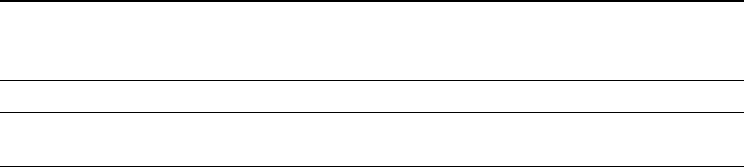
22Amaro Forests - Chap 19 1/8/03 11:53 am Page 222
222 A. Amaro
Table 19.6. Residual mean sum of squares for both SOP
1
and SOP
2
(n = 8577), considering both N2SLS
and N3SLS methods.
SOP model Number of parameters N2SLS N3SLS
SOP
1
(Amaro, 1997) 12 1.42223 1.45898
SOP
2
20 1.34977 1.34964
A new data set was obtained, filtered and validated and an estimation proce-
dure has been defined.
Due to the empirical nature of the
SOP system of equations, the step-by-step
approach, using different methods in order to estimate parameters, is probably more
appropriate. Although it is much more time-consuming, it allows, as the analyses
evolve, the addition of more knowledge to the system’s behaviour and understand-
ing.
The NOLS individual estimation allowed the initial estimate assessment and a
r
ough idea of its magnitude. The use of less heavy algorithms (Quasi-Newton and
Rosenbrock’s), although possibly not as efficient as Levenberg–Marquardt’s,
speeded up the individual estimation process.
The several possibilities for stand aggregation were used as an argument for the
simultaneous estimation with NOLS, using the r
egional dummy variables, built on
the basis of the less productive region (Poor). This procedure allowed the aggrega-
tion of regions without any concern for the verification of statistical assumptions:
seven different regions were selected as modelling units.
Due to the simultaneous nature of the equations, N2SLS was run and, further,
due to the corr
elation between errors on both equations and in order to prevent bias
and subsequently run significance tests, N3SLS was also run.
The estimates obtained during the three main steps on the seven final regions do
not dif
fer in a relevant way, although the final estimates obtained through N3SLS are
most probably the best estimates, and in particular produce the most efficient stan-
dard deviations parameter estimates that permit the significance tests.
The residual error variance estimate for
SOP
1
, actually being used by Soporcel,
was reduced when compared with
SOP
2
.
The statistical violations, namely the non-deterministic characteristic of both the
dominant height and basal ar
ea variables on the RHS of the correspondent equa-
tions, were considered non-relevant based on past analysis, parameter meaningful-
ness and impact of violation.
The non-significance of the parameter tests would lead to a region merge or
separation task that by no means invalidates the actual values: the pr
ocess is unfin-
ished and more work needs to be done in order to not only validate the
SOP
2
model
(which Soporcel will eventually do and will identify the real negative points of the
model) but also to continuously reduce the observed uncertainty (e.g. by modifying
the system’s functional forms – the most accurate way of targeting the statistical
assumptions – or by creating additional submodels). All of this must be assisted by
an accurate inventory database.
Finally and re-stating what has already been said (Amaro, 1997), water avail-
ability and fr
ost risk are both limiting factors to eucalyptus growth patterns! Water
seems to help to establish the potential and frost risk more as a rate-to-potential
regulator. In basal areas, however, the potential seems to be more influenced by frost
risk.