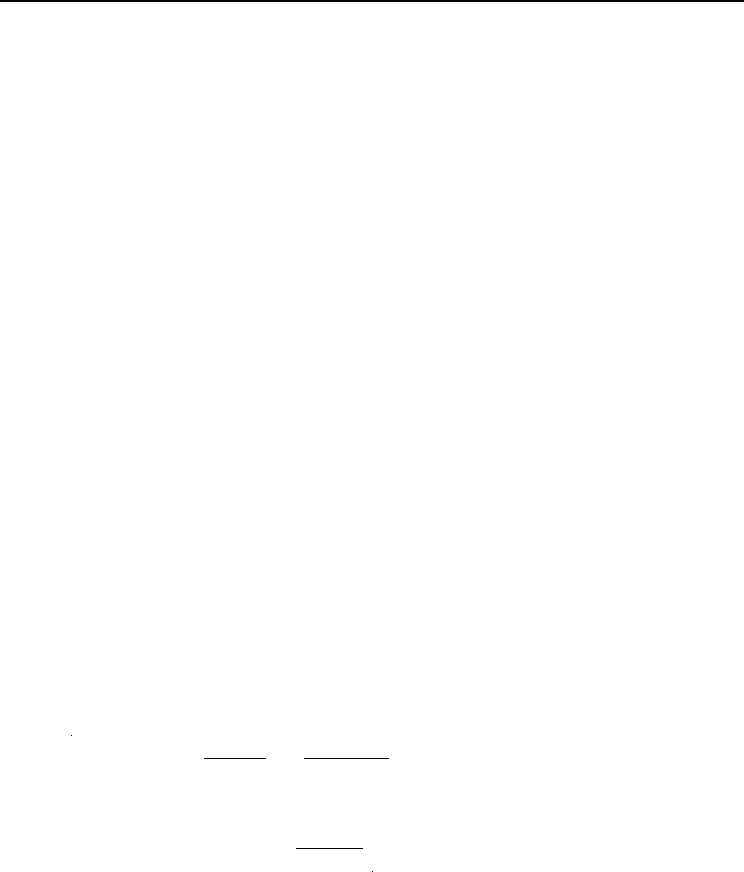
11Amaro Forests - Chap 09 1/8/03 11:52 am Page 103
103 GLOBTREE: an Individual Tree Growth Model
The modifier function (mod) was selected from a set of combinations of the type
= d
t1
+ipot × mod, where the modifier function was based on the logistic or exponen-d
t2
tial functions. The best modified functions were selected on the basis of the residual
mean of squares (RMS), non-existence of collinearity between variables, significance of
the parameters, normality of the studentized residuals and absence of heteroscedasticity
associated with the error term of the equations. Dominant trees were eliminated from
the data set, as they were assumed to attain the potential growth.
During the evaluation stage, bias and precision of the selected functions were
analysed based on the pr
ediction residuals (true value predicted value). Bias was
assessed from the mean of the prediction residuals. Precision was expressed by the
interquantile range of the prediction residuals (Q99–Q1) and by computation of the
mean of the absolute value of the prediction residuals. The model efficiency (ME)
was computed; this statistic provides a simple index of performance on a relative
scale, where 1 indicates a perfect fit, 0 reveals that the model is no better than a
simple average, and negative values indicate a very poor model (Vanclay and
Skovsgaard, 1997).
To develop the tree diameter increment equation, the total data set was ran-
domly split into two subsets and both wer
e used in order to fit, select and validate
the equations. To ensure that the data splitting was not affected by systematic influ-
ences, the equations selected in one data subset were evaluated using the other
subset, and vice versa.
Tree survival probability model
The probability of tree survival (P
) is simulated with a logistic function fitted with
the maximum likelihood method using
PROC LOGISTIC (SAS, version 6.12). This func-
tion is one of the most widely employed to express the probability of tree survival
(Vanclay, 1991; Zhang et al., 1997; Monserud and Sterba, 1999). The logistic function
is limited to the interval [0, 1] and the probability of death is given by (1P). The
dependent variable is a binary variable assuming 0 for dead trees and 1 for live
trees. The submodel to be fitted (logit transformation) is of the type:
( (
n
pS = 1) pS = 1)
f( ) x =
∑
b x
i
= ln
(
= ln
, where S is a binary variable, x is the vector
i=1
i
pS = 0) 1–(pS = 0)
of the independent variables, b is the vector of the parameters associated with the vari-
ables, and p(S=1) is the probability of survival in a defined period, being expressed
xb
f (, )
( (
xb
by
pS = 1) = (1− pS = 0)) =
f (, )
.
1 + e
The final model is the result of the stepwise logistic selection, which allows one
to define, based on the likelihood ratio χ
2
test, the most significant independent vari-
ables for the expression of the dependent variable. The tested tree and stand vari-
ables were selected based on the knowledge of the eucalypt system and the
behaviour modelled in the tree diameter growth equation. The practical non-exis-
tence of density-dependent mortality in the E. globulus stands, suggesting the sub-
occupancy of the site (Soares and Tomé, 1996), resulted in a reduced number of
observations in the subset ‘dead trees’. Consequently, distance-dependent competi-
tion indices, which imply a reduction in the number of useful trees, were not consid-
ered as independent variables. The qualitative analysis of the model was based on
standard tests and statistics for logistic regression (see, for example, Hosmer and
Lemeshow, 1989): likelihood ratio test – to analyse the overall significance of the
model; Wald’s test – to analyse the significance of one specific variable when the
other variables of the model are present; odds ratio – calculated for each independent