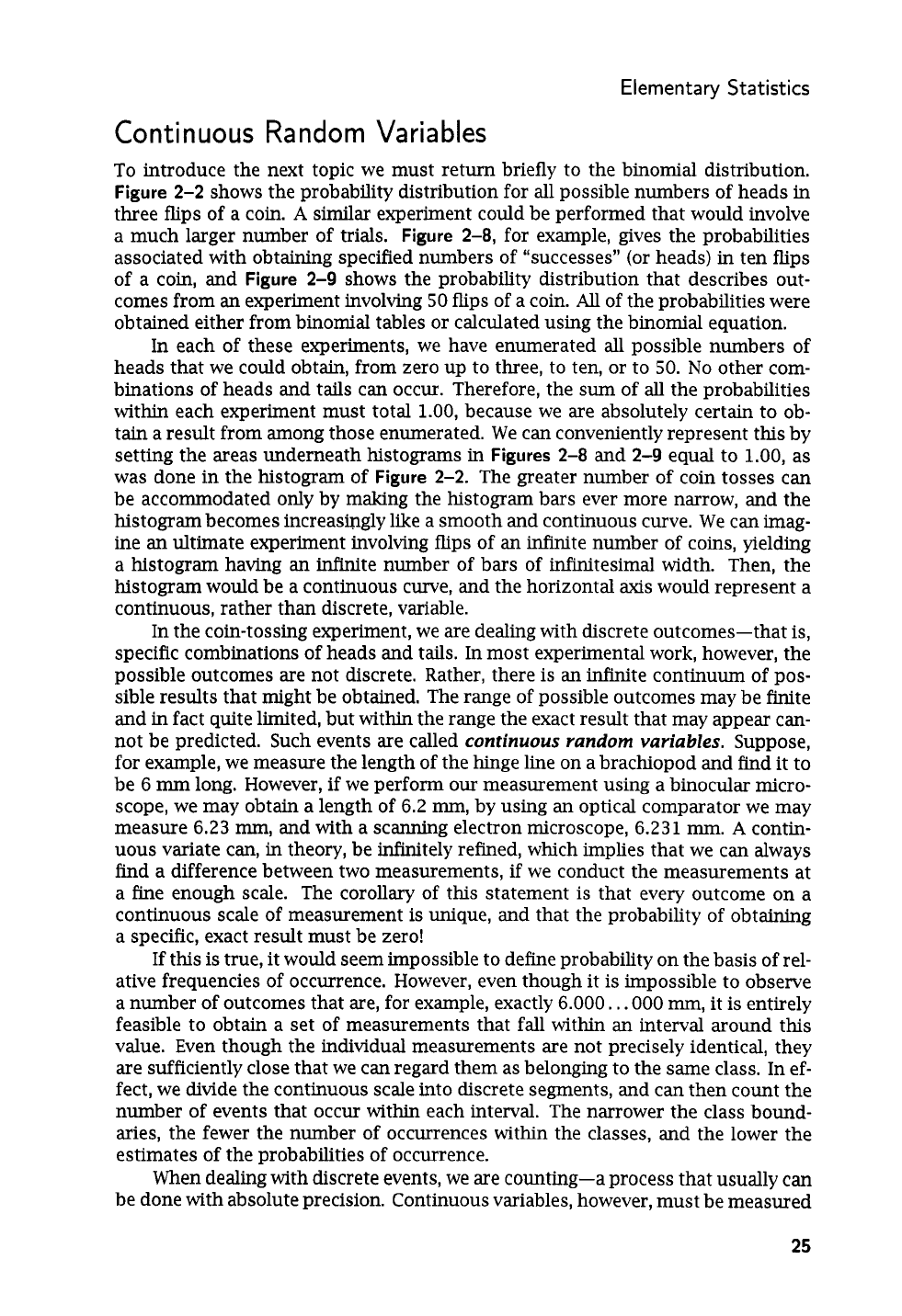
El
em
en
tary Statistics
Continuous
Random
Variables
To introduce the next topic we must return briefly to the binomial distribution.
Figure
2-2
shows the probability distribution for
all
possible numbers of heads in
three flips of a coin.
A
similar experiment could be performed that would involve
a much larger number of trials.
Figure
2-8,
for example, gives the probabilities
associated with obtaining specified numbers of “successes” (or heads) in ten flips
of a coin, and
Figure
2-9
shows the probability distribution that describes out-
comes from
an
experiment involving
50
flips of a coin.
All
of the probabilities were
obtained either from binomial tables or calculated using the binomial equation.
In
each of these experiments, we have enumerated all possible numbers of
heads that we could obtain, from zero up to three, to ten, or to
50.
No
other com-
binations of heads and tails can occur. Therefore, the sum of
all
the probabilities
within each experiment must total 1.00, because we are absolutely certain to ob-
tain a result from among those enumerated. We can conveniently represent this by
setting the areas underneath histograms in
Figures
2-8
and
2-9
equal to
1.00,
as
was done
in
the histogram of
Figure
2-2.
The greater number of coin tosses
can
be accommodated only by making the histogram bars ever more narrow, and the
histogram becomes increasingly like a smooth and continuous curve. We can imag-
ine
an
ultimate experiment involving flips of
an
infinite number of coins, yielding
a histogram having
an
infinite number of bars of infinitesimal width. Then, the
histogram would be a continuous curve, and the horizontal
axis
would represent a
continuous, rather than discrete, variable.
In the coin-tossing experiment, we are dealing with discrete outcomes-that is,
specific combinations of heads and tails. In most experimental work, however, the
possible outcomes are not discrete. Rather, there is
an
infinite continuum of pos-
sible results that might be obtained. The range of possible outcomes may be finite
and in fact quite limited, but within the range the exact result that may appear
can-
not be predicted. Such events are called
continuous
random variables.
Suppose,
for example, we measure the length of the hinge line on a brachiopod and find it to
be 6
mm
long. However,
if
we perform our measurement using a binocular micro-
scope, we may obtain a length of 6.2
mm,
by using an optical comparator we may
measure 6.23
mm,
and with a scanning electron microscope, 6.231
mm.
A
contin-
uous variate can, in theory, be infinitely refined, which implies that we can always
find a difference between two measurements,
if
we conduct the measurements at
a fine enough scale. The corollary of this statement is that every outcome on a
continuous scale of measurement is unique, and that the probability of obtaining
a specific, exact result must be zero!
If
this is true, it would seem impossible to define probability on the basis of rel-
ative frequencies of occurrence. However, even though it is impossible to observe
a number of outcomes that are, for example, exactly 6.000..
.
000
mm,
it is entirely
feasible to obtain a set of measurements that
fall
within an interval around this
value. Even though the individual measurements are not precisely identical, they
are sufficiently close that we can regard them as belonging to the same class. In ef-
fect, we divide the continuous scale into discrete segments, and
can
then count the
number of events that occur within each interval. The narrower the class bound-
aries, the fewer the number of occurrences within the classes, and the lower the
estimates of the probabilities of occurrence.
When
dealing with discrete events, we
are
counting-a process that usually
can
be done with absolute precision. Continuous variables, however, must be measured
25