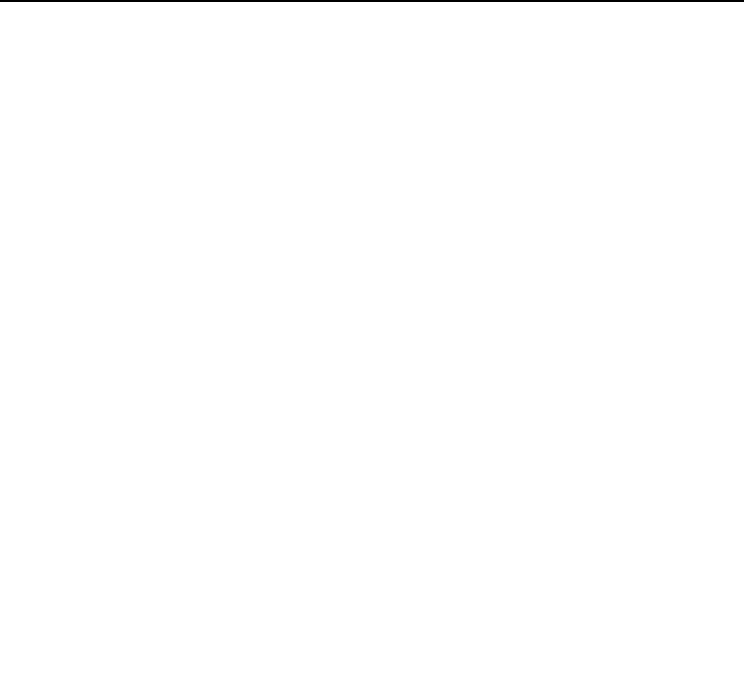
23Amaro Forests - Chap 20 25/7/03 11:08 am Page 227
227 Logistic Regression in Modelling Mortality
related to
π
(x). The model’s statistical evaluation is done on the basis of several cri-
teria developed to guide in choosing the best model from different candidates.
Assessing the fit is usually done with the Pearson
χ
2
statistic and the deviance. The
Hosmer–Lemeshow test looks for the correctness of the model in grouping probabil-
ities in risk deciles. The classification table describes the model’s goodness of fit
with the correct classification rate. The receiver operating characteristic (ROC) curve
(Hosmer and Lemeshow, 2001) is based on sensitivity and specificity measures. A
high ROC curve describes the good prediction ability of the model. The area under
the curve is interpreted as the model’s R
2
.
Multilevel logistic regression with random effect
Multilevel logistic regression models for hierarchically structured data have become
mor
e common in recent studies of social sciences and medicine (e.g. Hox, 1995;
Goldstein and Rasbash, 1996; Carlin et al., 1999; Fielding, 2000). Forestry applica-
tions are few (Jalkanen, 2001), and published tree mortality models, for example,
have been constructed from multilevel data, but in parameter estimation the data
structure has been ignored.
Multilevel binary data can be analysed using a logistic regression model which
is composed of fixed covariates fr
om different hierarchy levels and random effects
(Hosmer and Lemeshow, 2001; McCulloch and Searle, 2001). The random effects not
related to the lowest level of hierarchy are generally assumed to follow normal dis-
tribution with mean zero and constant variance. Here the random stand effect
describes the unmeasured variance between the stands in case of mortality. The
error variance (tree-level variance) is assumed to be:
2
(2)
xl
π
x
φπ
()
(
−
()
)
where
φ
is the dispersion scale (or extra-binomial variance). If
φ
= 1, then the error
variance is purely binomial. If
φ
> 1, the situation is called over-dispersion. Unlike
under-dispersion (
φ
< 1), over-dispersion is fairly common, and may occur when
data are collected from experiments with a hierarchical design (McCullagh and
Nelder, 1989). If the dispersion scale is forced to be equal to 1, the potential over-
dispersed variance is added to the random effect variance.
The ML and restricted maximum likelihood (REML) methods are relevant
when analysing linear multilevel models with normal distribution, but logistic
r
egression models with random effects need special quasi-likelihood estimation
methods. The key point is to estimate the effect of the covariates on the binary out-
come with awareness of the random effect(s). The idea of quasi-likelihood methods
is that only the model for the mean (response and covariates) and the relationship
between the mean and the variance is necessary for the estimation. Two quasi-likeli-
hood methods have been developed for estimation: the marginal quasi-likelihood
(MQL) and the penalized quasi-likelihood (PQL) methods. Today these methods are
available in most statistical software packages. However, Rodriguez and Goldman
(1995) have pointed out that in certain circumstances the first-order MQL estimates
are badly biased, sometimes more biased than those obtained by ML without ran-
dom effects. The PQL method has also been noticed to have a major problem in
practice if the cluster size is small (McCulloch and Searle, 2001). Alternatives to the
ML approach are, for instance, GEE, generalized estimating equations (Zeger et al.,
1988) or Bayesian methods (MCMC, bootstrapping) (e.g. Goldstein, 1995; Browne
and Draper, 2002), which will not be addressed in this chapter.