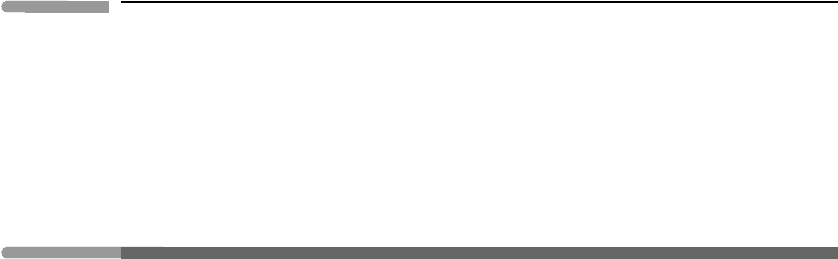
219 Uses of complex numerical models
still be difficulties in solving them numerically. For example, there may be a large number
of chemicals involved and hence a large number of equations in the set. Less obviously,
the possibility of both fast and slow reactions implies a disparity of natural time scales in
the differential equations, which are then said to be ‘stiff’ and require careful numerical
treatment.
9.3 Uses of complex numerical models
Over the past few decades the need for reliable weather forecasts has provided a strong
impetus for the development of complex atmospheric models, at least for the troposphere
and lower stratosphere. Numerical weather prediction models, for predicting a few days to
perhaps two weeks ahead, must include representations of large-scale dynamical processes,
small-scale frictional processes in the atmospheric boundary layer, small-scale drag due
to gravity waves, short-wave and long-wave radiative transfer, water-vapour transport, the
effects of clouds, precipitation and the transfer of heat, momentum and moisture between
the surface and the atmosphere. Some of these (such as cloud microphysical processes
and drag due to small-scale gravity waves) take place on length scales below the grid
size and are therefore not explicitly represented in the models. Such processes must be
parameterised, that is, expressed in terms of quantities that are explicitly represented.
Such parameterisations often involve empirical formulae that do not have rigorous physical
justification and may involve disposable constants whose values are poorly known. Much
of the art of NWP consists in selecting these constants in such a way as to optimise the
resulting forecasts.
Forecasts are made by integrating the equations of motion forwards in time, starting from
an observed initial state. Owing to measurement error and the inevitable sparsity of data in
some regions, the initial state may not be known with great accuracy. This can be a major
source of error in forecasts. In common with other chaotic processes, the evolution of the
atmosphere can be sensitive to initial conditions. One way to estimate the reliability of the
forecast model on any given occasion is to perform many forecasts, starting from slightly
different initial conditions. I f all members of the ‘ensemble’ of forecasts are similar,
then a high degree of reliability can be placed on a representative forecast; however,
if the ensemble contains widely differing forecasts, less weight can be placed on any
of them.
Complex models are also used for climate forecasting, for years or decades into the
future. Here the focus is on the long-term behaviour of the atmosphere, perhaps averaged
over seasons, rather than on the detailed day-to-day evolution. On these longer time scales,
some physical processes that can be neglected in NWP must be included: for example, a
representation of oceanic heat transfer is needed. Much work is being done, for example,
to estimate the global and regional responses of the climate system to various possible
scenarios for the increase of greenhouse gases over the coming decades. Climate-prediction
models of this kind involve very heavy usage of computer resources, not only because they
must be run for many simulated years but also because of the variety of physical processes